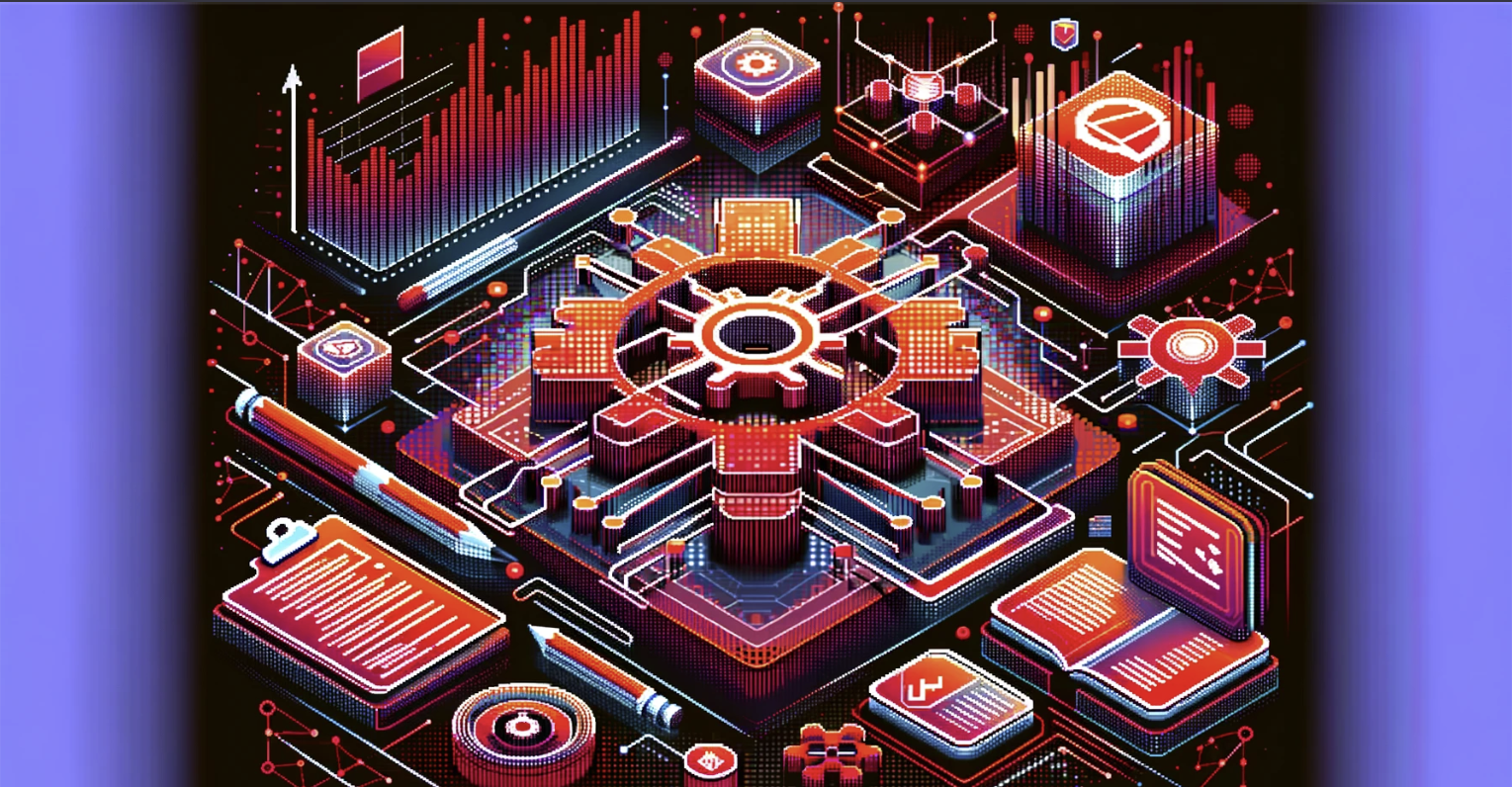
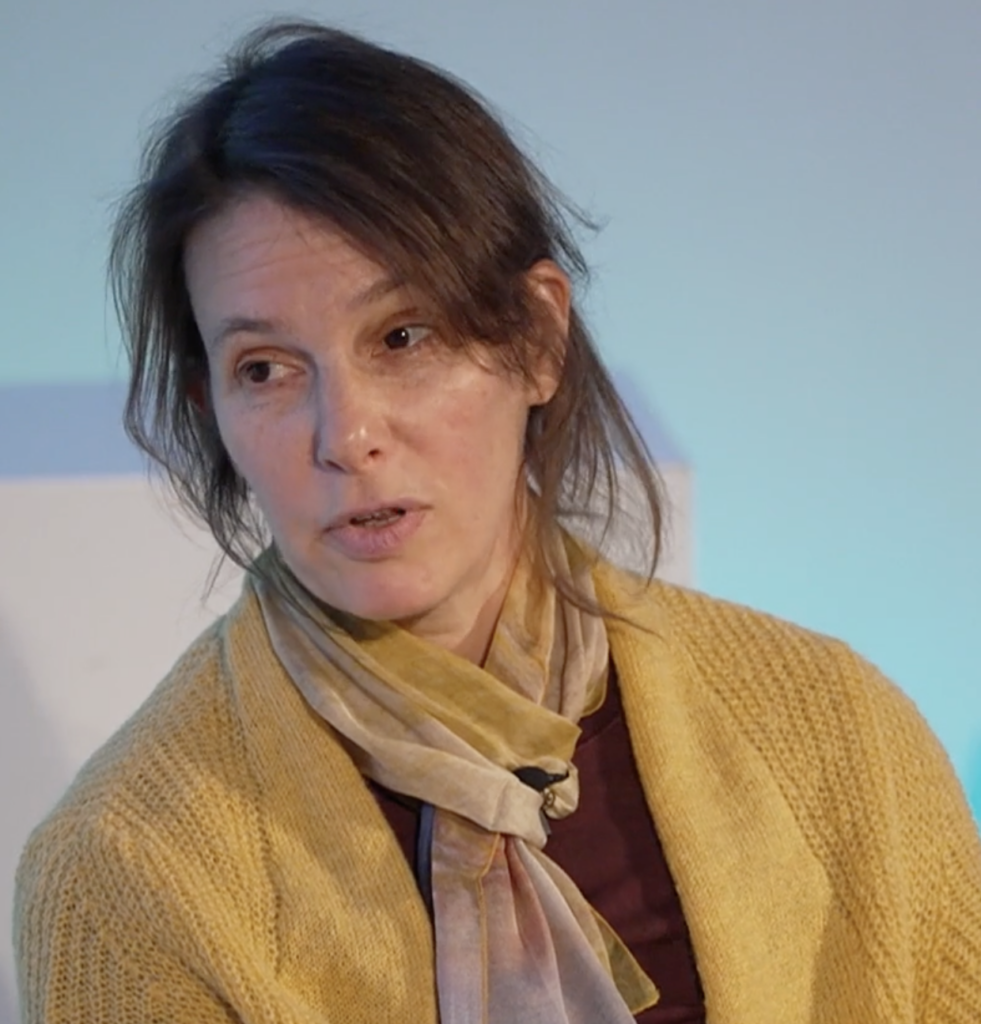
🔬 Research Summary by Kate Kaye, a researcher, author, award-winning journalist, and deputy director of the World Privacy Forum, a nonprofit, non-partisan, public-interest research group. Kate is a member of the OECD.AI Network of Experts, where she contributes to the Expert Group on AI Risk and Accountability. Kate was a member of UNHCR’s Hive Data Advisory Board and was selected in 2019 by the Montreal AI Ethics Institute as part of a multidisciplinary cohort of interns researching the social impacts of AI.
[Original paper by Kate Kaye and Pam Dixon]
Overview: Currently, policymakers in governments and organizations worldwide are moving beyond theoretically responsible AI principles toward implementation. How? By establishing AI Governance tools. These tools will affect how we build, use, understand, quantify, improve, judge, and regulate AI systems for years to come. In Risky Analysis: Assessing and Improving AI Governance Tools, researchers from the World Privacy Forum take an interdisciplinary approach to assessing existing AI governance tools and provide concrete pathways for creating a healthier AI governance tools ecosystem.
Introduction
The impulse for policymakers to present practical ways to measure and improve AI is positive. But as the World Privacy Forum shows in Risky Analysis, there’s already cause for concern. A key finding of the report demonstrates that some AI governance tools used for fairness and explainability feature faulty AI fixes and off-label uses of measurement methods that could introduce new problems.
In fact, the report finds that more than 38% of 18 AI governance tools reviewed either mention, recommend, or incorporate off-label, unsuitable, or out-of-context measures when applied to evaluate or improve AI systems.
How did researchers arrive at this finding? In addition to surveying existing AI governance tools established in Africa, Asia, Europe, Latin America, and North America, the report reviews scholarly technical and socio-technical literature addressing specific problematic AI fairness and explainability methods. Researchers discovered flaws in the nascent AI governance tools ecosystem by analyzing AI governance tools through this scholarly lens.
Ultimately, the Risky Analysis report aims to help build evidence toward improving the AI governance tools ecosystem.
The report also aims to help advance and articulate the discussion around AI governance by introducing a definition of AI Governance Tools and a lexicon of AI Governance Tool Types. Researchers used the evidence from the survey of tools in conjunction with in-depth case studies and scholarly literature review to construct the lexicon of AI governance tool types. Based on the evidence gathered, these tool types include practical guidance, self-assessment questionnaires, process frameworks, technical frameworks, technical code, and software.
Key Insights
In Risky Analysis: Assessing and Improving AI Governance Tools, readers will learn about various AI governance tools from Africa, Asia, Europe, Latin America, and North America. Rather than formal legislation, AI governance tools are the methods and mechanisms used to implement AI governance and put responsible AI principles into practice.
AI governance tools are defined in the report as “socio-technical tools for mapping, measuring, or managing AI systems and their risks in a manner that operationalizes or implements trustworthy AI.” The tools reviewed therein take many forms, from practical guidance, self-assessment questionnaires, and process frameworks to technical frameworks, software, and technical code.
Some examples of AI governance tools reviewed:
- A vast repository of AI governance tool types from The Organization of Economic Cooperation and Development (OECD), a multilateral institution
- An updated process for the acquisition of public sector AI from Chile’s public procurement directorate, ChileCompra
- Self-assessment-based scoring systems from the Governments of Canada and Dubai and Kwame Nkrumah University of Science and Technology in Ghana
- Software and a technical testing framework from Singapore’s Infocomm Media Development Authority (recently expanded for evaluation of generative AI systems such as Large Language Models)
- An AI risk management framework from the US National Institutes of Standards and Technology
- A culturally sensitive process for reducing risk and protecting data privacy throughout the lifecycle of an algorithm from New Zealand’s Ministry for Social Development
A Lexicon of AI Governance Tool Types and AI Governance Tools Comparison Chart
The report introduces a definition of AI Governance Tools and a Lexicon of AI Governance Tool Types to contribute to the nascent conversation around the emerging AI Governance Tools ecosystem.
A related AI Governance Tools Comparison Chart spotlights key features of select mature AI Governance Tools. A Map of Global Data Governance and a Chart of Regional Global Data Governance Trends offer even more context.
Spotlight on Problematic Fairness and Explainability Methods in AI Governance Tools
The report spotlights specific fairness and explainability methods criticized in scholarly literature that are mentioned or promoted in some AI governance tools. The first involves encoded translations of a complex US rule called the “Four-Fifths rule” or “80% rule” in attempts to automatically alleviate bias from disparate impacts of AI systems. The report also spotlights SHAP and LIME, two related metrics intended to explain how AI systems produce particular outputs or decisions, both of which have attracted scrutiny among computer science researchers despite their popularity.
The Four-Fifths or 80% Rule
The rule is well-known in the US labor recruitment field as a measure of adverse impact and fairness in hiring selection practices. It is based on the concept that a selection rate for any race, sex, or ethnic group that is less than four-fifths—or 80% of the rate reflecting the group with the highest selection rate — is evidence of adverse impact on the groups with lower selection rates.
Although the rule was not designed to measure disparate impacts in AI or AI fairness outside US employment contexts, it has been repurposed in computer code form and used out-of-context to automate disparate impact measurement and AI fairness more broadly.
SHAP and LIME
The report also found examples of two problematic AI explainability measures mentioned or promoted in several AI governance tools. The measures – called SHAP and LIME — are commonly used. However, they have attracted an abundance of criticism from scholars who have found them unreliable methods of explaining many complex AI systems.
In a typical use case, an AI practitioner might employ SHAP or LIME to explain a single instance of an AI model output, such as one decision or prediction, rather than the whole model. However, they may produce misleading results because both methods work by approximating more complex, non-linear models (often called “black-box” models) with more straightforward linear models.
In addition to providing in-depth detail in two use cases spotlighting The Four-Fifths or 80% Rule and SHAP and LIME, the report also presents a compendium of the potential risks of automating fairness.
Key Finding: Faulty AI Fixes and Off-Label Measures
The impulse for policymakers to present practical ways to measure and improve AI is positive. However, as noted above, the Risky Analysis report found cause for concern. In fact, a detailed analysis of 18 AI governance tools found a worrisome statistic:
More than 38% of 18 select AI governance tools reviewed either mention, recommend, or incorporate off-label, unsuitable, or out-of-context measures when applied to evaluate or improve AI systems.
AI Governance Tools and the Evolution of Privacy and Data Protection
As the report suggests, it is too early to tell how or even if any current data privacy law or techniques, such as Privacy Enhancing Technologies (PETs), will apply in the impending AI era. Policymakers and others working to protect data in relation to AI will need to change the way privacy, human rights, and data governance are operationalized and implemented to meet the new challenges brought forth by rapidly advancing AI systems.
But in these in-between times, AI Governance Tools are helping fill the gap by providing measurements, procedures, and other mechanisms intended to implement privacy and data protections in the context of AI use.
Pathways to a Healthier AI Governance Tool Ecosystem
Like most areas of AI governance, the AI governance tool ecosystem is developing rapidly. However, the report suggests it is not too late to recalibrate by establishing sound approaches for best practices and standards for quality assessment. The report suggests concrete steps to take toward this goal:
Gather evidence – Evidence built up over many years shows what works and what does not when governing and protecting the data privacy of individuals, groups, and communities. The report suggests gathering necessary evidence and setting up measurement environments to facilitate this work to avoid “just making guesses.”
Include documentation – Many AI governance tools lack the appropriate documentation necessary to establish transparency and accountability and ensure they are applied properly. It would be helpful if these tools routinely included information about the results of quality assurance testing and instructions on the contexts in which the methods should or should not be used. Documentation will also help identify those who finance, resource, provide, and publish AI governance tools and help prevent conflicts of interest.
Create an evaluative environment – Multilateral organizations and standards bodies can help to foster a multi-stakeholder environment in which an evidentiary basis for AI governance tool best practices is created. Such groups can help develop recommendations for producing, evaluating, and using AI governance tools.
Between the lines
Not unlike how we measure the climate or the economy, the AI governance tools we establish today to measure and improve AI systems will form the foundation for how we understand and govern these systems for years to come. They could be the basis of AI risk scores, fairness ratings, or other statistics we rely on to help make sense of AI systems and enforce the laws and regulations addressing them. This is why it is essential to establish sound and effective methods to assess and measure how fair, explainable, reliable, privacy-protective, or safe AI systems are. As the Risky Analysis report suggests, there is a window of opportunity to foster a truly healthy and reliable AI governance ecosystem today.