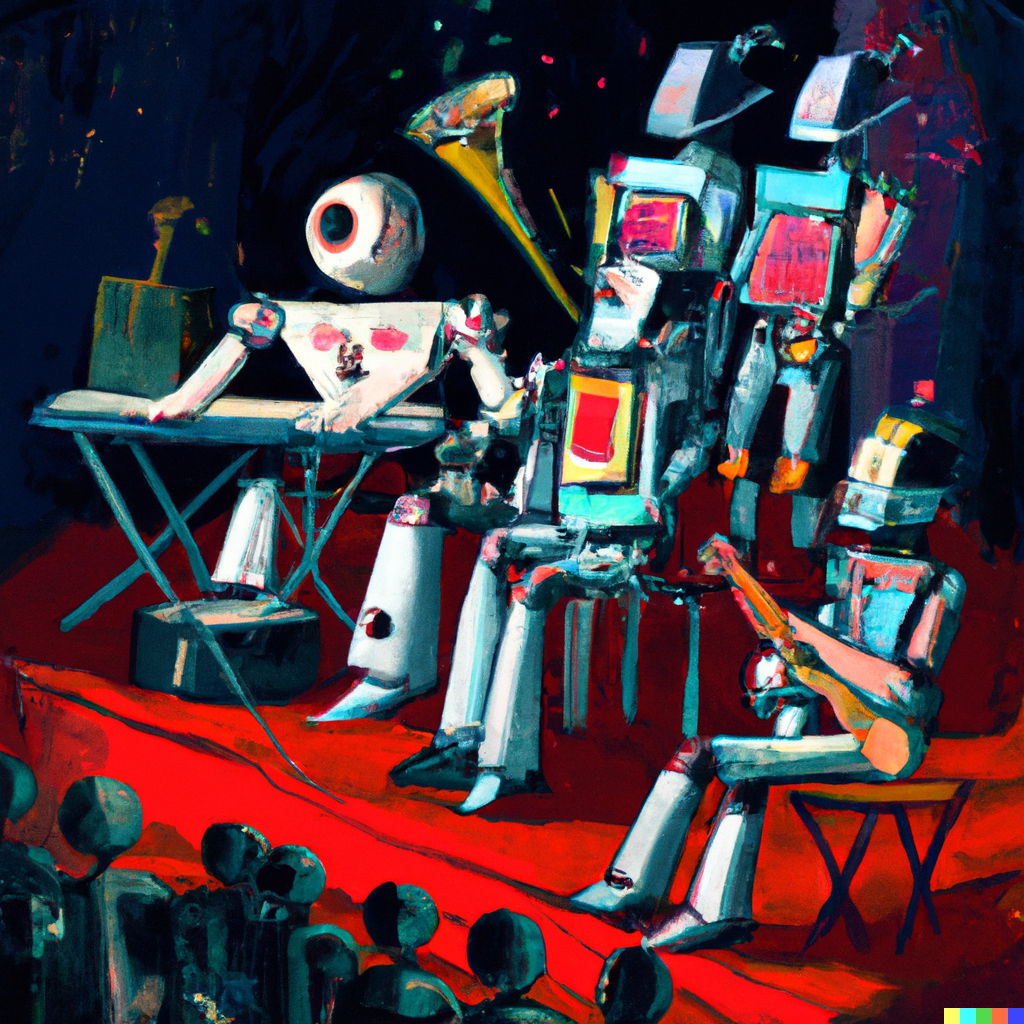
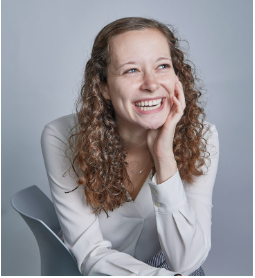
🔬 Research Summary by Julia Barnett, a PhD student in Technology and Social Behavior, a dual PhD program in computer science and communications at Northwestern University, whose research aims at reducing the socio-technical harms of algorithmic systems.
[Original paper by Julia Barnett]
Overview: This paper analyzes an exhaustive set of 884 papers in the generative audio domain to quantify how generative audio researchers discuss potential negative impacts of their work and catalog the types of impacts being considered. Jarringly, less than 10% of works discuss any potential negative impacts—particularly worrying because the papers that do so raise serious ethical implications and concerns relevant to the broader field, such as the potential for fraud, deep-fakes, and copyright infringement.
Introduction
Generative audio modeling is a growing area of research with recent models having human-like quality in their audio output for both music and speech generations. Recent work needs only 10 seconds of a speaker’s voice to create high-quality realistic text-to-speech audio generation (Kim et al. 2022) that could easily be used in deep fakes or phishing. In generative music, a new model made by Google now allows us to make new pieces of music by inputting highly detailed text descriptions (Agostinelli et al., 2023). However, it’s hard to say when these models produce outputs with substantial similarity to their training data of potentially copyrighted works or scraped songs from artists without their consent.
The creators of both of these models announced they had no intention to release their models to the public due to the strong potential for misuse. However, they are in the small minority of generative audio researchers who discuss any potential negative impacts or ethical considerations of their creations, and out of the 171 full-text research papers analyzed in this study, they were among the lone 9% of papers that mentioned negative impacts even once in their papers.
Key Insights
What Are Generative Audio Models?
Generative models have been a large focus of AI researchers over the past few years, and recently society has seen these models first-hand in public facing algorithms like ChatGPT for text and DALL-E 2 for images, but generative audio models have fallen a bit under the public radar. At their core, generative models use a large amount of training data to predict something similar to and statistically likely to exist in the dataset it was trained on; in generative audio models, this means they typically train on some sort of music database to create new songs or speech database to create human-sounding speech. Some audio models you can play around with that you may not have heard of are MusicLM and AudioLM, a text-to-music and a text-to-speech model, respectively.
Researcher papers in this domain often have one big gap: they do not tend to discuss potential negative impacts. This is not for lack of considering any potential impact; the author found that 65% of the papers analyzed talked about some potential positive implications of their work. They just neglected to mention any potential negative impacts.
Different Negative Impacts of Generative Audio
In addition to quantifying the degree to which researchers in the field discuss ethics and negative impacts, the author also strove to catalog the different types of ethical considerations discussed in the small percent of papers that did so. These are split into negative broader impacts in generative music models, generative speech models, and those present in both areas.
Generative Music
One of the most important considerations of generative music models—ethically and potentially legally—is copyright infringement. It is widely established that generative models can memorize and reproduce information from their training data, and it stands to reason that these models could recreate copyrighted material.
The most common potential negative impact discussed in the corpus was the stifling of creativity due to AI music generation, which focused on the repetitive nature of the music generation and that limiting the creative output to possibilities of the model may result in a similar bound on human creativity. Another issue concerns the loss of agency and authorship that human creators can feel when creating music with the assistance of an AI generative model.
Machine learning models often perpetuate biases in the training data, and generative models are no different. It is important to be aware of the composition of the training data to
understand what biases could be perpetuated; models trained on Western music will perpetuate the biases of Western culture. Additionally, generative audio models sometimes
train on incomprehensible amounts of training data, and it follows that some of this data come from cultures outside the algorithm’s creator or users of the model. A fundamental lack of understanding of model attribution will result in cultural appropriation if the training data contains content from marginalized communities.
Generative Speech
Models that can accurately recreate human-sounding voices, especially of a targeted speaker (think: someone’s child or grandma), have enormous potential to be misused in cases of phishing and fraud. Some of these models only need 10 seconds of someone’s voice to train on. A slightly nuanced aspect of speech generative models’ ability to impersonate victims exists
when the victims are famous, and the model misuse can take the form of misinformation or deepfakes. As these models continue to become easier to use, the prevalence of deepfakes and misinformation online will continue to grow. There are also security and privacy concerns in the form of machine-induced audio attacks on intelligent audio systems, such as hidden voice commands that can manipulate voice-protected or operated systems.
All Audio Models
One concern for all generative audio models is the energy consumption of these models. There are two types of energy consumption of a generative model: the energy required to train and to generate samples. Current research points to machine learning models at risk of significantly contributing to climate change. It proposes the total energy consumption and carbon emissions of training these models be reported alongside the other standard suite of metrics like accuracy and speed.
There are certainly more ethical considerations than those detailed above, but these are some of the main ones already being discussed by researchers in the field. We can and should continue to build this list of considerations as we continue to build these models.
Between the lines
Generative audio models are not going away—they will only continue growing. It is essential to consider these impacts going forward at all stages of research: during the design process, the implementation of these models, and their publication and publicization. Two papers in the corpus explicitly mentioned that they did not intend to release their models or code due to the potential for misuse by bad actors. This is a viable consideration for model creators to make and should not be taken lightly.
This is an agenda-setting paper at the right time—it is important to both diagnose the degree to which research papers on generative audio models are discussing ethics and encourage the plethora of researchers to come to contemplate these negative broader impacts in their future work before the field being clogged by studies without ethical consideration.