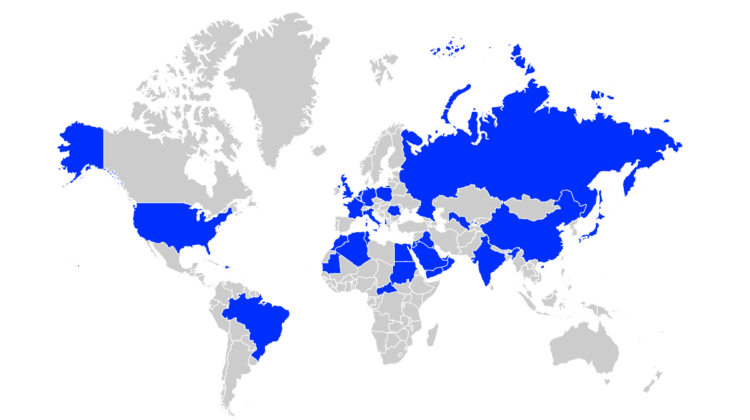
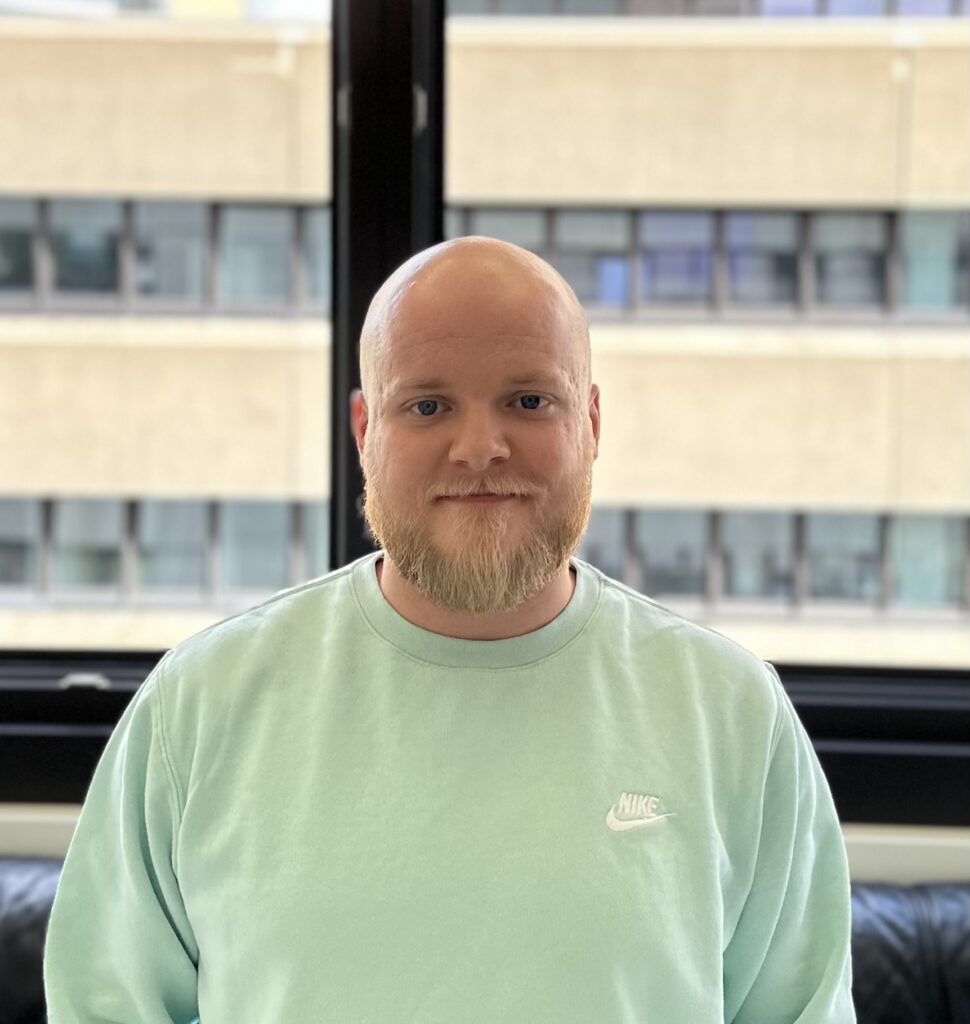
🔬 Research Summary by ✍️ Timm Dill
Timm is a Student Research Assistant at the Chair for Data Science, University of Hamburg.
[Original Paper by Margaret Mitchell, Giuseppe Attanasio, Ioana Baldini, Miruna Clinciu, Jordan Clive, Pieter Delobelle, Manan Dey, Sil Hamilton, Timm Dill, Jad Doughman, Ritam Dutt, Avijit Ghosh, Jessica Zosa Forde, Carolin Holtermann, Lucie-Aimée Kaffee, Tanmay Laud, Anne Lauscher, Roberto L Lopez-Davila, Maraim Masoud, Nikita Nangia, Anaelia Ovalle, Giada Pistilli, Dragomir Radev, Beatrice Savoldi, Vipul Raheja, Jeremy Qin, Esther Ploeger, Arjun Subramonian, Kaustubh Dhole, Kaiser Sun, Amirbek Djanibekov, Jonibek Mansurov, Kayo Yin, Emilio Villa Cueva, Sagnik Mukherjee, Jerry Huang, Xudong Shen, Jay Gala, Hamdan Al-Ali, Tair Djanibekov, Nurdaulet Mukhituly, Shangrui Nie, Shanya Sharma, Karolina Stanczak, Eliza Szczechla, Tiago Timponi Torrent, Deepak Tunuguntla, Marcelo Viridiano, Oskar van der Wal, Adina Yakefu, Aurélie Névéol, Mike Zhang, Sydney Zink, Zeerak Talat]
Proceedings of the 2025 Conference of the Nations of the Americas Chapter of the Association for Computational Linguistics: Human Language Technologies (Volume 1: Long Papers), pages 11995–12041, April 29 – May 4, 2025.
Image header: Figure 1: Regions with recognized stereotypes in SHADES.
Overview
SHADES represents the first comprehensive multilingual dataset explicitly aimed at evaluating stereotype propagation within large language models (LLMs) across diverse linguistic and cultural contexts. Developed collaboratively by an international consortium of researchers, SHADES compiles over 300 culturally-specific stereotypes, rigorously gathered and validated by native and fluent speakers across 16 languages and 37 geographical regions.
The primary objective of SHADES is to facilitate systematic analyses of generative language models, identifying how these models propagate stereotypes differently depending on their linguistic and cultural inputs. Historically, bias evaluations in AI have predominantly focused on English-language and Western-centric stereotypes, neglecting the complexities and subtleties present in different cultural contexts. They have also tended to be fairly limited linguistically, created using simple sentence structures that ease the automatic generation of sentences based on templates. SHADES addresses this critical shortfall by categorizing stereotypes based on linguistic form, the identities they target, and their cultural relevance. Comprehensive methodological details and the dataset itself are openly accessible, serving as essential resources for researchers, technologists, and policymakers seeking to benchmark and address the nuanced challenges of multilingual stereotype propagation.
Why It Matters
The SHADES dataset substantially advances our understanding of biases within multilingual AI systems, directly impacting discussions around fairness, accountability, transparency, and safety within the broader context of AI ethics. As AI tools continue to be deployed globally, it is imperative to move beyond Western-centric perspectives and thoroughly investigate how stereotypes permeate multilingual AI environments.
The implications of this research extend far beyond academia. Policymakers can leverage insights from SHADES to craft more inclusive regulatory frameworks. Developers and technologists can use the dataset to inform more effective bias mitigation strategies, ultimately leading to fairer and safer AI systems. Furthermore, global communities gain critical transparency into the ways in which AI might unintentionally perpetuate harmful stereotypes. SHADES thus provides a foundational resource for ongoing global initiatives dedicated to mitigating bias within AI, which increasingly shapes public discourse, media representation, education, and societal interactions.
Between the Lines
While the creation of SHADES marks significant progress, the dataset and associated research reveal important ethical and methodological complexities that require thoughtful consideration:
- Cultural Context and Ecological Validity: SHADES underscores significant inconsistencies in stereotype sensitivity across various stereotype categories and linguistic contexts. Notably, stereotypes related to nationality or regional identities are less regulated within existing AI frameworks compared to stereotypes around categories like gender. This inconsistency emphasizes the urgent need for culturally sensitive AI governance approaches that consider local nuances and the context-specific implications of stereotype propagation.
- Confirmation and Automation Bias Risks: The dataset highlights the potential for stereotype propagation to be amplified through confirmation bias (accepting information aligned with pre-existing beliefs) and automation bias (excessively trusting automated systems). These cognitive biases pose a risk of normalizing and embedding harmful stereotypes within society, thereby shaping public attitudes and possibly exacerbating existing societal prejudices and divisions.
- Equity in Data Representation and Resource Distribution: Multilingual datasets, such as SHADES, inherently risk reinforcing existing global inequities. Languages and cultures that are already underrepresented in data and resources may inadvertently be further marginalized. Addressing this issue demands a deliberate effort toward equitable representation, genuine collaboration with diverse communities, and avoidance of “data colonization,” thus ensuring the responsible and fair development of multilingual AI resources.
Moving forward
SHADES is one step in addressing technology’s tendency to reify views that disproportionately disadvantage marginalized communities. It highlights many further opportunities to improve technology for everyone, including culturally sensitive data collection and new methods for analysis. Ultimately, by bringing together people from diverse cultures and languages to participate in defining what AI technology should be, we can create technology that better serves the needs of users throughout the world.