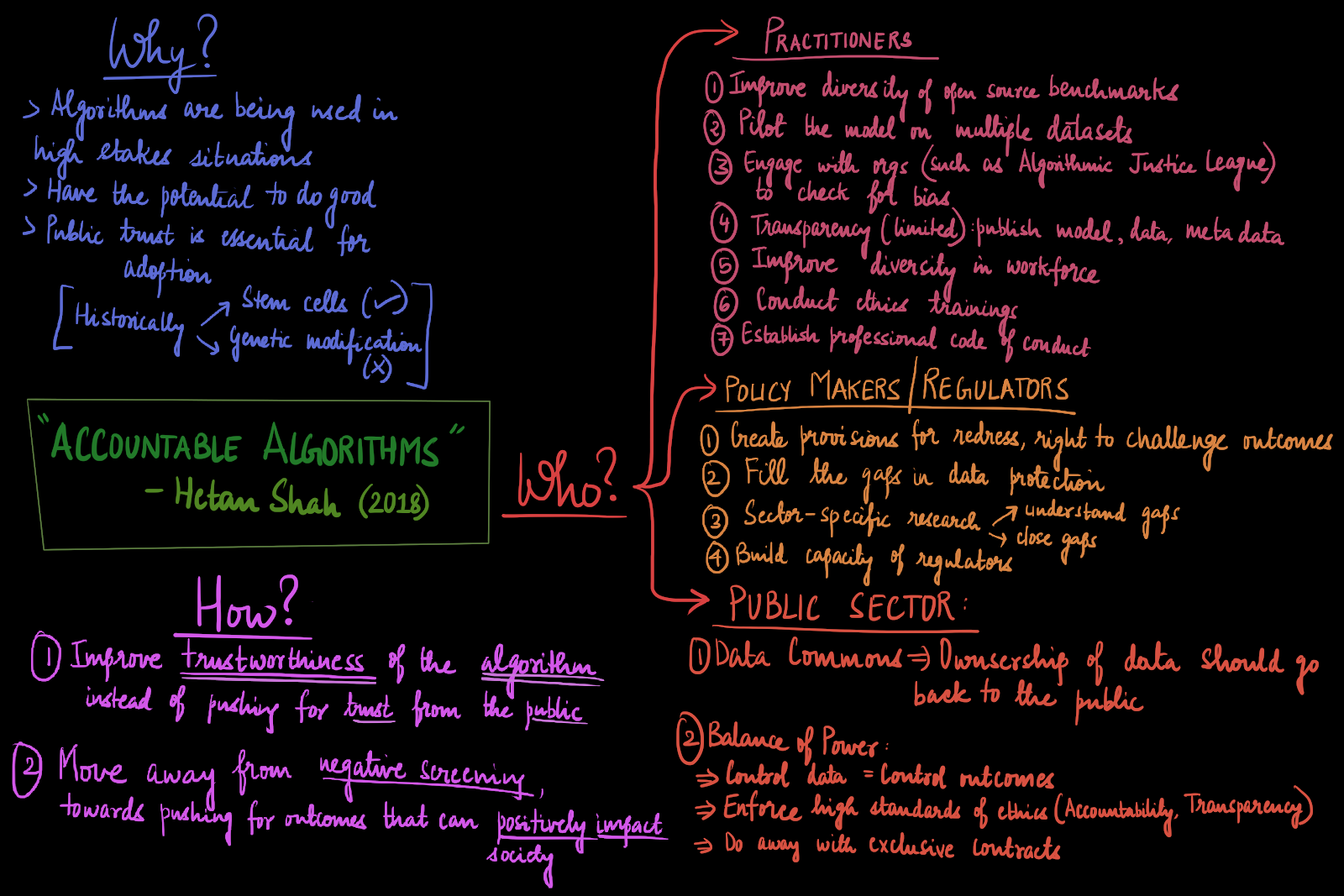
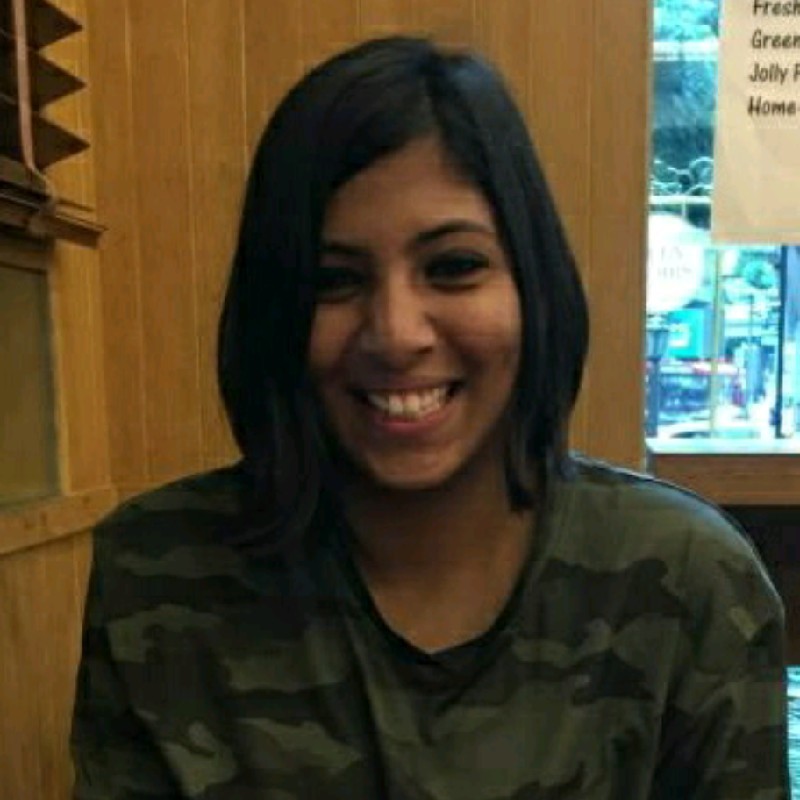
Summary contributed by Falaah Arif Khan, our Artist in Residence. She creates art exploring tech, including comics related to AI.
Link to full paper + authors listed at the bottom.
Mini-summary: Considering algorithms are being used in high stakes situations and that public adoption is essential for adoption, it is essential that we figure out a way to make algorithms accountable. We can do this by improving trustworthiness of algorithms, moving away from negative screening toward pushing for algorithms that can impact society positively. This has varying implications for 3 primary groups of stakeholders: practitioners, regulators, and the public sector.
Full summary:
The public trust in algorithmic decision-making systems is at an all-time low. In the last 6 months we’ve seen three major companies abandon their pursuit of general-purpose Facial Recognition software and as of this week, a government scrapping the results of an Exam Grading algorithm. Given the immense economic interest in and the rapid adoption of this (arguably) budding technology, AI fiascos have become commonplace in recent times, but what is truly groundbreaking this time around is the power of the public in forcing the hands of powerful companies and governments to disavow this technology in settings where it’s performance has been sub-par.
With this social backdrop, it serves us to remember some of the guiding principles for creating Accountable Algorithms laid out by Hetan Shah in his op-ed from 2018. In this piece, Shah argues that it is crucial to build public trust in a new piece of technology before pushing for its widespread adoption. He contrasts the speedy adoption of Stem Cell technology, that had a great deal of careful public dialogue around it during its development, with the paralyzing impact of public pushback on the advancement of genetic modification technologies. He then underscores that the best way to build trust is to implicitly improve the trustworthiness of algorithms, rather than explicitly pushing for more public trust.
Given the gravitas of the situation, there needs to be a coordinated effort by all the different stakeholders; namely: the practitioners (research labs, industry), the public sector and the policy-makers/regulators.
Practitioners need to be more conscientious about the creation of open source benchmarks by improving the diversity and representation in datasets. This is critical since benchmarks navigate the research direction of entire communities and so bias needs to be eradicated at its root. Shah also recommends piloting any model before it is deployed, using multiple datasets. In settings where in-house expertise is lacking, companies could engage bodies such as the Algorithmic Justice League to audit biases in models. Another approach could be to monitor for differential impacts, specially on vulnerable demographics, using causal models and counterfactuals. While Shah agrees that transparency would help only in a limited capacity, he recommends publishing models and the associated data and meta-data.
In addition to technical solutions, Shah outlines some important process changes that companies can make, such as improving diversity in the workforce, conducting ethics training and enforcing a professional code of conduct.
Regulation also has a key role in building trust. Following suit with the EU’s GDPR, provisions such as the right to challenge an unfair decision from an algorithmic system and the right to redress, would go a long way in confirming a commitment to mitigating the negative effects of misbehaving models. Other recommendations from Shah include building capacity for regulators to be able to understand and close gaps across the several sectors in which algorithms are driving decisions.
Lastly, the public sector holds a key role in building trust. Shah envisions the emergence of a Data Commons, in which the ultimate ownership of data would go back to the public. This would allow for a much-needed balance of power, where the public could do away with exclusive contracts and have the bargaining power to enforce high standards of accountability and transparency from any contractor that wishes to use their data to create predictive models.
Keeping pace with evolving technology is an uphill battle, but it is one we must take on. As Shah eloquently argues in this piece, we need to approach the creation of accountable algorithms by pushing for systems that can positively impact society, and do away with our extant approach of negative screening/mitigating damage.
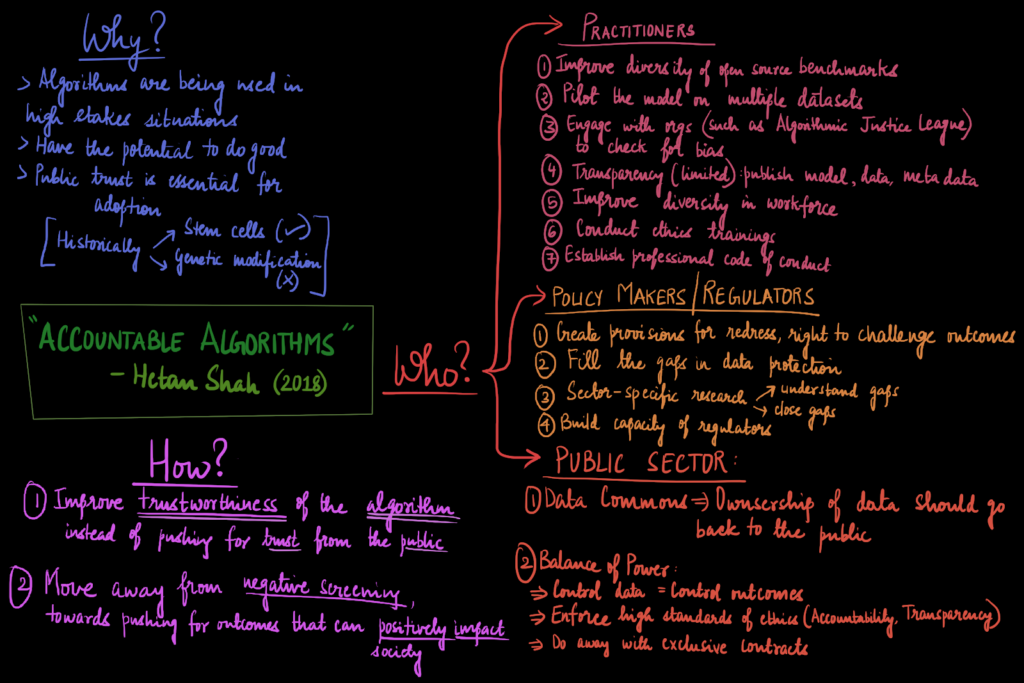
Original paper by Hetan Shah: https://royalsocietypublishing.org/doi/pdf/10.1098/rsta.2017.0362