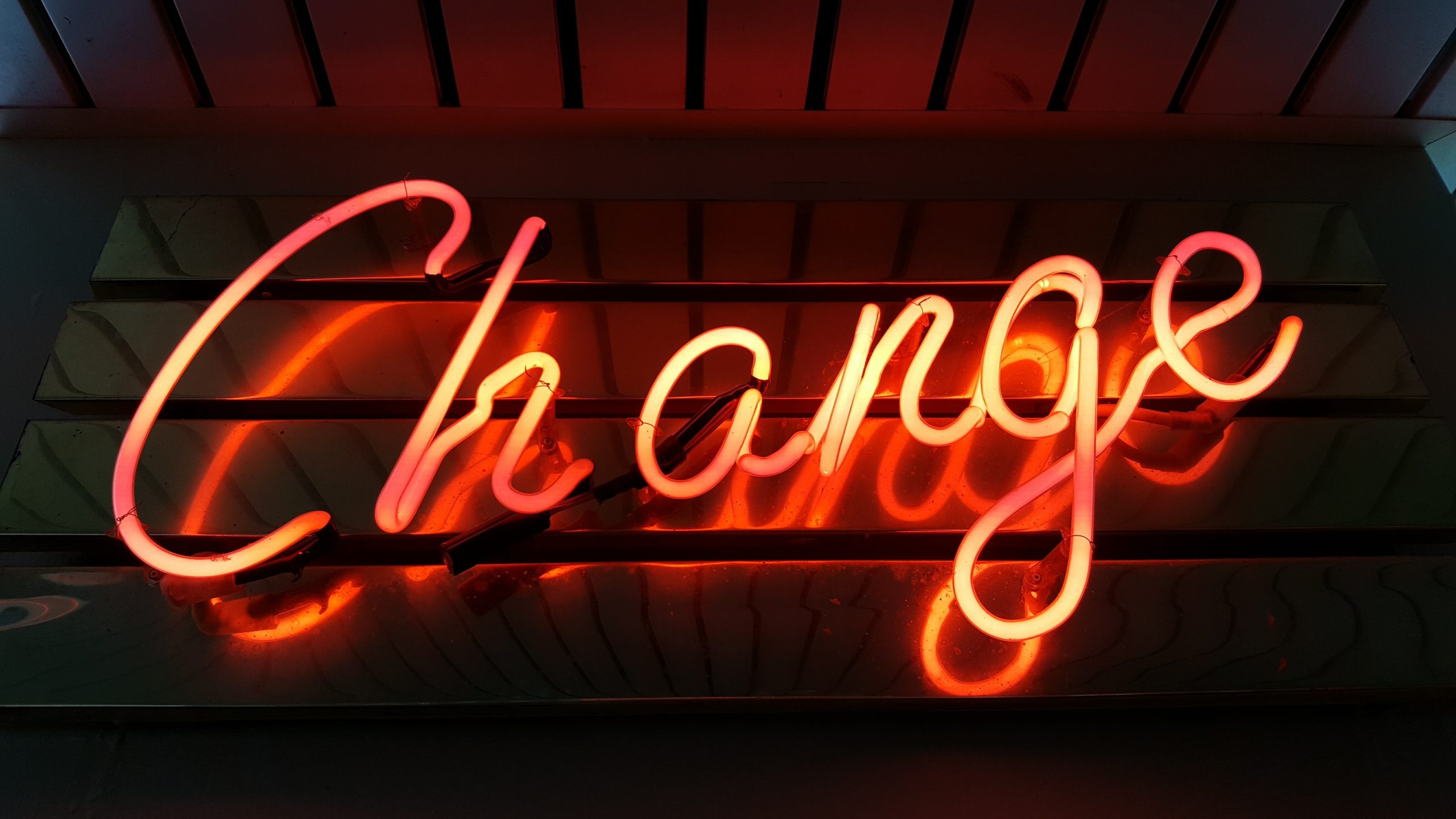
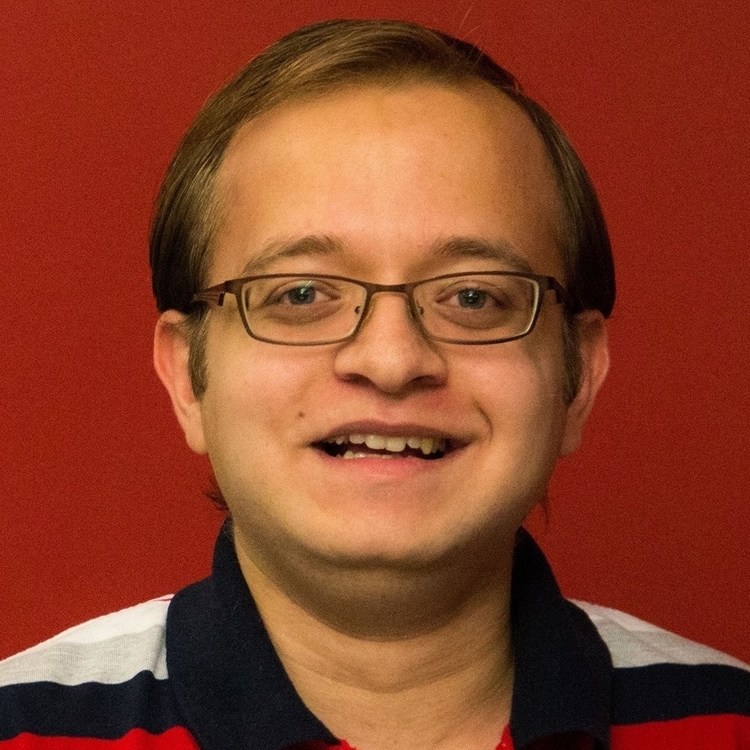
🔬 Research summary by Abhishek Gupta (@atg_abhishek), our Founder, Director, and Principal Researcher.
[Original paper by Abeba Birhane, Pratyusha Kalluri, Dallas Card, William Agnew, Ravit Dotan, Michelle Bao]
Overview: Machine learning is often portrayed as a value-neutral endeavor; even when that is not the exact position taken, it is implicit in how the research is carried out and how the results are communicated. This paper undertakes a qualitative analysis of the top 100 most cited papers from NeurIPS and ICML to uncover some of the most prominent values these papers espouse and how they shape the path forward.
Introduction
As we get a higher proliferation of AI in various aspects of our lives, critical scholars have raised concerns about the negative impacts of these systems on society. Yet, most technical papers published today pay little to no attention to the societal implications of their work. And this is despite emerging requirements like “Broader Impact Statements” that have become mandatory at several conferences. Through the manual analysis of 100 papers, this research surfaces trends that support this position and articulates that machine learning is not value-neutral. They annotate sentences in the papers using a custom schema, making open source annotated versions of the papers and their schema and code. The researchers used an inductive-deductive approach to capture the values that are represented in the papers. The researchers found that most of the technical papers focused on performance, generalizability, and building on past work to demonstrate continuity. There has also been a rising trend in the affiliations and funding sources for the authors of these papers to come from Big Tech and elite universities. Through these findings, the authors hope that technical research can become more self-reflective to achieve socially beneficial outcomes.
Key Ideas and Results
Methodology
The authors choose NeurIPS and ICML as the source of their papers because they have the highest impact (as quantified by the median h-5 index on Google Scholar), and conference submissions are a bellwether to judge where the field is headed and what areas of the field researchers care about and focus their efforts on as they form critical evaluative factors. Many of these papers are written to win approval from the community and the reviewers drawn from that community to achieve these goals. The annotation approach includes examining the content of the paper and creating a justificatory chain with a rating on the degree to which technical and societal problems serve as the motivation for the work. They also pay attention to the discussion of the negative impacts of the work as stated in those papers. The authors acknowledge that this methodology is limited because it is manual and can’t be easily scaled. They justify that by pointing out that automated annotation would be limiting in that the categories would be pre-encoded and subtleties will be lost that, for the time being, only human reviewers would be able to pick up on.
Findings
Values related to user rights and stated in ethical principles rarely occurred, if at all, in the papers. Other moral values like autonomy, justice, and respect for people were also noticeably absent. Most of the justifications provided for carrying out research point to the needs of the ML community with no relation to the societal impacts or problems that they are trying to solve. The negative potential of these works was also conspicuous by their absence. Though, some of those omissions might be the result of the taxonomy and awareness of the societal impacts of AI being more recently discussed, especially related to the analysis of the papers from 2008-09.
In terms of performance, the typical characterization for it is average performance over individual data points with equal weighting. This is a value-laden move as it deprioritizes those who are underrepresented in the datasets. In choosing the data itself, building on past work to show improvements on benchmarks is the dominant approach, but this presupposes a particular way of characterizing the world that might not be accurate, as demonstrated with many datasets codifying societal biases. The emphasis on large datasets also moves to centralize power because it shifts control over to those who can accumulate such data and then dictate what is included and what is not. The reliance on ground-truth labels in this case also codifies the assumptions that there is necessarily a correct ground-truth that is single-valued, which is not the case.
Representational harms arise from the excessive focus on generalization capabilities of the systems since it moves to disregard context and enforce a particular view of the world onto the rest of the incoming data in the interest of generalization. Efficiency is another value that is emphasized, but it is rarely discussed in the context of accessibility which could create more equity. Instead, it focuses on using fewer resources and scalability as the values that are the most important. The focus on novelty and building on previous work also entrenches existing positions further with limited critical examination of that prior work in the interest of continuity and demonstrating improvements on existing benchmarks rather than questioning if the benchmarks are representative in the first place.
Finally, the increasing influence of Big Tech and elite universities on the state of research is another avenue through which ethical principles are being sidelined and a specific set of values, as highlighted above, are being pushed into the research and development of machine learning. The current trend of treating machine learning as neutral creates insulation for the field in terms of critiquing the values that it espouses, both implicitly and explicitly.
Between the lines
This meta-analysis of the state of affairs in machine learning research is a significant contribution to better understanding where we are headed. In particular, the authors’ contribution of a data annotation schema and the set of annotated papers will be helpful for future analysis and research. Some developments that I’d like to see building on this work would be finding a way to scale this approach to have a more real-time analysis of where the field is headed and self-correct as we go along. A broader test of the inter-research agreement on the annotations would also be helpful. While the authors do indicate a high degree of inter-reviewer agreement through the Cohen Kappa coefficient, it would be interesting to see how that changes (if at all) when you get a broader set of people to take a look, especially those coming from a variety of fields (even though the authors themselves are quite diverse in the composition of this team).