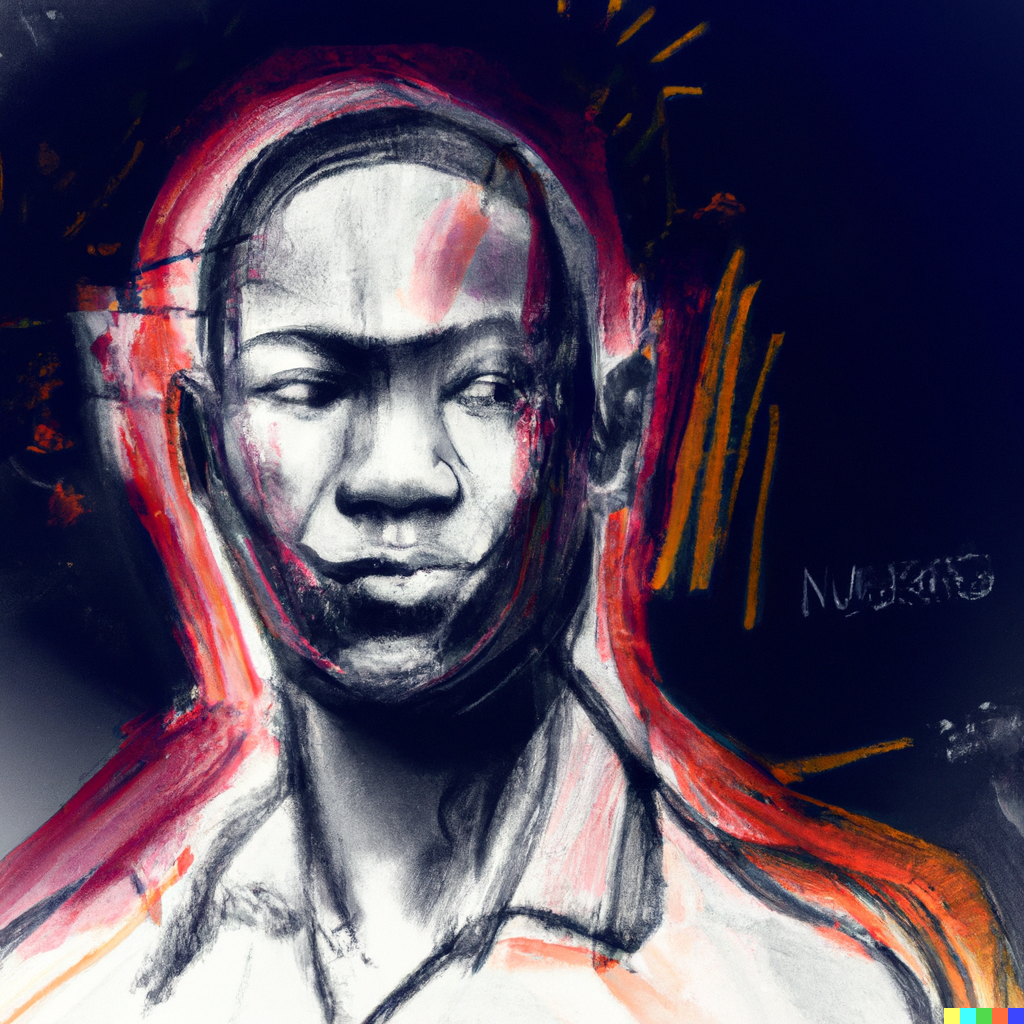
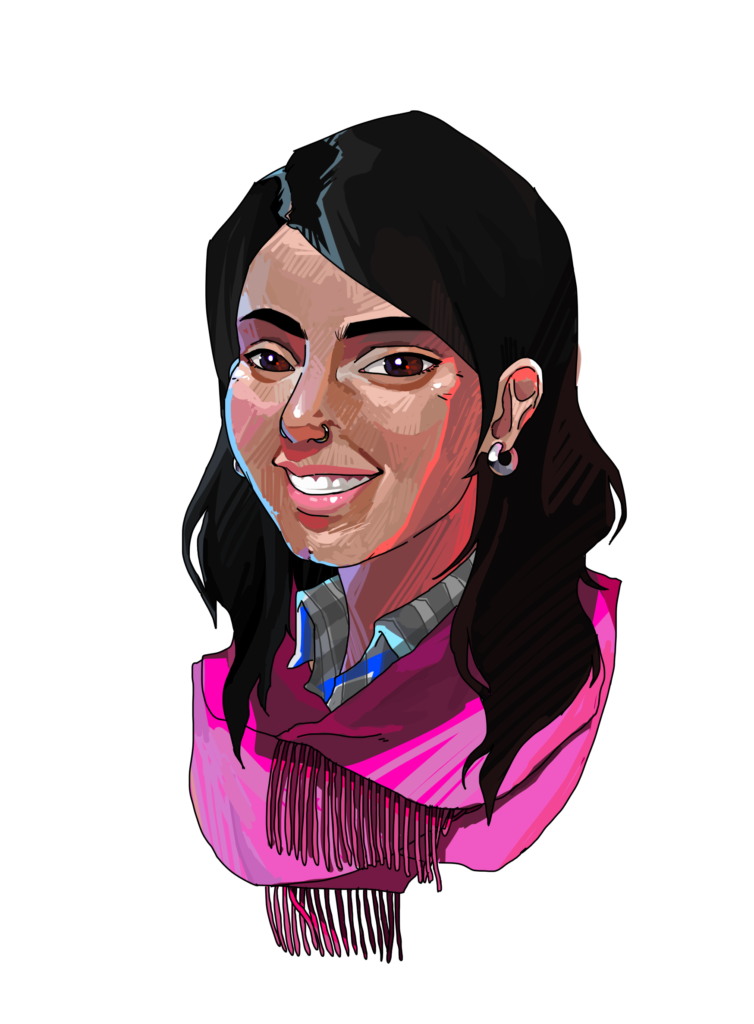
🔬 Research Summary by Shreyasha Paudel, a Ph.D. student at the University of Toronto with an interdisciplinary research focus that combines Human-Computer Interaction with critical theories from social sciences. Her current research looks at the use and impact of data, digitization, and algorithms in developing countries in applications related to crisis informatics, disaster risk reduction, civic technologies, and climate adaptation.
[Original paper by Milagros Miceli, Julian Posada, and Tianling Yang]
Overview: This paper borrows ‘Studying Up’ from Anthropology to make a case for power-aware research of Machine Learning datasets and data annotation practices. The authors point out the limitations of a narrow focus on dataset bias and highlight the need to study the historical inequalities, corporate forces, and epistemological standpoints that create existing data design and production practices along with the embedded bias.
Introduction
News headlines about biased datasets and output in AI systems have become increasingly common as more and more ML systems are integrated into public-facing products. Bias and ways of mitigating bias in AI are also increasingly an area of focus in academic research. This paper argues that such a narrow focus on bias is incomplete and insufficient to address algorithmic harms fully. The authors argue that the study of bias locates the problem within technical systems and obscures its root causes. Instead, the authors argue for an epistemological shift towards more interdisciplinary research investigating the context and economic imperatives guiding data creators, annotators, and users.
The authors follow an infrastructural approach to studying technology and borrow the “Studying Up” concept proposed by anthropologist Laura Nader. In anthropology, studying up means expanding the field of inquiry to study the elites occupying power positions. Similarly, the authors here take the case of crowdsourced data annotation work and examine the labor conditions, institutional practices, infrastructures, and epistemological stances encoded into datasets. They discuss how research documenting data bias and addressing worker bias is insufficient and misguided. As an alternative, they point to prior research in Human-Computer Interaction (HCI) and make a case for a more comprehensive understanding of datasets as socio-technical systems. They conclude with three suggestions for researchers on ” studying up” machine learning data in a power-aware way.
Key Insights
The Distraction of Debiasing “Data”
In this section, the authors point out the limits of current research focus around debiasing data. They argue that discriminatory outcomes from machine learning systems are as much a product of social structures, problem definitions, and a belief in the neutrality of algorithms as the existing bias in datasets. The premise that data can be neutral and debiased is the same premise that leads us to believe in the neutrality and superiority of algorithms and quantification. By their very nature, machine learning systems are designed to cluster and classify data, and data is always a product of subjective and asymmetrical social relations. Thus, designing and using ML algorithms is always value-laden and subject to interpretation. Harms from these algorithms do not simply manifest as a result of biased data but are a product of existing power asymmetries: “the power to decide how systems will see and classify, what data is worth including, and whose data we can afford to ignore.” Debiasing data still requires a value-driven agreement on the desired outcomes and ideal representation of the said outcomes. Therefore, instead of focusing on bias and debiasing, the authors argue for examining the values and subjectivities embedded in datasets by tracing the links to historical and ongoing symmetries.
Power Differentials of “Data Work”
Recent research has focused on the individual subjectivities of annotators and proposed frameworks for reducing noisy labels and creating higher-quality data. In this paper, the authors point out the flawed premise of such work using a power-aware lens. Most data annotators are at the bottom of hierarchical labor structures, and they collect and label data within organizational structures and predefined conventions given to them by managers and clients. The authors argue that social and labor conditions affect workers’ dependency on data work, which can potentially prevent their ability to raise questions about annotation instructions and tasks. Similarly, business incentives and profit-driven imperatives, the role of surveillance enabled and enforced by technology-mediated platforms, and the design choices of clients and managers often shape data work as much as the subjectivities of the data workers. The authors argue that researchers could study and expose fundamentally different issues regarding bias in data work if they used a lens of power and examined annotation and annotators as part of a networked system of dataset creation.
Data Documentation Beyond Bias Mitigation
This section highlights several frameworks and tools for documenting machine learning datasets, such as Datasheets for Datasets, Model Cards for Model Reporting, Dataset Nutrition Labels, and Data Statements for Natural Language Processing. While the authors agree that these frameworks help diagnose biases, they argue that merely listing biases in the composition of a dataset without interrogating the political nature of the data is insufficient to reduce harm. This process can be harmful if they naturalize and reify social categories such as race. In the paper, the authors propose that current approaches to data documentation could be improved by providing context around annotation workflows and documenting conflicts, disagreements, or assumptions used during data collection and annotation. Some of these contexts can only be discovered by examining the relationships between data workers and requesters. Many open datasets widely used today were produced for a particular purpose by researchers or corporations, and the data collection, cleaning, and annotation pipeline is often designed around the said purpose. Therefore, the authors argue for expanding data documentation beyond documenting data’s technical features and including the production contexts and dynamics of power that shape datasets.
A Research Agenda to Study Up
The authors conclude by proposing a power-oriented research agenda to study datasets and data work in Machine Learning along the following three lines:
- Researchers should conduct more qualitative and ethnographic research on data workers and data work production to understand the geographical and cultural contexts, workflows, infrastructural, and other incentives/constraints that affect the data work.
- Secondly, along with the annotators, the authors point out a need to study the motivations and incentives of ML practitioners who outsource the data work. Often, these requesters are the customers for data annotation platforms and shape the service relationships and market logic that shape annotation work.
- Along with the workers and the requesters, the authors also propose expanding data documentation research to incorporate power relations in data production contexts. They argue for research into documentation frameworks that can prioritize the needs and workflows of the workers and bridge the existing power gap in data work.
Between the lines
This paper borrows the “studying up” concept from anthropology and shows a potential path for AI ethics work to examine challenges and bridge power differentials in practice. The authors’ proposal to expand the study of AI harm and harm mitigation beyond the models, code, and datasets to the structures of power they operate in is a useful and necessary step for AI ethics-related research to truly address the injustice and harm that data-driven systems can produce.