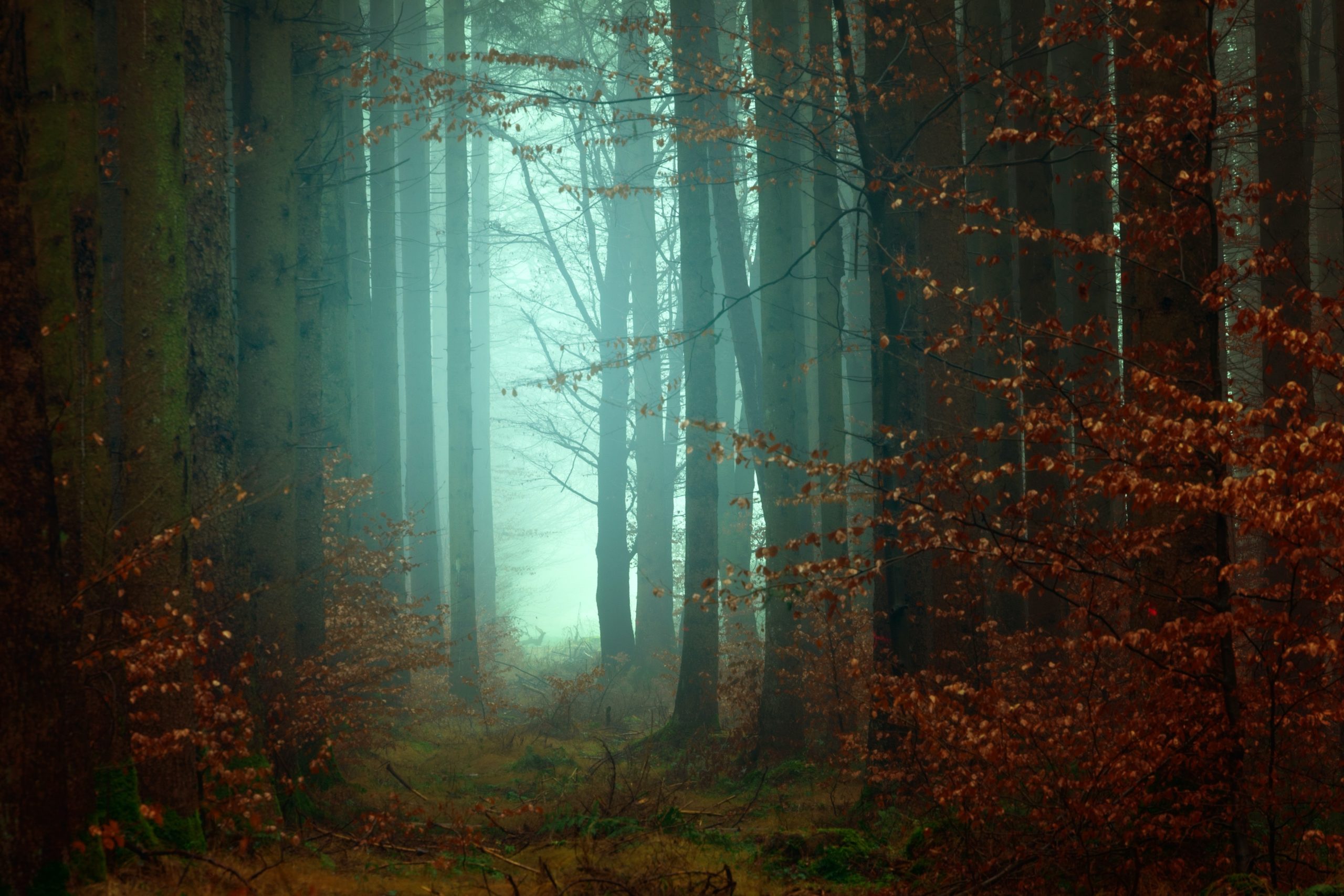
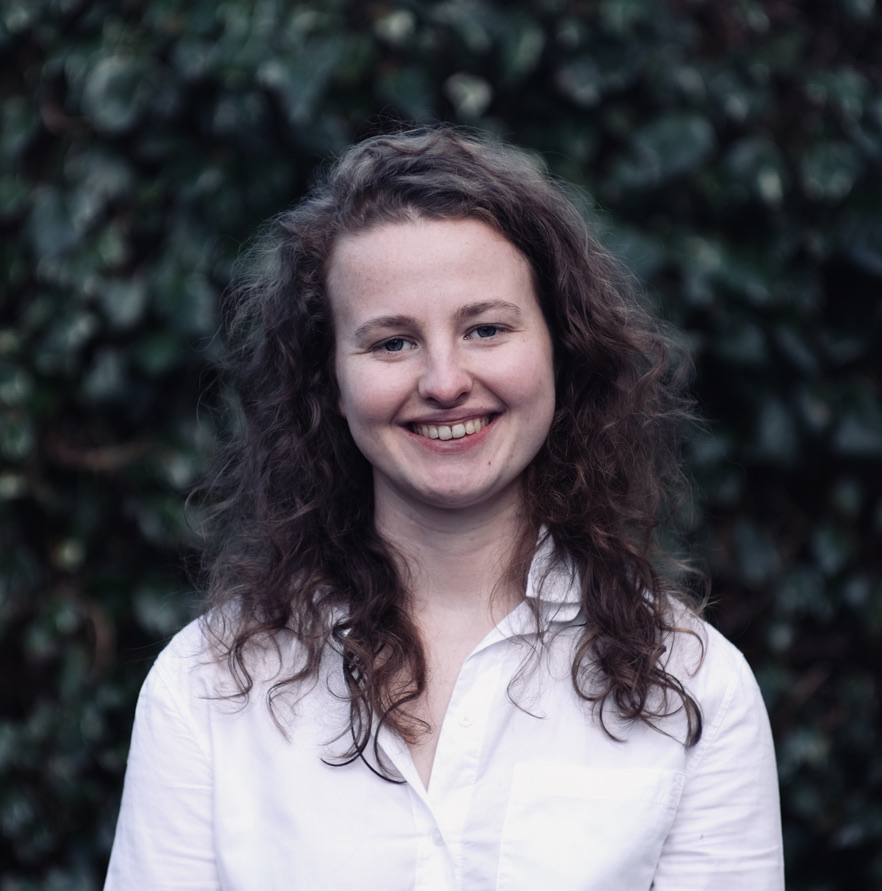
🔬 Research Summary by Eryn Rigley, a PhD research student at University of Southampton, specialising in the intersection of environmental and AI ethics, as well as defence & security AI ethics.
[Original paper by Jan‑Hendrik Heinrichs]
Overview: The multitude of AI ethics guidelines published over the last 10 years take for granted certain buzzwords, such as ‘responsibility’, without due inspection into their philosophical foundations and whether they are actually fit for purpose. This paper offers a challenge to the notion that ‘responsibility’ is suitable and sufficient to AI ethics work.
Introduction
We have all seen the AI ethics buzzwords by now: ‘explainability’, ‘transparency’, and the big one, ‘responsibility’. But what do these buzzwords offer in practice? This paper challenges the notion that AI ethicists can gain anything meaningful from employing the catch-all term ‘responsibility’. Responsibility is disassembled into differentiated parts, ‘accountability’, ‘liability’, and ‘praise and blameworthiness’, which each offer unique insights into the ethical challenges AI poses. This paper argues bundling them together under the heading ‘responsibility’ will not only fail to capture these insights, but will leave our ethical problems unanswered.
Key Insights
Responsibility is defined in ordinary parlance, rather crudely, as the identification of a person who ‘has to’ give reasons for her actions. However, this overly simplified dialogical prototype fails to capture the differentiated practice of actually attributing responsibility. To prove this point, Heinrichs teases out the definition of responsibility into three distinct parts, “In what way (1) is who (2) responsible for what (3)”, which each face challenges in approaching AI ethics dilemmas. Responsibility in its current form, it is argued, cannot account for the complex structure of actions taken with AI support.
Responsible in what way?
This paper argues that when we say an agent is responsible we are actually saying that the agent ought to give justification for their actions. Just as a suspect is called to give an alibi, responsible individuals are called to morally justify their actions. The extent to which one can be worthy of praise, blame, and liability rest upon this justification. Being able to give reasons for our actions is therefore a necessary precondition of being involved in our shared morality.
Who is responsible?
With this definition, who can be responsible for an action? This paper argues machines cannot give reasons for their actions beyond mere causality. For instance, a machine learning model answering the question, ‘why did you do x?’, would at best supply a causal history which led to x. This is because a machine is incapable of moral reasoning and justification. They are, in this way, unable to be held morally accountable. To avoid a repugnant moral responsibility gap, the direction of accountability must shift to another agent to morally justify the action, seemingly a human involved in the AI system’s development or usage. However, this is not as clear a solution as one might hope.
There are many hands involved in the development of an AI system, including the designers, software engineers, data scientists and senior management – all before the system is even deployed. For this reason, a single human cannot reasonably be held morally accountable for the actions of AI. Meanwhile, this paper dispels the possibility of group responsibility, as this would lead to a ‘deviant’ form of accountability. This is because individuals within an AI system’s development are not connected in a moral way, they are connected only by a shared involvement with a machine. This isn’t enough to cluster them together as a single legal person, or other recipient of moral or legal accountability. Even if it were, the group exists as a complex and dynamic structure; people leave, join, and change roles, and so it is not always clear which role each person has played in bringing about the action of an AI system.
Responsible for what?
Seemingly, we are held accountable for the actions we perform. This paper argues machines cannot be held accountable for the actions they bring about, as they do not perform them. This paper defines AI as a complex support system to humans of varying autonomy; sometimes the AI can output independently, but most of the time the human determines the outcome. For this reason, the machine is not a genuine author of its own actions. This paper reinforces the point that machines do not perform actions in themselves as they lack “pro-attitudes” such as beliefs or desires. Machines simply perform the function of altering an input into an output, and so their ‘actions’ are merely the outputs of a predetermined algorithm.
Humans on the other hand are capable of “pro-attitudes” which guide our actions. Yet, Heinrichs argues, AI ethicists have failed to consider in sufficient depth how humans might be accountable for the performance of AI actions. Rather than ascribing a human with the entire action of an AI system, Heinrich argues we should consider how collections of individuals are each accountable in different ways for the outcomes of AI. For example, the data scientist is not accountable for the entire output, but perhaps for the underlying bias which led the system to output one answer instead of another.
Heinrich’s conclusion
Responsibility, Heinrich concludes, does none of the work necessary to solve ethical problems in the development of AI. The foundations of moral responsibility may be reducible to accountability. However, neither the machines nor humans offer a clear subject of moral accountability in the complex ethical problems which arise in AI development.
Moreover, he argues, solving ethical problems involves using differentiated terms individually to solve individual problems, as opposed to catch-all ‘responsibility’ solving everything. This is especially important in AI which requires specialised terms and concentrated examination. AI is itself not a moral agent, Heinrich argues, but a ‘non-agent’ or ‘pseudo-agent’. AI ethics, therefore, requires specialised terms from the start. As Heinrich succinctly concludes, ‘ we would do better so skip the detour and use the more specialized terminology from the outset’.
Between the lines
Heinrich offers a fresh take to the noisy world of AI ethics, in which we are repeatedly fed buzzwords without due challenge and examination. Exploring the limitations of responsibility, he has outlined for readers some of the challenges in AI ethics, and where responsibility fits in this crowded field.
But, he seems pessimistic about the capabilities of future AI, brushing off the notion that AI systems can ever be the author of their own actions. Today, we are still overcoming hurdles in making AI more autonomous across a range of tasks; however, we are overcoming them. Brushing off AI as incapable of being the real, independent source of change in the world may be selling AI short and underestimating the kinds of ethical consequences we face when no human should be held accountable and the real accountability gap emerges.