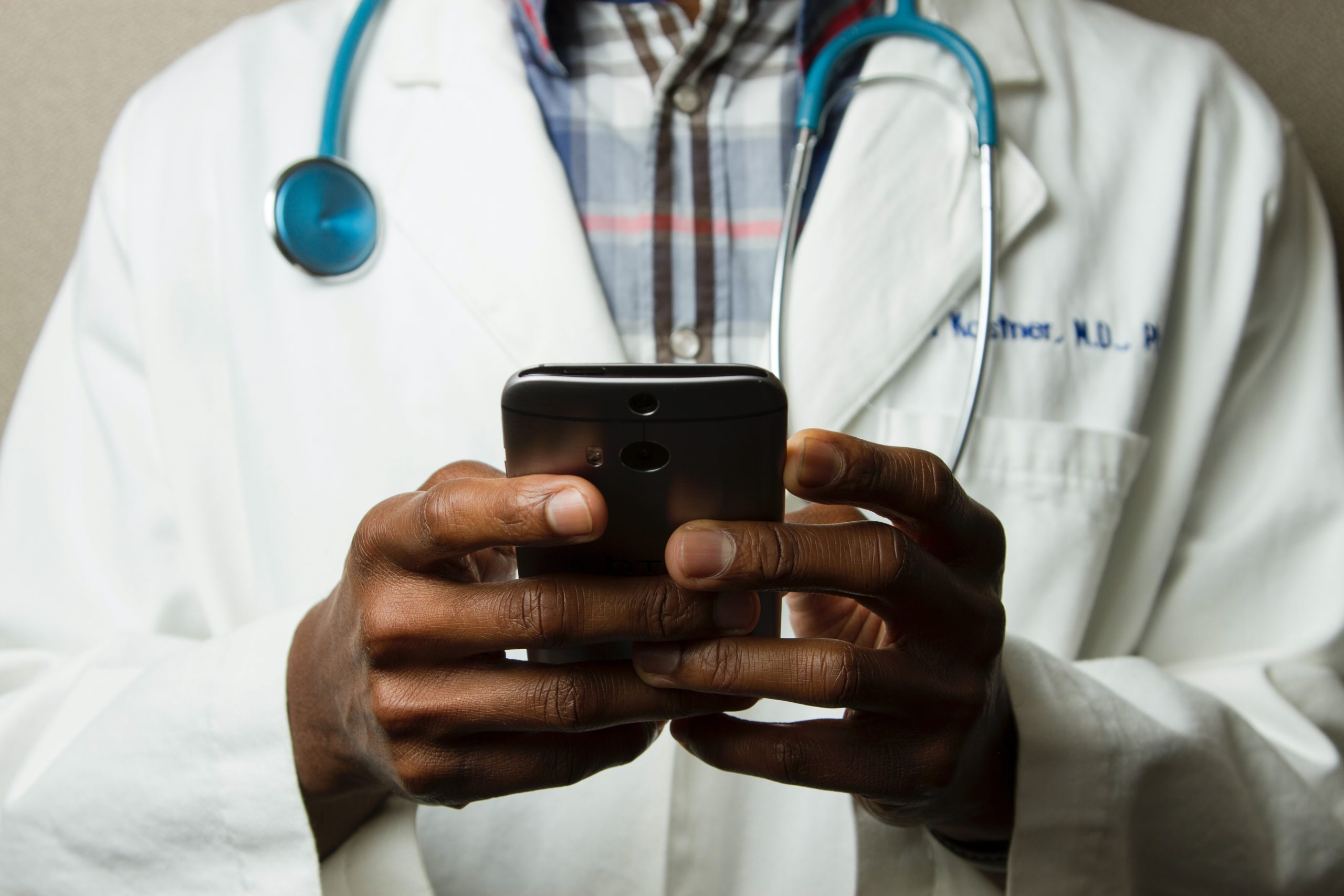
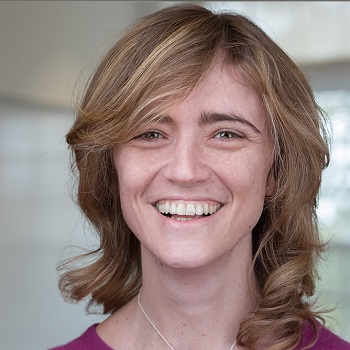
š¬ Research summary by Dr. Marianna Ganapini (@MariannaBergama), our Faculty Director.
[Original paper by Juan Manuel DurƔn & Karin Rolanda Jongsma]
Overview: The use of AI in medicine promises to advance this field and help practitioners make faster and more accurate diagnosis and reach more effective decisions about patientās care. Unfortunately, this technology has also come with a specific set of ethical and epistemological challenges. This paper aims at shedding some light on these issues and providing solutions to tackle the problems connected to using AI in clinical practice.Ā We ultimately concur with the authors of the paper that medical AI cannot and should not replace physicians. We also add that a trustworthy AI will probably lead to more trust among humans and increase our reliance on experts. Thus, we propose that we start looking at the question: under what conditions is an AI system conducive to more human-to-human trust?
Introduction
The use of AI in medicine promises to advance this field and help practitioners make faster and more accurate diagnosis and reach more effective decisions about patientās care. Unfortunately, this technology has also come with a specific set of ethical and epistemological challenges. The epistemological challenges are specifically connected to the opacity of the so-called āblack-box algorithmsā: āblack boxes are algorithms that humans cannot survey, that is, they are epistemically opaque systems that no human or group of humans can closely examine in order to determine its inner statesā. The problem is that these algorithms make assessments in a way that is opaque to both their designers and the physicians using them because it seems impossible to know how the algorithms came to their conclusions.
The challenges that this epistemic opaqueness poses are both epistemic (are these algorithms in fact reliable?) and ethical (are these algorithms ethical, e.g. fair, respectful of human autonomy?). Both of these challenges touch on the issue of warranted trust in AI: if I canāt check whether an algorithm is trustworthy (reliable & ethical), is trusting it ever permissible?
Even though this is not something the authors point out, it is worth noticing that ātrustā is already a loaded term: so letās unpack it a little bit. Say, an agent A can be said to trust B on some issue Y if A is willing to do at least one of the following: (i) A comes to believe what B says about Y and (ii) A uses what B says about Y as a sufficient reason for reaching a specific decision (e.g. making a certain diagnosis). Though we donāt employ the same terminology,Ā I believe the authors of the paper would agree that (i) and (ii) are not the same thing: (i) is what we can call ādoxastic trustā and (ii) is āpragmatic trustāĀ (note: the normative standards for doxastic trust might not be the same as for pragmatic trust).Ā
We are now in a position to reformulate the question of the paper: When is it permissible for a physician to pragmatically trust a black box algorithm? The authorsā answer is: even if the algorithm is reliable, what it says should rarely be used as a sufficient reason to make a diagnosis, prescribe a cure and so on. The algorithms’ recommendations need to be interpreted by the physician’s knowledge and understanding of the context and situation of the patient.Ā
Key Insights
To answer the question above the authors of the paper look at the relationship between transparency and opacity in black box algorithms.
Transparency ārefers to algorithmic procedures that make the inner workings of a black box algorithm interpretable to humans. To this end, an interpretable predictor is set out in the form of an exogenous algorithm capable of making visible the variables and relations acting within the black box algorithm and which are responsible for its outcome.āĀ
Opacity āfocuses on the inherent impossibility of humans to survey an algorithm, both understood as a script as well as a computer process.ā
Relation between transparency and opacity:
ādesigning and programming interpretable predictors that offer some form of insight into the inner workings of black box algorithms does not entail that the problems posed by opacity have been answered. To be more precise, transparency is a methodology that does not offer sufficient reasons to believe that we can reliably trust black box algorithms. At best, transparency contributes to building trust in the algorithms and their outcomes, but it would be a mistake to consider it as a solution to overcome opacity altogether.ā
The authors are arguing here that transparency is not the solution to the problems of an opaque AI: it might be part of the solution, but it is not enough. What is the missing piece? Ensuring our blackbox AI is reliable.
Solution: as part of the solution the authors adopt computational reliabilism (CR). As the authors put it, āCR states that researchers are justified in believing the results of AI systems because there is a reliable process (ie, the algorithm) that yields, most of the time, [correct/accurate] results.ā They provide some insights on how reliability-assessments should be made in the context of blackbox algorithms by discussing some reliability-indicators (e.g. verification, expert knowledge, transparency). These reliability-indicatorsĀ are still quite unclear, though.Ā
However, the key point is that doxastically trusting AI might not be enough to justify acting on it, as we mentioned earlier. This is a contextual matter: what constituted enough reason for acting may vary given the context and what is at stake. This could mean two things: one has to do with the fact that epistemic standards for pragmatic trust may be more stringent than for doxastic trust. The second has to do with the fact that reliability is just one among the factors that make AI trustworthy: we need to make sure AI is also ethical (e.g. fair) before acting on its assessments and predictions. The authors explain that āif recommendations provided by the medical AI system are [doxastically] trusted because the algorithm itself is reliable, these should not be followed blindly without further assessment. Instead, we must keep humans in the loop of decision making by algorithms.ā
In other words, even if considered reliable, an algorithm should be rarely used as the only reason for reaching a decision in clinical practice. āIt follows that it is unlikely and undesirable for algorithms to replace physicians altogether.ā
Between The Lines
The authors of this paper rightly argue that given what is at stake, (pragmatic) trust in medical blackbox algorithms is rarely justified. Practitioners and doctors still provide the necessary experience, reliability and commitment for patients to trust their decisions and diagnosis. That is, patients should trust doctors not algorithms. Doctors may trust algorithms to form beliefs but should not base their decisions only on what those algorithms say.Ā Ā Ā
As a result, I believe we need to focus our attention on how AI can be trust-conducive: experts that rely on a robust, ethical and helpful AI are also themselves more trustworthy. Doctors that rely on a trustworthy AI system will themselves be and be perceived as more skillful, experienced and reliable. Hence, AI does not replace physicians: a trustworthy AI is conducive to more and better trust among humans and will probably make us rely on our experts even more.Ā So from now letās ask the following question: under what conditions is an AI system conducive to human-to-human trust?