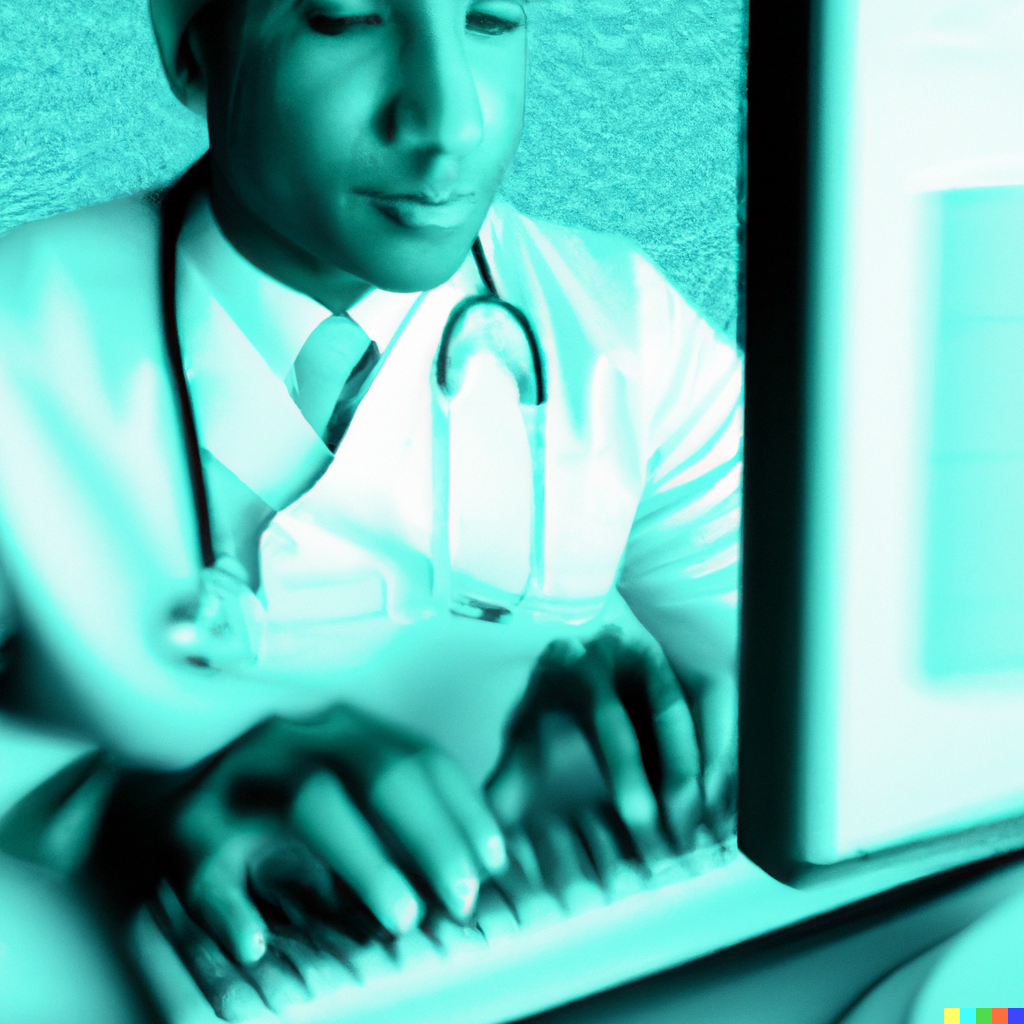
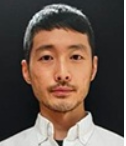
🔬 Research Summary by Min Lee, an Assistant Professor in Computer Science at Singapore Management University, where he creates and evaluates interactive, human-centered AI systems for societal problems (e.g. health).
[Original paper by Min Hun Lee and Chong Jun Chew]
Overview: Although advanced artificial intelligence (AI) and machine learning (ML) models are increasingly being explored to assist various decision-making tasks (e.g., health, bail decisions), users might place too much trust even with ‘wrong’ AI outputs. This paper explores the effect of counterfactual explanations on users’ trust and reliance on AI during a clinical decision-making task.
Introduction
Advanced artificial intelligence (AI) and machine learning (ML) models are increasingly being considered to increase efficiency and reduce the cost of performing decision-making tasks from various types of organizations and domains (e.g., health, bail decisions, child welfare services, etc.). However, users might place too much trust in the AI/ML system and even agree with ‘wrong’ AI outputs, and they achieve worse performance than humans or AI/ML models alone.
Key Insights
What did we do?
In this work, we contribute to an empirical study that analyzes the effect of AI explanations on users’ trust and reliance on AI during clinical decision-making. Specifically, we focus on the task of assessing post-stroke survivors’ quality of motion. We conducted a within-subject experiment with seven therapists and ten laypersons to compare the effect of counterfactual explanations with one of the widely used AI explanations, feature importance explanations.
- Feature importance: describes the contribution/importance of each input feature (e.g., kinematic variables — joint angle, distance between joints for the context of the study)
- Counterfactual explanations: describe how the inputs can be modified to achieve an AI output in a certain way (e.g., how does a patient’s incorrect/abnormal motion need to be changed to become a normal motion?)
One potential reason for overreliance on AI might be that humans rarely involve analytical thinking on AI outputs. This work hypothesizes that reviewing counterfactual explanations will allow a user to think critically about changing AI inputs to update an AI output and improve the user’s analytical review of an AI output to reduce overreliance on AI.
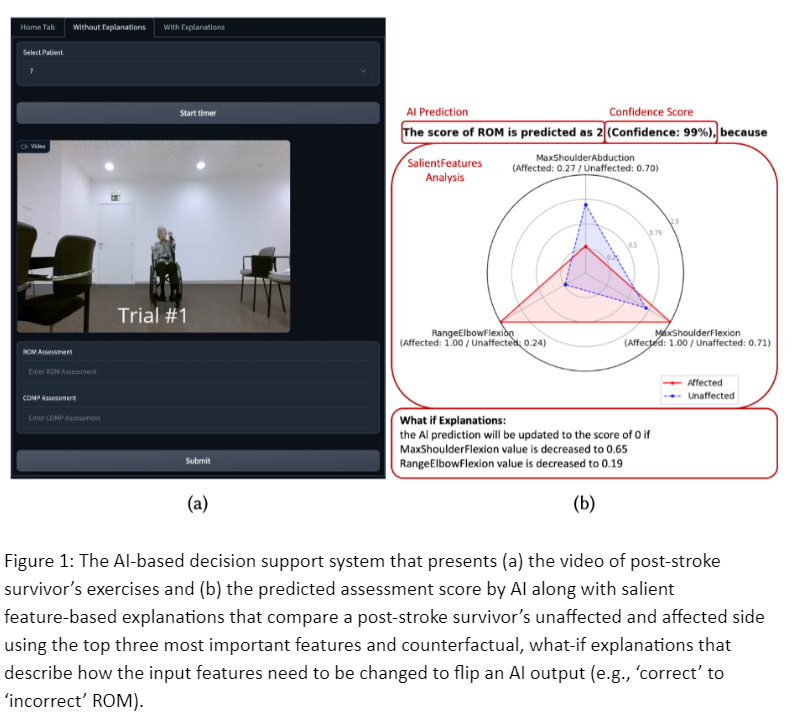
What did we learn?
- When ‘right’ AI outputs were presented, human+AI performance with both feature importance and counterfactual explanations increased than humans alone
- When ‘wrong’ AI outputs were presented, human+AI performance with both feature importance and counterfactual explanations decreased than humans alone
- Counterfactual explanations reduced overreliance on ‘wrong’ AI outputs by 21% compared to feature importance
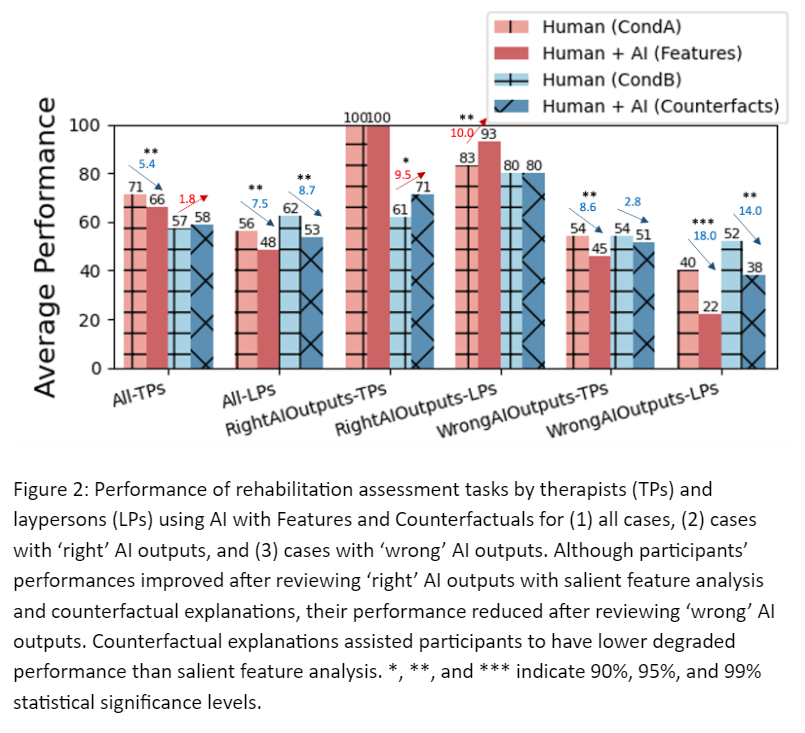
- Domain experts (i.e., therapists) had lower performance degradation and overreliance on ‘wrong’ AI outputs than laypersons while using both feature importance and counterfactual explanations
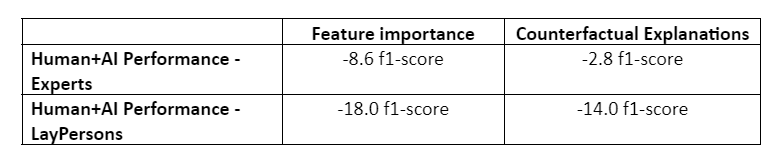
- Both experts and laypersons expressed higher subjective usability scores of ‘usefulness,’ ‘less effort & frustration,’ ‘trust,’ and ‘usage intent’ on feature importance than counterfactual explanations.
Between the lines
Implications: Our work shows that providing AI explanations does not necessarily indicate improved human-AI collaborative decision-making. This work provides new insights into:
1) the potential of counterfactual explanations to improve analytical reviews on AI outputs and reduce overreliance on ‘wrong’ AI outputs with the cost of cognitive burdens.
2) a gap between users’ perceived benefits and actual trustworthiness/usefulness of an AI system (e.g., improving performance while relying on ‘right’ outcomes)
Please check our paper for the details of this work (link). If you are interested in further discussing this work or collaborating in this space, please contact Min Lee (link).
Citation Format: Min Hun Lee and Chong Jun Chew. 2023. Understanding the Effect of Counterfactual Explanations on Trust and Reliance on AI for Human-AI Collaborative Clinical Decision Making. Proc. ACM Hum.-Comput. Interact. 7, CSCW2, Article 369 (October 2023), 22 pages. https://doi.org/10.1145/3610218