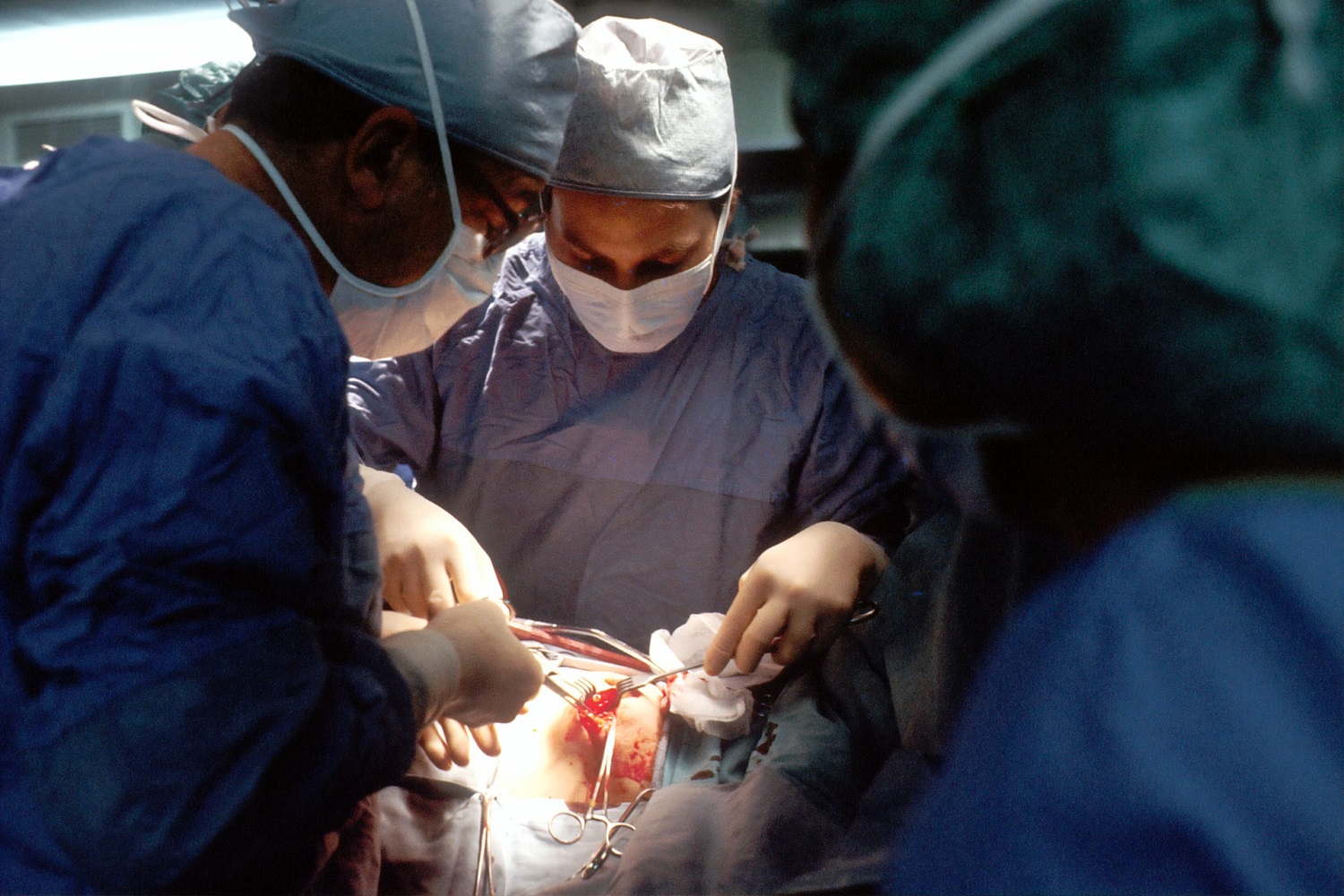
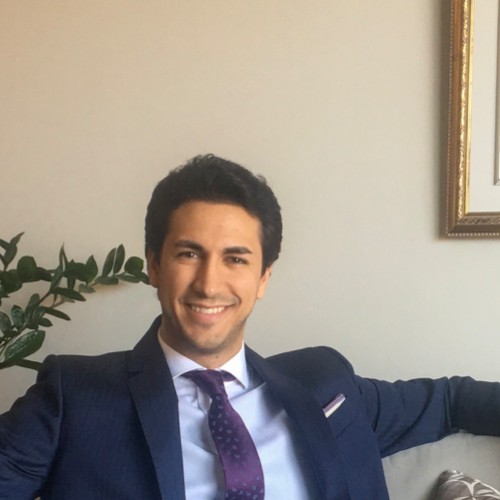
Article contributed by Jeremie Abitibol, Co-founder and CEO at Castella Medical.
The development of new computational methods and the heightening awareness around the value of artificial intelligence (AI) is finally starting to garner interest within the healthcare industry. The sensitive nature of healthcare services, however, creates medicolegal challenges and may entail particular ethical dilemmas for emerging AI solutions.
To discuss some of the challenges that might be faced by players in the data management and AI space, Jeremie Abitbol, PhD, CEO of Castella Medical, Inc., is speaking with Abhishek Gupta, founder of the Montreal AI Ethics Institute.
About Castella Medical
Castella Medical (“Castella”) is leveraging state-of-the-art video data management technology to scale a platform that enriches eLearning and facilitates the development of AI solutions for video-based medical procedures such as endoscopy, laparoscopy, and robotic surgery.
About the Montreal AI Ethics Institute
The Montreal AI Ethics Institute (MAIEI) is an international, non-profit research institute dedicated to defining humanity’s place in a world increasingly characterized and driven by algorithms. MAIEI’s goal is to build public competence and understanding of the societal impacts of AI and to equip and empower diverse stakeholders to actively engage in the shaping of technical and policy measures in the development and deployment of AI systems.
The Q&A below has been edited for clarity.
Jer: Hi Abhishek, I’m so happy we got acquainted and I want to thank you for taking the time to chat. Before I fire away, I’d love to hear more about the Montreal AI Ethics Institute and what inspired you to launch the organization?
Abhishek: The genesis started in 2017 at the United Nations AI for Good Global Summit, and what I saw was that Europe was much further ahead on some of these issues, and in Canada there were relatively no discussions on this subject back then. So I started up the AI Ethics community in Montreal, which still remains one of the only public consultation firms for this subject. It became formalized in 2018 and I guess we were lucky that we had the support of the entire community behind us and the institute has grown. We’re six people now at the Institute and one of the core pillars of our work is building public confidence so that’s where the ethics community comes into play. We consulted for the federal government in terms of amending their privacy legislations in Canada as it relates to AI, and we are doing the same for the provincial Quebec government as well. So you have that, and we’ve consulted for the Australian Human Rights Commission, the OECD, and we played a pivotal role in putting together the Montreal Declaration for Responsible AI.
Jer: Do you mostly consult to set up legal frameworks, say, for governments or quasi-governmental organizations, or do you also consult for the private sector and dive down to the nitty-gritty such as data handling policies and techniques and so on?
Abhishek: Both, it’s a comprehensive proposal. For example, the most recent one we did last week was for the federal government, so it was a 76-page document that we submitted that was both technical and legal, so a combination of both.
So that’s the work that we do. We also ran an internship cohort last summer, and actually one of our interns from last summer is now doing AI ethics for the Pentagon in the United States, so we were very happy about that, seeing the success of our program.
Jer: That’s quite impressive. So, as you know, our team is building a comprehensive platform that addresses a range of pain points for video-based medical procedures. And one of the core components of our mission is the development of AI tools to support real-time clinical decision making during these procedures.
Now, in doing our AI research earlier on, we noticed that some people were highlighting certain ethical considerations, and some of these issues struck a chord with our team, which led us to think that this is probably something that we would want to address from the outset. We figured that a lot of companies may develop these algorithms and then perhaps realize at some point that something wrong might have happened during the R&D process. By that point, maybe it’s too late or it would require considerable work to fix the problem. So we looked to incorporate some of these insights and expertise early on to help with the design of the kinds of algorithms that we’ll be developing, to inform our data collection strategies, and so forth.
Abhishek: I think that’s a great mindset. It’s sort of the principle in cybersecurity that at every subsequent stage of development, the costs of implementing cybersecurity measures jump by a factor of ten. So it’s a little bit along the same lines in terms of having to retrofit some of these ethical considerations – it’s prohibitively more costly further down the pipeline rather doing it right from the outset. It’s a little bit like baking it in rather than bolting it on later, right? So that’s the mindset and I’m happy to hear that you’re proactive about it because it’s something that’s very important, especially given the area that you are in.
Jer: I appreciate that.
Abhishek: As I was hearing about your work in AI, I think there’s a company in Montreal that’s doing something with AI in polyp detection?
Jer: Polyp detection is one application of endoscopy. It’s not something that we are focusing on in terms of the AI solutions that we are looking to develop as there are a few companies that are quite advanced in the polyp detection space already, so there’s no point in us reinventing the wheel. We are looking at different applications, but still in the endoscopy realm, so we aren’t competing with them, and if anything, our platform could actually help these companies streamline and modernize their processes for acquiring data.
Abhishek: That’s good, absolutely, there’s no point in trying to resolve the same problem.
Jer: You bet!
Abhishek: And what’s the composition of your team in terms of backgrounds?
Jer: I count myself incredibly lucky to be quarterbacking such an incredible, multidisciplinary, and experienced team. My background is in the evaluation of the clinical and economic implications of technology and how to improve a healthcare organization’s ROI [return on investment] with hi-tech. We have two co-founders who are serial entrepreneurs with deep expertise in the telecommunications field, software platforms, and business operations, including previous executive experience in a publicly traded company. We have engineers, including a machine learning developer. We have a truly all-star advisory board including key opinion leaders and world-renowned thought leaders in the medical field and in AI. So individuals with a range of expertise in different disciplines, but unified in our mission and our passion for healthcare and innovation, and we’re super excited to keep growing.
Abhishek: Of course, that’s critical to the success of the work that you do. Yeah, let’s talk AI.
Jer: Great! So, to start off more generally, obviously an algorithm or model can only be as good as the data on which it was trained (“garbage-in-garbage-out”). One AI-related ethical issue that seems to be making headlines across different industries is the potential of training algorithms on data that may contain biases, right? And once the algorithms are developed, potentially exacerbating those biases. It would be great if you could share your thoughts on this with our readers and how you think this could be addressed.
Abhishek: When we are talking about addressing biases in datasets, I would split that along two lines, and why I make that distinction is because you can either have primary data collection or secondary data use, and I see that you’re familiar with that. The problems are more severe in secondary data use, I would say, because a lot of the underlying assumptions are often unstated, so you don’t know what decisions were made during the data collection process. Are they storing raw video feeds or are they performing some sort of transformation on it? And even if it’s a simple change in the encoding of the video format, if that’s unstated between the raw and the processed video, what effect does that transformation have in terms of being able to do whatever task you’re trying to achieve? You don’t know what the impact is going to be unless you have that information upfront. So that’s an example. Another is how representative your data is. I’m no medical expert but let’s say you have differences across ethnicities or geographies, if the dataset doesn’t have that metadata in terms of what the distribution of that training data is, it’s again hard to say how that kind of data is going to creep in. So in terms of secondary data use there are all of these problems.
Now when it comes to primary data collection, I think being cognizant of the kind of target or people who will be the recipients of the decisions from those systems, those people need to be kept in mind. It can be beneficial to have two kinds of people that can provide guidance on that. One is the clinical expertise to understand the domain specificities – for example, someone who knows that it differs between men and women or it differs between people of African descent versus Asian descent or whatever. The second would be someone in the social sciences, and this is something that doesn’t happen as frequently, and someone who can give that intelligence in terms of the demographic mix of what the general distribution might look like. Most startups will not have a vision of just operating in one city but probably have a vision to scale across a country, hopefully the world, and serve as many people as they can, and in that scenario, having that intelligence ahead of time is very important. I think the degree of granularity should also increase as we go deeper into the development phase, so in the beginning it could be something very high level to give you a sense of where you need to place your focus in a general sense, but as you go deeper, maybe there needs to be a higher degree of granularity in terms of knowing what that demographic distribution is, and modelling that as part of the training dataset from the onset as you go deeper into the development phase, because of course something that requires effort and a very significant investment is not something you’re going to want to do upfront.
So, from a data collection phase I would say that that primary and secondary distinction is very important.
Then there’s the second phase, once you’ve collected all that data, with all the transformations that you do on that data. It’s very interesting in terms of how you clean and process that data. Any sort of transformations that you do should be documented, but more importantly, knowing and understanding what impacts these transformations have in terms of altering the distribution of the data. What I mean by that is often you’ll have pieces or certain attributes or features that you’re missing for particular data points. Let’s say we’re talking about two attributes: age and gender for hundreds of people. Let’s say you miss capturing the age for a few people for some reason, or maybe the person collecting the data didn’t do a good job. You have two options: you can make a guess and interpolate and fill out that data, or you can choose to eliminate that data point because it’s incomplete. Both of those approaches have consequences and I’m not saying one is better than the other but being aware of what the consequences are is important. So, if you’re interpolating, you’re making a guess – what you’re doing is altering the distribution. If they were distributed normally, maybe you’re shifting the curve one way or another, altering the mean and standard deviation by a certain amount if it’s significant – if it’s only a couple of data points maybe not. Now if you’re choosing to eliminate those data points maybe you’re altering the distribution again, but another thing that can happen is that you might be trimming out outliers or people that are underrepresented in the larger community to begin with. And in this example, let’s say these were people of certain minorities who were not comfortable sharing their age for some reason. If you were to eliminate those people and some of those features are not captured as part of your model during the training phase, when those people subsequently interact with that system, because the system never actually captured an adequate representation of these people.
Jer: It’s not validated for that population…
Abhishek: Yeah, the prediction it would make for them would be inaccurate. So that’s the consideration when processing and transforming data.
And then of course model selection in terms of the different decision boundaries that emerge as part of different model classes has an impact in terms of the thresholds that you choose. Of course a learning system will have thresholds that will vary and sometimes you want to go for a simpler model that maybe doesn’t have as high a predictive power but has a high degree of explainability versus one that is complex but isn’t as explainable, because for a practitioner who is going to administer the final decision, they want to be able to justify why they’re choosing to accept this decision.
So I guess that diverges away from the data bias question, but this sort of pervades the entire life cycle, so it starts with data collection and design, transformation, model selection, and also how that system learns in an online learning setting. The point that needs to be made there is to be careful about out-of-distribution data. What that means is that of course you can’t capture all of reality because any model is a simplification of reality, so when it encounters things that are outside of the typical distribution that you’ve been training on, is it going to attempt to give a decision or a prediction – which could be inaccurate because it has never encountered this before – or is it going to not give a decision or prediction at all saying “I’ve never encountered this before”? The reason it’s important to consider that is because when you encounter this sort of data that’s out of distribution, it’s almost always better to have some sort of guardrails in place that govern behavior than trying to make a best guess that might be inaccurate, especially in medicine. So being cognizant of this is very important and that data issue runs throughout the entire pipeline.
Jer: That makes perfect sense. Some researchers have found that changing so much as one pixel, or a few pixels, in an image can completely alter the output of neural networks. The famous example that has been given is how after changing a few pixels from an image of a cat, an advanced AI model – I think it was Google’s – accepted with 100% certainty that the image represented guacamole (avocado dip). Beyond cybersecurity standards, which you touched on briefly earlier, to prevent malicious actors from affecting AI outputs, perhaps you can speak to the sorts of guardrails that companies are advised to consider as part of AI R&D.
Abhishek: The example you are talking about falls in the realm of adversarial machine learning and machine learning security.
Jer: Exactly.
Abhishek: When we’re talking about those altered pixels that change the classifier output, we’re essentially looking at trying to jump over a line. Let’s say we want to identify whether something is blue or red, and there’s a decision boundary that separates all the blue examples from the red examples, essentially what adversarial perturbations do is they move you over that decision. So, let’s say there’s a line, it moves you over just a little bit so that it misclassifies the output. Now, the reason that these adversarial examples succeed is because there is an inherent brittleness in the representations of these learning systems in the sense that they’re very much dependent on what they were trained on. So you can craft malicious examples that can trigger a misclassification, which is what adversarial machine learning is all about. What you want to ideally be able to do is to have robustness in those models that needs to be done at the training and testing phase. The way you test for this is using these image recognition benchmark datasets that are increasingly available with these malicious examples that are not classified correctly, and you can test your model that you’ve trained against those examples and see if it holds up to get a sense of how resilient your system is against these malicious examples.
Then there are simple transformations like rotating a picture. One example is they took a picture of a bus that was lying sideways on a road and the system misclassified it as a snowplow. So you can have simpler transformations like rotating pictures and such, but the more serious test that I would say is a litmus test is using those malicious examples like altering pixels that don’t affect the picture overall.
Essentially, if you take the example of the cat and the guacamole, if I showed it to a human, with those few pixels that are altered you’d still say that it’s a cat – you won’t be fooled by it but a machine will be. Whereas the overturned bus, if you rotate the image, okay maybe even a human will have to look at it carefully before saying “oh it’s an overturned bus” and maybe tilt their head, so it’s not immediately obvious.
And that’s for image; you have the same thing for text, for voice, and the list goes on. Those are the crafted malicious examples that you need to be able to address and have as a part of your testing phase when you have your machine learning models. Ideally you don’t want to have performance only in terms of accuracy of prediction – classifying a cat as a cat – but also in terms of resiliency to these maliciously crafted examples and being able to correctly classify them or evade those sorts of attacks.
The other part is sort of general cybersecurity practices that apply within the space of machine learning and there are guidelines such as from NIST [National Institute of Standards and Technology, United States Department of Commerce] in terms of cybersecurity, a lot of which still matter in the machine learning sphere as well. An example is sanitization of input – being careful of what you feed into your system to begin with is something that it’s important. Again, for public facing systems it might not always be possible having that in place. An example that comes to mind is the Tay chatbot that Microsoft put in place in 2016, you know the one I’m talking about?
Jer: Mhm, it was racist.
Abhishek: That’s right, they fed it racist tweets and it turned racist because it learned it from those tweets. So, that’s what I’m talking about when I’m saying sanitization of inputs. If a filter in place already figured out “hey, these are racist tweets, I’m not meant to take that as a part of the input to train my system” —
Jer: You actively remove it.
Abhishek: Right, so you’re proactive about it. It’s just good cybersecurity practice. And there are many principles that apply and one of the ones that I think is particularly important is this idea of defense in depth.
Jer: That’s right.
Abhishek: Defense in depth is essentially saying, let’s say you think of a bank that stores cash, you don’t just have one guard that stands in front of a pile of cash, you have numerous measures in place including multiple keys that need to be put in place, concrete walls, steel doors, and a security guard, and surveillance cameras, etc. There are multiple measures, so it’s defense in depth in that you have multiple defensive measures successively in the system. A similar practice needs to be adopted for the machine learning world as well where you don’t just rely on sanitization of input, or being able to evade malicious examples, you apply all those examples collectively to secure your system.
Jer: Absolutely, it’s crucial to be aware of the multitude of tools that can help safeguard these systems, and the added effectiveness of a layered security approach. Now at the moment, machine learning and predictive analytics in healthcare are mostly being commercialized as “support tools” for healthcare professionals, rather than computerized decision making. There are some who do fear an over-reliance on such tools for different reasons, including clinical validity and implications for the training of future healthcare providers. What are your thoughts on this?
Abhishek: In the case of the decision support system with the clinician being this filter before giving it to the patient, there’s still a risk of what I call “atrophy of skills” where people can start to become overly reliant on a system that gives them the right advice and, as you said, if something is contrary, to not question it. We can think of the GPS as a toy example. Maybe growing up you drove a car without a GPS and you know the best way around your hometown in terms of going from your friend’s place to your place. But now the GPS gives you a better route, and after years of training of the human in terms of being reliant on the GPS to tell you which roads to take, you forget that you already know the best route to get to your friend’s place, so you rely on the GPS and, presumably, most of the time it tells you the right thing but maybe sometimes it doesn’t, but we stop questioning it. We lose that practicality, we lose that independence of thought, we lose that skill in terms of making an independent decision. And I fear that that may happen with these clinical decision support systems where clinicians will, over time, become overly reliant and not question enough the outputs from the system, which defeats the purpose of having the clinician there in the first place because then you might as well just directly give the diagnoses or whatever to the patient.
Jer: On a similar note, I know you mentioned earlier this approach about reaching out to people in the social sciences, right? There was an article two years ago on the ethical dilemmas that may be faced by algorithms operating autonomous vehicles. The point that our team found very intriguing is that the computer programmers on this research team were joined by philosophers and psychologists at the R&D stage. At Castella, we put a great deal of emphasis on using an interdisciplinary approach to problem solving. Still, despite the value of such diversity in the workplace, it seems as though people with training and experience in the liberal arts are really few and far between in the AI world. Do you agree that companies in the AI space could benefit from working harder to get people on board with such experience and mindsets to consider these ethical perspectives more profoundly, and to even work in tangent with the programmers and so forth?
Abhishek: Absolutely! When we have a diversity of opinions and experiences and backgrounds, the “product” will be more robust in terms of all those concerns, be they ethical or technical. I guess you can’t demand people who have technical training to also have expertise in moral philosophy and ethics because there’s just such a vast body of knowledge that it’s just not feasible for a single person to have, and so folks from other backgrounds can bring in different sets of expertise. And that realization is something that’s gradually dawning on the community.
Jer: Thank you Abhishek! I really appreciate you taking the time to chat and to help shed some light on a lot of these points.
Abhishek: Thank you!