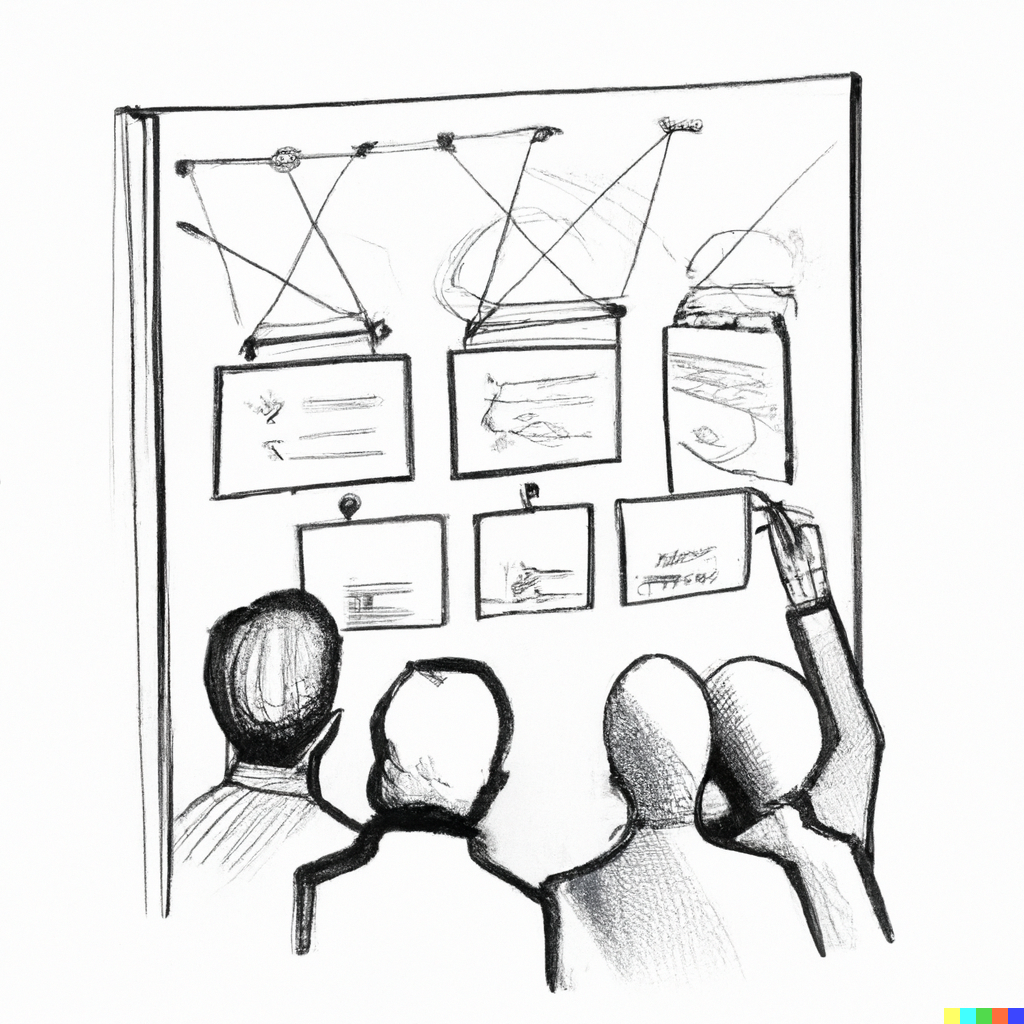
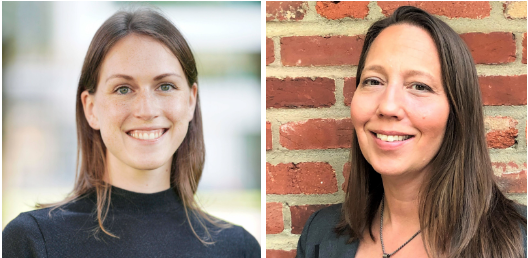
🔬 Research Summary by Mia Hoffmann and Heather Frase.
Dr. Heather Frase is a Senior Fellow at the Center for Security and Emerging Technology, where she leads the line of research on AI Assessment. Together with Mia Hoffman, who is a research fellow at CSET, she studies how to manage risks and harms from AI systems.
[Original paper by Mia Hoffmann and Heather Frase]
Overview: As AI systems are deployed across all domains of daily life, harmful incidents involving the technology become more frequent. This paper presents a framework for defining, identifying, classifying, and understanding harms from AI systems to facilitate effective monitoring and risk mitigation. Understanding whom, how, and when AI systems cause harm is critical for AI’s responsible design and use.
Introduction
In recent years, AI systems have been linked to many harms, including car accidents, wrongful arrests, racist healthcare decisions, discriminatory hiring, and digital sexual violence. Such real-world incidents reveal major shortcomings in existing AI safety efforts and represent crucial sources of information on previously unforeseen and underestimated risks. We developed a conceptual framework for defining, tracking, and classifying harm incidents caused by AI to better understand AI harms and inform risk mitigation efforts.
This framework
- Decomposes AI harm and defines its core elements
- Provides a structure for characterizing harm
- Serves different monitoring needs through customization
The CSET AI Harm Framework serves as a blueprint for organizations interested in tracking and studying harms from AI. It offers a standardized approach that increases comparability between different monitoring efforts. To demonstrate the framework’s use, we apply it to classify and characterize around 70 incidents from the AI Incident Database, a public archive of instances in which AI systems have been implicated in real-world harm, which can be explored here.
Key Insights
Defining AI Harm
We identify four essential components of AI harm that form the basis of the CSET AI Harm Framework:
- The harm itself can manifest in various ways, depending on the specific situation. What constitutes harm and does not is inherently subjective and guided by social norms and legal environments that can vary substantially worldwide.
- The entity experiencing the harm: Entities are people, organizations, places, or things. Knowing who or what AI harms is key for accountability and learning about risks. It can also help developers of AI identify groups and communities that should be involved in stakeholder engagement processes.
- The AI system involved: AI harm cannot occur without an AI system. Examples of AI systems include chatbots powered by Large Language Models (LLMs), AI systems used to approve loans, AI-assisted driving in vehicles, and facial recognition used to unlock phones, among many others.
- The AI-to-harm link: This component establishes a clear relationship between the AI system and the harm. The harm must be specifically attributed to the AI system’s behavior, even if it is not the sole cause. For example, a phone with an AI assistant is not directly at fault if a child accidentally calls an expensive number. However, an AI HR tool recommending job candidates by name is to blame if it leads to discrimination.
Structuring Harm
Types of harm
Because what is considered harmful will likely differ across organizations engaging in AI incident tracking, our framework distinguishes two categories to enhance consistency.
The first category, ‘tangible harm,’ covers material and generally observable harms. This includes, for example, injuries, financial loss, or physical property damage. Tangible harms are more similarly defined and understood to be harmful across communities and organizations, and due to their material effect, their occurrence is less disputed.
Intangible harm, the second category, encompasses harm that is neither material nor observable in itself despite often having observable causes and consequences. Intangible harm includes invasion of privacy, differential treatment, psychological harm, or rights violations. Because privacy, fairness, and rights interpretations vary widely, intangible harms are less consistently identified as harm.
Actual and potential harm
In addition to characterizing different types of harm, the framework distinguishes between harm that happened (harm events) and harm that nearly happened or could reasonably have happened (harm issues). By monitoring and analyzing realized harm events, we can assess the effectiveness of existing harm mitigation efforts and learn about emerging types of harm not previously anticipated. Tracking potential harms allows us to take preventive actions before the harm actually occurs for the first time.
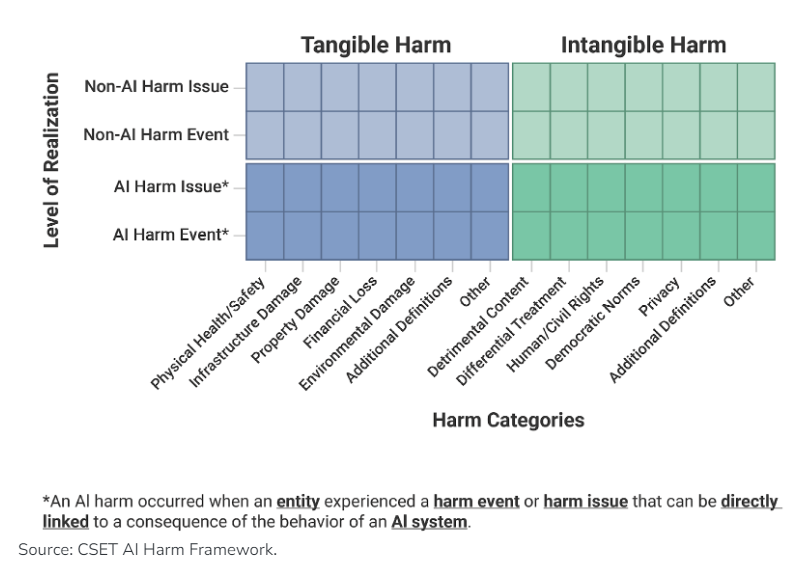
The Framework in Practice
To apply the framework to data, parties engaging in harm-tracking efforts should customize the CSET AI Harm Framework. This requires specifying the qualities and scope of each of the four elements presented above to establish a practical definition of AI harm. This involves deciding on the types of harm that will be monitored and developing a definition of ‘AI’ that serves their research purpose. Some organizations might be more interested in algorithmic harm, while others may want to focus only on machine learning technology. Yet others might just be interested in tracking harms from generative AI systems.
To illustrate, our paper explains how we customized the CSET AI Harm Framework to reflect our analytic goals and the specific data in the AI Incident Database (AIID). The below figure summarizes the customization, the CSET AI Harm Taxonomy for AIID. The annotation guidance for this taxonomy provides specific definitions, illustrative examples, guidance on complicated situations, and details on supplementary characteristics of interest that the CSET AI Harm Taxonomy for AIID records.
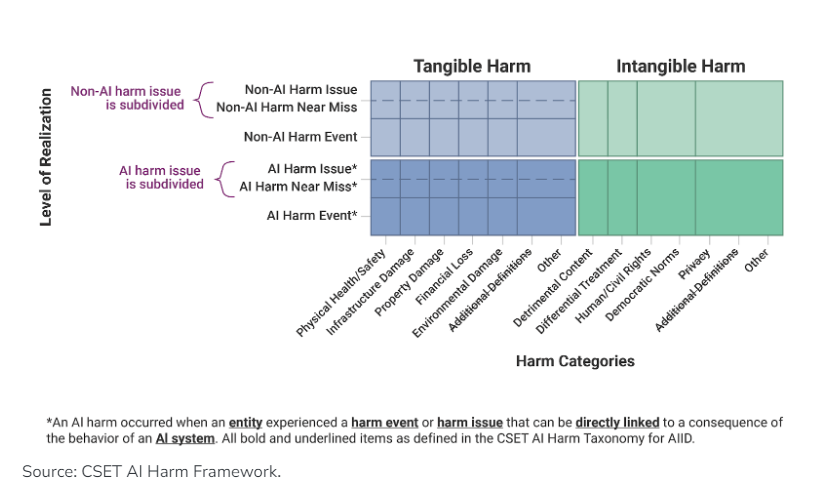
Between the lines
Despite the obvious need for better harm prevention methods for AI and the insights that can be gained from studying harm incidents, formal processes currently don’t exist to track AI harms at scale. This has so far been complicated by the scarcity of hard data and the difficulty of defining ‘AI harm.’ We tackle both issues with the CSET AI Harm Framework and the corresponding taxonomy. By adding structure to data on AI harms, we intend to turn the plethora of reports, articles, and anecdotes of incidents into evidence of diverse and significant harm from AI.
As we continue to add to the dataset, the resulting analyses can improve risk mitigation efforts, for example, by broadening the scope of vulnerabilities a system is tested for or informing the design of standards governing the challenges of human-AI interaction. Analyses also help identify where use-case specific deployment limitations and restrictive terms of use are required and where non-technical engagement and evaluation procedures, such as stakeholder involvement, serve best to mitigate risks.
Benefits are amplified when data on incidents is systematically collected, structured, and analyzed. That would facilitate the detection of patterns across AI use cases and methods and help identify priority areas for interventions. Tracking over time enables evaluations of new mitigation strategies and policy and regulatory efficacy. Critically, when data and findings are shared, vulnerabilities detected once can be mitigated by all. Our CSET AI Harm Framework can serve as a blueprint for others interested in studying harms from AI.