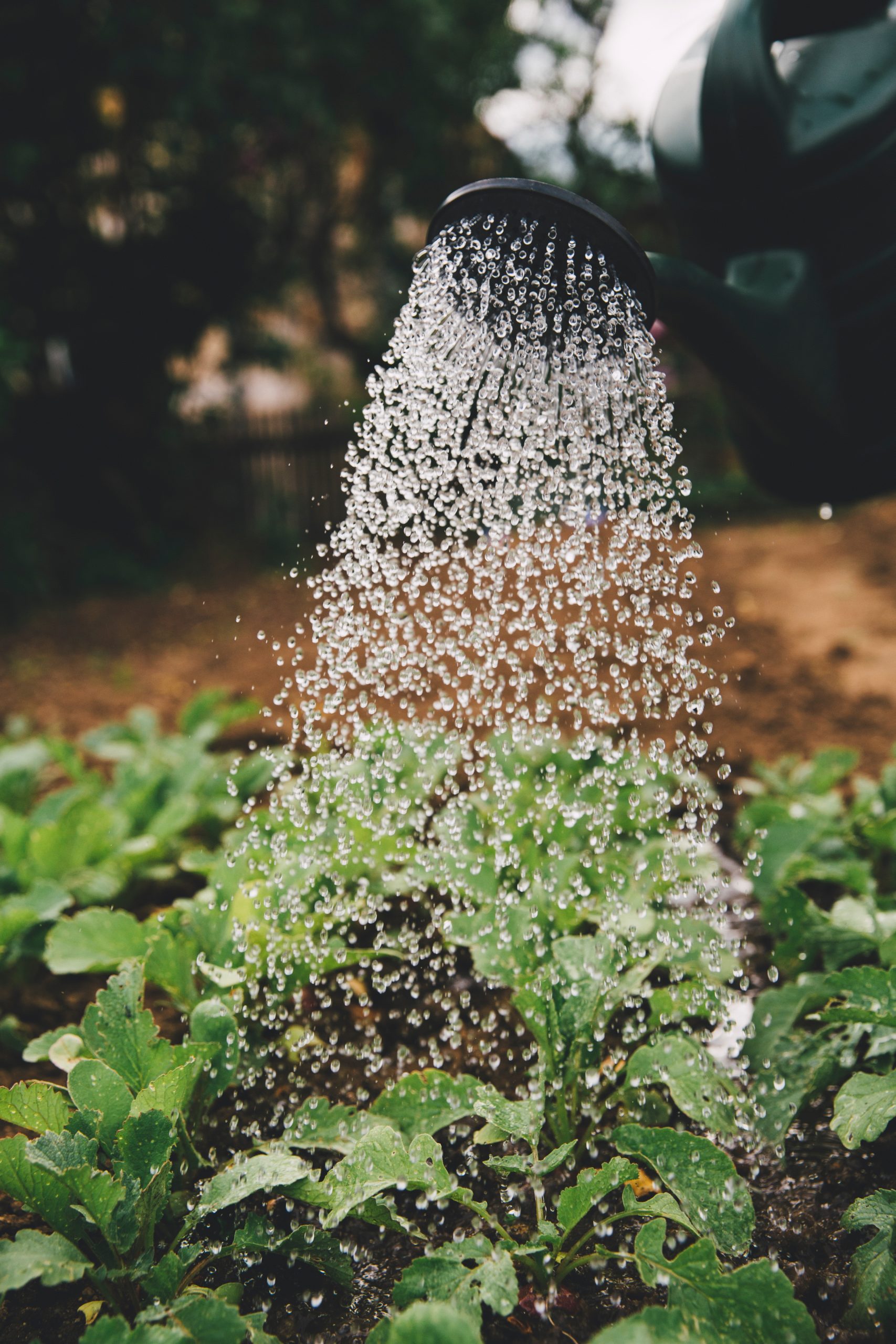
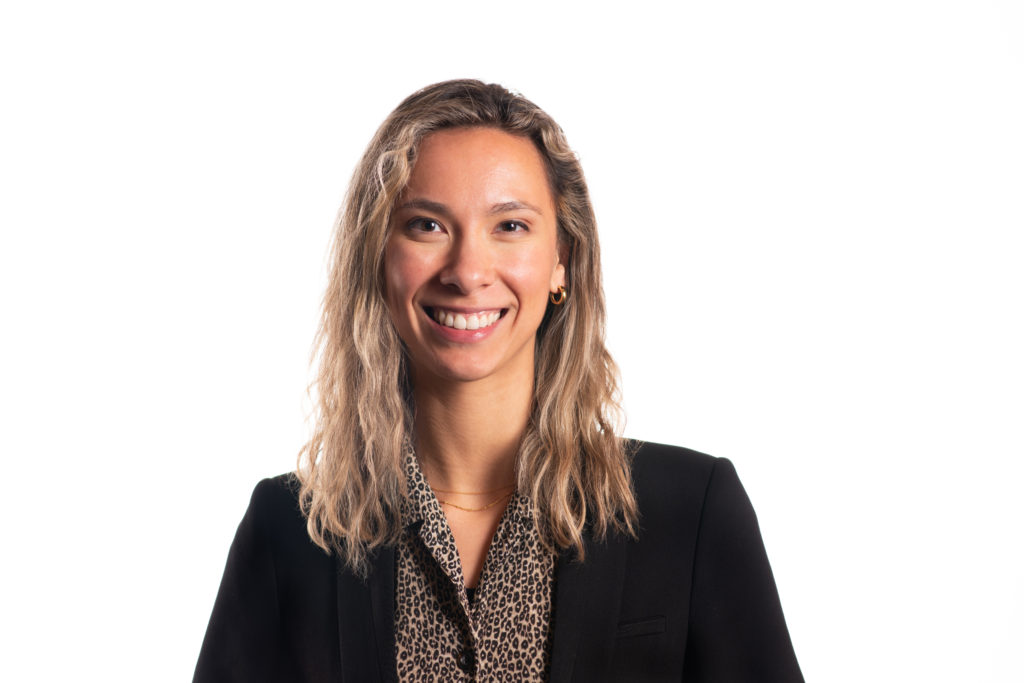
🔬 Column by Julia Anderson, a writer and conversational UX designer exploring how technology can make us better humans.
Part of the ongoing Like Talking to a Person series
Product launches are complex. Conversational AI, like many AI products, relies on large datasets and diverse teams to bring them to the finish line. However, these product launches are often iterative, requiring future optimization to satisfy customers’ needs.
Sustainable AI is the quest to build AI systems that are as compatible for a company as it is for the people they are serving. This type of sustainability means rethinking the lifecycle of AI products so that the technology can scale while minimizing costs to consumers, society and the environment.
Without the proper mechanisms in place, today’s big product launch can become yesterday’s embarrassing mistake. Beyond maintenance costs, concerns around integration, secure data gathering and ethical design must be addressed. Creating AI that is compatible with scarce resources requires proper AI governance, collaborative research and strategic deployment.
Responsibility as a Cornerstone
Adopting artificial intelligence is appealing to businesses that want technology to guide decision-making, automate processes and save time. While the promise of AI is immense, widespread adoption is much slower in practice. To ensure seamless and scalable integration, various stakeholders must have a seat at the table to determine AI’s vision and governance.
The principles of Responsible AI may serve as a starting point for businesses that are serious about developing an AI strategy. While governance varies within organizations, it helps when the CEO or senior leader accepts responsibility of AI leadership. As responsibilities are assigned, decisions can be held accountable. The challenge then becomes how to measure success. Oftentimes, data scientists and engineers must be involved early on to clear up misunderstandings about how AI can grow alongside a company to solve its problems.
Typically, a formidable culture shift is necessary to implement a new vision. If AI isn’t trusted or understood within the organization, then its customers likely will hold similar perceptions. This is the basis of a social license. In AI a social license is the perception that the technology is using fair and transparent algorithms to benefit the markets they are operating in. For conversational AI such as in-car assistants, this could mean incorporating the objectives of not only automobile drivers, but manufacturers and regulators, as well.
Considering the perspectives of various stakeholders also means constant iteration. Rather than see AI software as “plug-and-play technology with immediate returns” companies should embrace cross-functional teams, user feedback and the reality that the first deployment won’t be the last. Before technology can scale within a business, the company must understand customer complexities as well as what data are required to create a successful experience.
Gathering the Goods
A common, and costly, pitfall is not gathering enough data. For chatbots or voice assistants, this is a limiting factor in not only how the technology understands customer problems, but how they can solve them as well. Choosing the right learning model for the problem along with using a representative training data set can reduce biases early on and yield better long-term results. Without the right model, disasters like Microsoft’s Tay, a bot that adopted the personality of a racist Internet troll, spring up.
In conversational AI, there are usually several pieces of a platform that create the end experience. For enterprise solutions, this incorporates NLP performance, backend data integration, security, data storage and more. The question of building vs. buying a platform is a crucial one, due to the high costs of switching systems later on. When an organization uses one service for speech recognition and another for dialog management, for example, another question arises: “How well can we secure our data?”
Obtaining high-quality datasets requires time and money. One way to make this easier is by using human-in-the-loop solutions. Here, humans can create and label datasets that are reviewed by the AI system and refined by humans. Biases are inevitable but could be reduced if the humans in the loop are working under responsible AI leadership.
Data privacy remains a contentious subject for users who may not understand how a company’s system shares information to deliver customer experiences. Sometimes, companies don’t understand how different elements of the system work together. While many vendors claim to have an “enterprise-ready platform” to create conversational AI solutions, this is often not the case. The secure transfer of data is one of many challenges that vendors must overcome. Once a solution is deployed, companies may feel pressured to stay with a vendor due to the complexities of switching to an alternative.
Environmental Expectations
The misallocation of computing power is a cost to the public. For one, the money necessary to train large data models is a barrier to entry for smaller firms, stifling competition and research projects. With less equitable access comes fewer opportunities to tackle community challenges.
Customer perceptions of AI products are always a factor for businesses trying to scale. Depending on the use case, people may want to solve a problem by talking to humans over conversational AI. When this finding isn’t clear to the designers or developers, then a company may have invested in the wrong solution. The inability for organizations to connect AI investments to business value hinders innovation.
Due to AI’s evolving nature, it is difficult to manage expectations of what success looks like. However, carbon efficiency is one metric being explored. Green Algorithms is one tool striving to quantify carbon footprints at a time when there are no universal standards to measure carbon impact. The Informations & Communications Technology industry, where Machine Learning and AI are fast-growing disciplines, generates about 2% of global CO₂ emissions and counting.
New solutions such as LightOn are finding ways to use less energy for complex computations using novel hardware. Crystal, a conversational AI platform, auto-classifies business requests with half the computing power of similar products. Thoughtful design paired with such innovation can bring sustainability to the forefront of AI solutions.
Conclusion
A narrow AI vision can set the stage for larger operational and ethical issues down the line. By realizing there is no one-size-fits-all approach to emerging technology, sustainable AI can be a nimble solution in a once rigid landscape.
Businesses like growth. When speed is prioritized over collaboration, however, AI deployments may not live up to expectations.