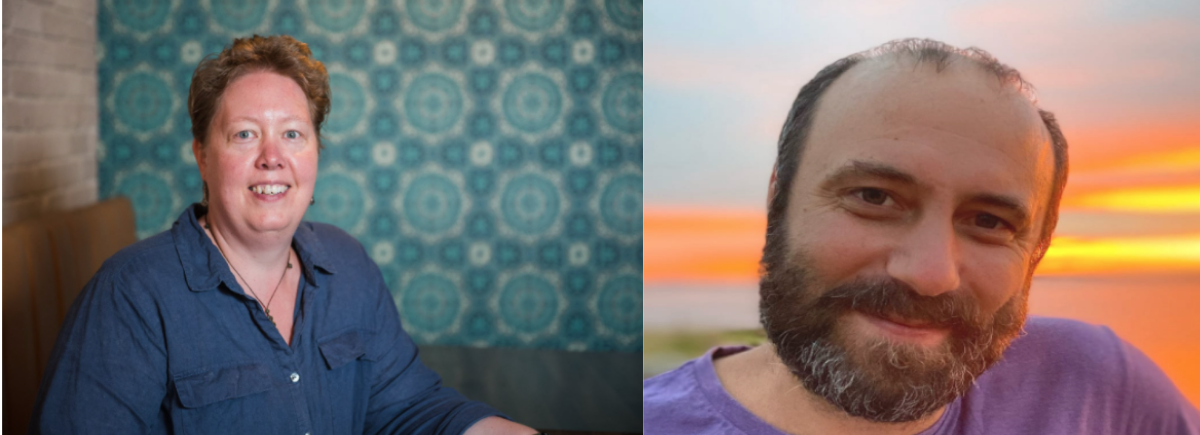
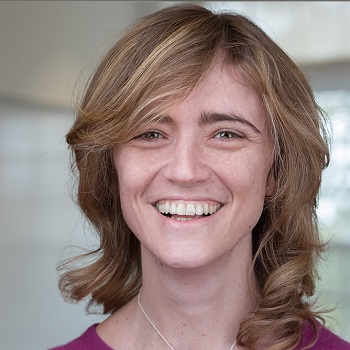
✍️ Column by Dr. Marianna Ganapini, our Faculty Director. This is part 4 of her Office Hours series. The interviews in this piece were edited for clarity and length.
What’s missing in the way Tech Ethics is taught currently? Two experts in this field, Heather von Stackelberg and Mathew Mytka, shared their ideas and experiences on these and other vital issues. See their full bios at the end of the article.
What is your background? What courses do (or did) you teach connected to Tech Ethics, and who’s your audience (e.g., undergrads, professionals)?
Heather: My Masters’s is a cross-disciplinary social sciences degree with a focus on Adult Education. I also have undergraduate degrees in communication studies and biological sciences. I usually teach with colleagues who have CS/engineering graduate degrees. I don’t, at the moment, teach a course that is solely on Tech Ethics, but I teach classes on AI literacy and AI management for business leaders, which have vital ethics components. I teach mainly to business professionals, though it’s not unusual to have a substantial minority of post-secondary students in any given class.
Mat: I’ve got a relatively diverse 27-year career—everything from construction and journalism to product management and UX design. I studied social sciences and spent several years working at the intersection of information sharing, trust, and ethics. I’ve designed and delivered entrepreneurship programs to co-design modules for postgrads and data ethics to professionals and practitioners. For the past three years, I’ve been leading programs with Greater Than X, helping cross-functional teams to operationalize data ethics. We have also worked on national data sharing ecosystems like Consumer Data Right in Australia and Open Banking in the UK, and universities like Northwestern Kellogg School of Management’s Trust Project. Late last year, we launched a social learning platform to help people design more trustworthy organizations and technology. One of the recently published courses on the platform is a primer to operationalizing data ethics. It builds on the work we’d been doing in our services business with global clients and years of working in these areas. The framework we cover in that course is more like an operating system to interface with modern product development workflows, factoring in proactive, reactive, and retroactive decision making and diverse stakeholder engagement.
What kind of content do you teach? What topics do you cover? What types of readings do you usually assign?
Heather: What I teach is very focused on application and real-world examples. It’s usually not so much the technical aspects of AI but how the technical relates to the business and larger social and ethical impacts. So we talk a lot about how to choose a project that provides business value and is technically feasible. On the ethics side, we talk about ethical questions across the entire lifecycle – from selecting an appropriate project (that is, one that isn’t inherently meant to harm people) to data collection, data labeling, choosing proxies and metrics, operationalizing concepts like fairness, to working with users, training them, evaluating, and watching for unexpected consequences.
Assigned readings aren’t relevant for industry training, but I have a list of resources I recommend if people ask. We also have been working to develop reference sheets, summaries, and checklists that are more likely to be used by people in the industry than textbooks or academic papers.
Mat: The community at Greater Than Learning. as a broad thematic area, we’ve focused on helping people design more trustworthy products and services. We cover topics such as operationalizing data ethics, behavior design in organizational change, and how the collection and use of people’s data influence trust. There are also areas on how to design privacy notices or terms of use and establish the workflows to do this in a modern business. Content is a small part of it. So you might learn about these topics via watching a video or exploring a scenario. Then reflective activities to connect it to your lived experience. There is a range of learning experiences, but most of the focus is on social learning amongst the small but diverse community of practice. While readings are not prescribed, we provide reference reading in courses, from journal articles to books. These might be articles from someone like Luciano Floridi to books like Social Physics from Alex Pentland. But because it’s a social learning platform, content comes via community members sharing resources with each other.
What are some teaching techniques you have employed that have worked particularly well? For Tech Ethics, what kind of approach to teaching do you recommend?
Heather: We’ve had people work through a full proposal for an ML project, which requires them to make decisions about it and state the reasons for their choices. That seems to work well for getting people to think about the realities and application of the ideas and principles. Of course, this only works in more extensive, long-term courses. In sessions that are only a few hours, this isn’t practical. The other method that seems to work with Ethics is the discussion of case studies. Provide an example of an organization with a notable ethics failure, and discuss why they did it and both what and how they should have done differently. Again, you need to have enough time for that discussion, which is difficult when you’re teaching busy industry professionals.
Mat: Any extensive curriculum needs to cover a comprehensive set of fields, from philosophy and history to science fiction and co-design. But most of all, it needs to be practical. It needs to simulate the pressures that come with making ethical decisions when designing and building technology. You have to do it to learn it! Assigning applied learning projects that involve interdisciplinary focus is one technique we’re exploring with the community at Greater Than Learning. When it comes to tech ethics, we need to focus on experiential knowledge and practical skills, helping with forming ethical “muscle memory.” If ethics is about deciding what we should do, learning environments need to mimic the decision-making context. There is little point in learning about consequentialism or deontological approaches if the learner can’t relate. And this approach has worked when I’ve been helping people in organizations where people are dealing with real trade-offs and commercial constraints, and they have a “skin in the game.” Indeed, a perception of loss, risk, and consequence are essential motivators in learning even, more so when it comes to learning to navigate the grey area of tech ethics.
In your opinion, what are some of the things missing in the way Tech Ethics is currently taught? For instance, are there topics that are not covered enough (or at all)? What could be done to improve this field?
Heather: The thing that often frustrates me is how often deep research and best practices from the social sciences are ignored in STEM fields, especially Data science and ML. For example, all of the research and best practices on getting accurate and unbiased data annotation (which in ML is data labeling) or on validating a proxy. These are very relevant in ethics and preventing harm, but when I talk about them to CS people, they’ve often never heard about it before.
Mat: I don’t have a clear view of the ways tech ethics is being taught everywhere. So what I express here is based on my limited reach into all the different formal and informal learning contexts. There is a wide range of topics being covered across this space. Universities and various organizations have courseware popping up. There’s loads of content and reports to read, history to draw upon, or frameworks and principles to learn. So it’s not for lack of material that there are gaps. What’s missing is the practical focus. How on Earth do I raise an ethical concern in the workplace when I don’t feel safe to do so? Or how might I work through a moral decision on a new product feature within a cross-functional team? What metrics matter, and how do we measure them? How might we balance our commercial KPIs with our responsibility to the communities in which we impact? How do we bring these communities into the design process upfront? The skills required for where the rubber meets the road are missing in most of what I’ve seen in the market. It’s hard to “teach” tech ethics without the realities of actually doing it.
In general, the field is emergent, and there are intersecting movements. Be that responsible innovation and humane tech, data for good and tech ethics or many other permutations. I think we’re all trying to work through the ambiguity, sense-making and find pathways to learn better. So from that perspective, there needs to be more coordinated collaboration across these diverse communities of practice. Be that to co-create curricula or to support ongoing and experiential learning. I believe we need an open-source social network for learning in this area that leads by example. It shows people how it’s done and involves people in the process by also providing a case study for the ecosystem to learn from. There is a plethora of providers and platforms talking big on tech ethics and using technologies that misalign to the values they espouse. For example, if I come to your website and you’re communicating all these things about ethical tech, it sets some expectations. If, at first, I get a cookie consent notice that gives me no real choice…well, that’s misalignment! This ‘ethical intent to action gap’ eventually diminishes trust.
How do you see the Tech Ethics Curriculum landscape evolve in the next five years? What are the changes you see happening?
Heather: Both in post-secondary and in the industry, the big thing that is missing – and is slowly changing – is the operationalization of AI ethics principles. Globally, we’re slowly converging on a generally agreed-upon set of principles. Still, we’re only at the beginning stages of defining what they mean in terms of the day-to-day work of data scientists and ML scientists. Over time, I think we’re going to see the development of norms, standards, and best practices for the development of ML that integrate and operationalize those ethical principles. Still, it’s going to take a while.
Mat: I think there will be a plethora of curriculums evolving both via formal and informal methods. I’ve seen an increase in the number of universities offering courses on these topics. Then certifications are also starting to pop up. Workplaces are increasingly advertising for these new roles. And curriculum design in this space will be more collaborative and shifting to a practical focus: I see the various communities of practice coalescing and co-creating curriculums. It’s already been happening, and there is some fantastic thinking and direction starting to take shape. And the demand for these types of courses is there.
The signal-to-noise ratio will be a problem, though in the sense that the commercial opportunity in the space brings with it organizations that are mainly in it for the money. Shiny courseware and big talk attract the audience. This means that upskilling in these areas is potentially being done in an inferior way. People might get certification as an ethical technologist, and they get a promotion or are hired based on this credential. Still, it is unclear that all certificates should have the same value.
It will be messy, and lots of misconceptions will develop. So we’ll see a mix: inadequate approaches to curriculum design and more innovative approaches to co-creating and crowdsourcing curriculums. There’s so much great work happening in this space, so I believe that what is happening is positive on balance.
Is there anything else you’d like to add?
Mat: Yes. If anyone reading this wants to collaborate on crowdsourcing curriculums, reach out. We can create better together.
Full bios of interviewees:
Heather von Stackelberg: Heather is a Machine Learning Educator at Alberta Machine Intelligence Institute (Amii). She has been teaching and developing educational material on the interactions between society and machine intelligence systems. Before this, she taught math, chemistry, and biology at MacEwan University in Edmonton and at two First Nations colleges.
Mathew Mytka: Mat is a humanist, generalist, father, gardener, wannabe extreme sports enthusiast, and Co-founder of Greater Than Learning. He’s worked across digital identity, e-learning systems, behavioral design, decentralized technologies, and spent the past few years helping organizations to design more trustworthy products and services.