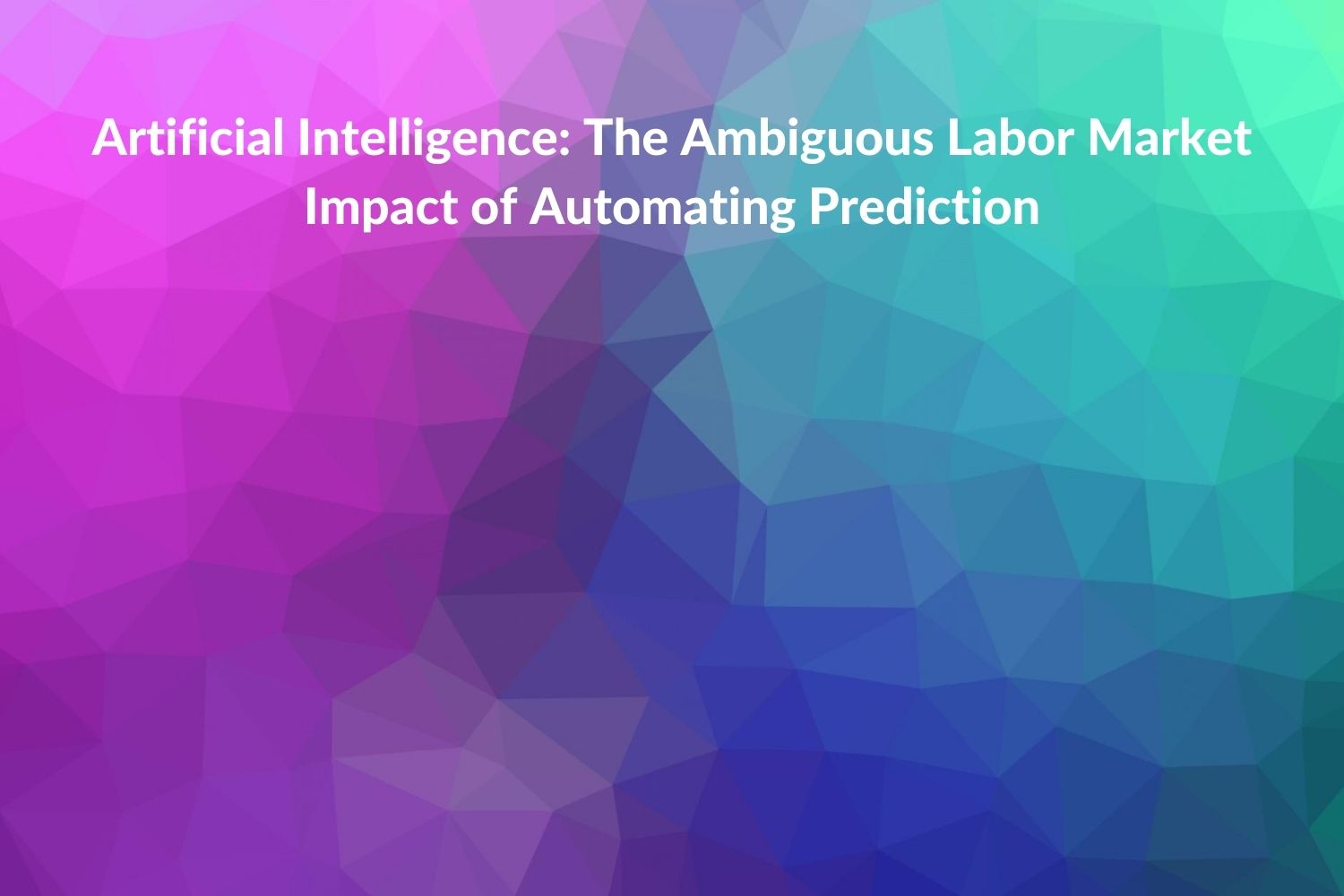
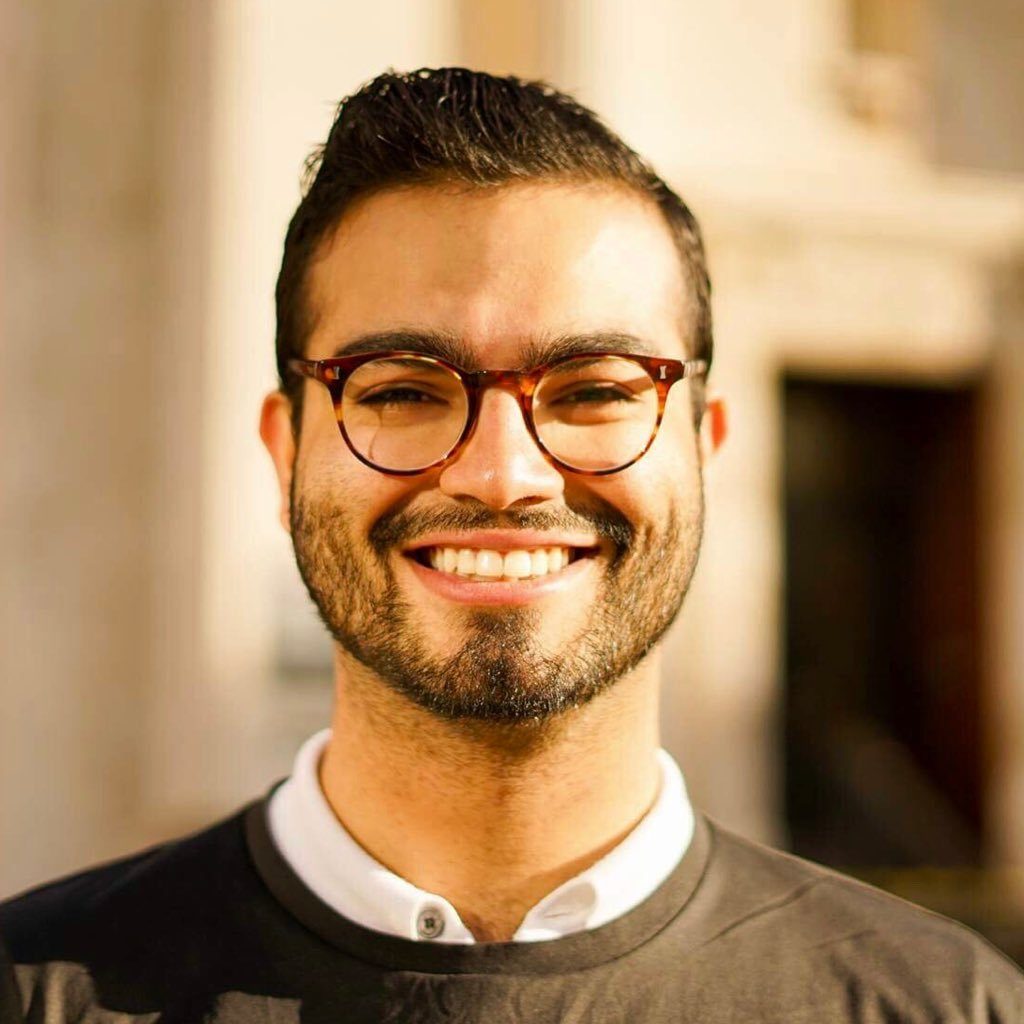
Summary contributed by Ryan Khurana, a graduate of UofT’s Rotman School of Management in the Master’s of Management Analytics program.
*Authors of original paper & link at the bottom
Mini-summary:
The automation impacts of artificial intelligence have been the subject of much discussion and debate, often hampered by a poor demarcation of the limits of AI. Agrawal, Gans, and Goldfarb have provided a framework that helps us understand where AI fits into organizations and what tasks are at risk of being automated. They argue that AI is fundamentally a prediction technology, and prediction is one of the key aspects of a decision-task, though not the only one. Things like judgement and action are also critical parts of decision-making and are not susceptible to direct automation by AIs. This does not mean, however, that they will not be affected by improved prediction, since the value of judgement and action may change as predictions become cheaper, better, and faster. Using this framework they identify four possible ways AI can affect jobs: replacing prediction tasks, replacing entire decision tasks, augmenting decision tasks, and creating new decision tasks.
A job is highly susceptible to automation when the work is mainly one of prediction. Tasks like responding to emails done by executive assistants, case summaries done by paralegals, and demand forecasting done by operations staff are all capable of being done by AIs either now or in the near future. These jobs, however, often have non-prediction tasks as well, and being able to redesign workflows to emphasize the uniquely human tasks is critical for enabling them to continue to provide value. Another possibility is judgement and action tasks being automated because predictions make them easier. They give the example of self-driving cars which can process the environment, anticipate the behavior of other actors, and predict the outcomes of different maneuvers with higher accuracy and speed than a human. Under these conditions the expert judgment required in good driving may become less valuable. Another possibility is that better predictions can enable humans to do their jobs better, such as in emergency rooms, where faster and higher quality assessments improve the ability for medical staff to prioritize patients and give them even better care. Finally, there may be new tasks that the rise of AI creates that were previously too costly or unnecessary. We already see this with the rise of data labelling. By providing this framework the authors help us have a more nuanced assessment of the impacts of automation, allowing policy makers, educators, and business leaders to understand what tasks should be emphasized and what initiatives can help workers prepare for AIs impact on their jobs.
Full summary:
The automation impacts of artificial intelligence have been the subject of much discussion and debate, often hampered by a poor demarcation of the limits of AI. In this paper, Agrawal, Gans, and Goldfarb build upon the framework that they introduced in their book Prediction Machines, that AI is fundamentally a prediction technology, and as it improves it will decrease the cost of prediction resulting in wider use of the technology in prediction tasks. The effect this has on labor then depends on the relative importance of prediction in a given job. They identify four possibilities.
The first is that many job tasks are pure prediction, for example forecasting work within operations departments, legal summary work done by paralegals, and email response work done by executive assistants, are all tasks that can be substituted by AIs as is, and possibly with greater efficiency, threatening these jobs if they do not have other high value-add tasks to do. A second possibility is that while a task may have a decision-component beyond prediction, this would no longer be important if predictions were better and cheaper. They give the example of autonomous vehicles, as driving is a common task that involves both prediction (what is happening in the environment around you and the potential payoffs of each decision) and judgement (what is the right action to take given this information). This judgement component may only be important because humans cannot make as fast and accurate a prediction as an autonomous vehicle. Should understanding the environment and the outcome of each action be nearly instantaneous and highly accurate, picking the one with the highest expected reward given a certain set of rules may result in the human judgement having reduced importance. In these contexts, even decision tasks could be substituted by AIs when the predictions are fast, cheap, and high quality enough.
The third possibility is that AI results in greater labor need as expert judgement becomes an important complement to better prediction. For example, in emergency medicine should diagnostics become better, faster, and cheaper through better AI, medical staff are now able to have a more accurate understanding of their patient needs, being able to prioritize workloads better and make targeted interventions. This increased productivity could in turn make hospitals more efficient, requiring more staff to provide even more care. Finally, the possibility exists that new types of tasks are created by the advent of AI. We can already see this appear somewhat in the data labelling industry that has arisen to support the models being deployed. As prediction becomes better and cheaper there may be more tasks that were simply unfeasible when prediction was poor and costly that are now able to be done.
This paper provides a valuable framework for decomposing the impacts of AIs on jobs and provides a language for understanding the different effects it can have on workers depending on their specific circumstances. This theoretical understanding can provide the basis for more nuanced quantifications of the employment impact of new AIs, help policy makers and educators understand the skills that will have stable demand, and help businesses prepare for worker transition by identifying which employees require skills support.
Original paper by Ajay Agrawal, Joshua S. Gans, and Avi Goldfarb: https://papers.ssrn.com/sol3/papers.cfm?abstract_id=3341456