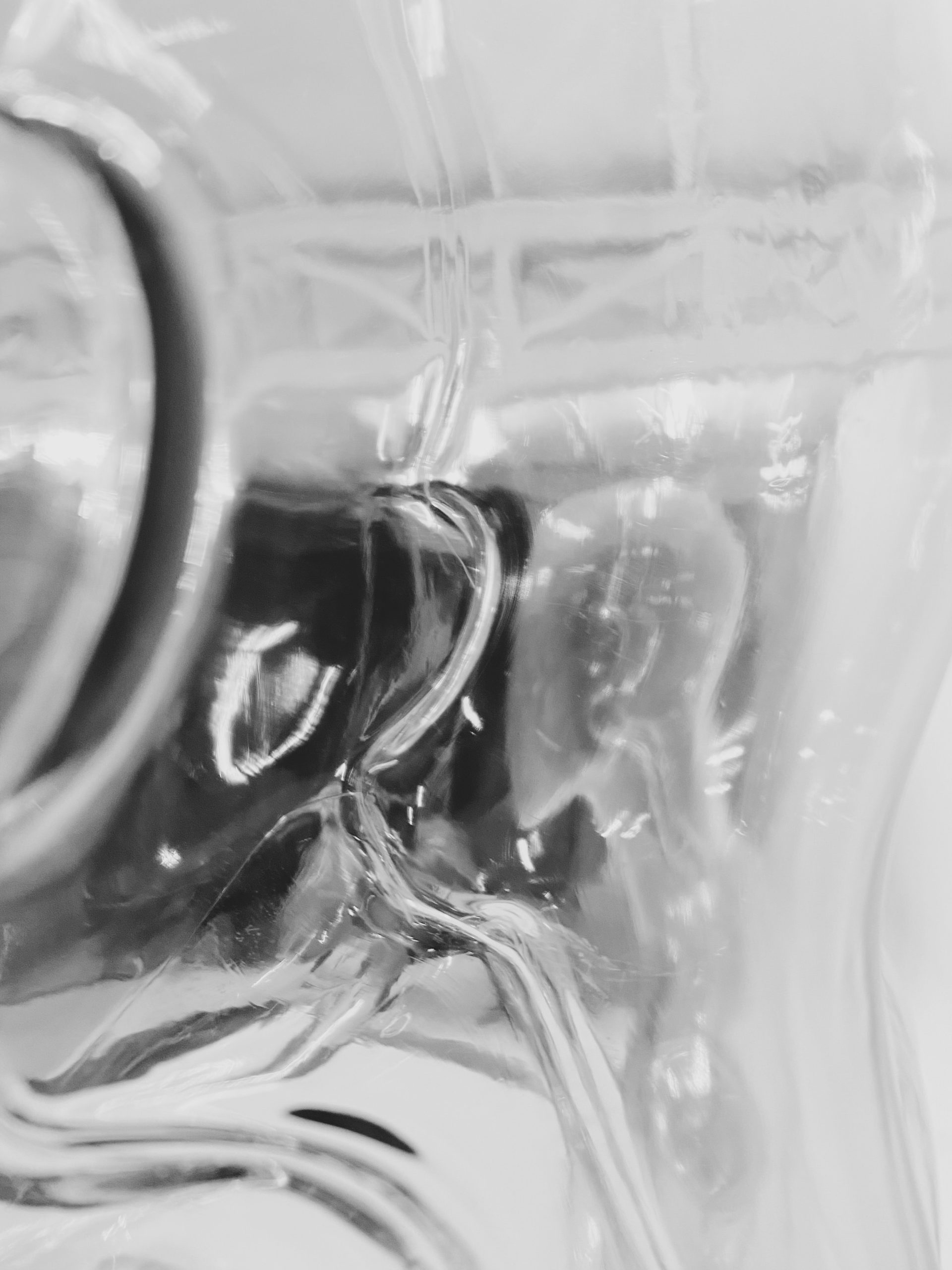
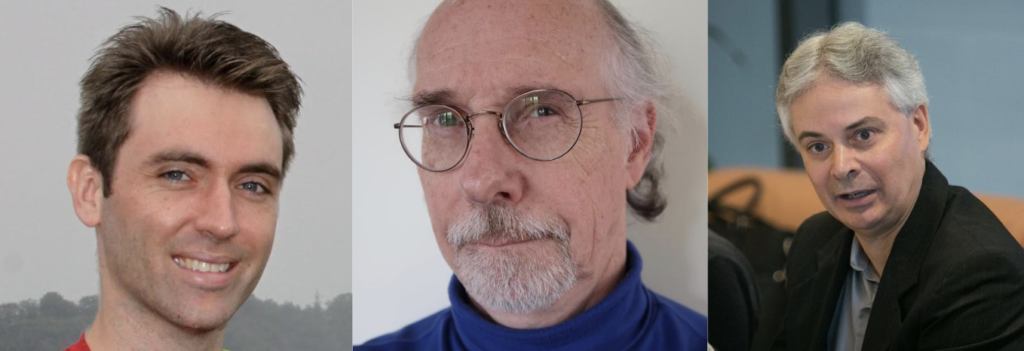
š¬ Research Summary by David Piorkowski, John Richards, Michael Hind. David Piorkowski is a Research Staff Member at IBM Research. His work focuses on the human-factors of AI, most recently in the contexts of AI transparency and trust. John Richards is a Distinguished Research Staff Member at IBM Research. He is currently investigating the formation of sustainable trust in technology. Michael Hind is a Distinguished Research Staff Member at IBM Research, where he leads the AI FactSheets project, focusing on AI transparency, governance, and risk.
[Original paper by David Piorkowski, John Richards, Michael Hind]
Overview: This paper discussed the efficacy of the AI FactSheet methodology, a user-centered technique for identifying, gathering, and presenting AI documentation. We report on a development team that used the methodology in their AI documentation process over a three-month period.Ā We found that the methodology was readily incorporated and resulted in high-quality documentation that met the needs of several different roles.
Introduction
AI model decisions have the potential to cause harm. Consequently, governments and society have demanded greater transparency into how AI models are built and used. In response,
numerous proposals for AI documentation have been suggested. However, given the diversity of documentation consumers, model uses, and model types, a uniform documentation template will not be sufficient. Our recent work [3] evaluates a methodology [2] to address this diversity by suggesting, instead, a uniform process for creating model documentation. We have applied it to the creation of a form of model documentation we call FactSheets [1], and we will describe it in connection with this, but it can be usefully applied to whatever form of documentation is needed.
Our FactSheets methodology centers on three roles: content producers, content consumers, and a FactSheets (FS) team. At a high level, the FS team drives the methodology identifying consumer needs, gathering the relevant information from producers, and curating that information into a FactSheet. The FS team repeats this process, iteratively developing the FactSheet until the consumersā needs are met.
The goal of our recent work is to evaluate the methodology in a real-world context via a case study of an FS team who built and documented multiple AI models over three months. Our research questions were:
RQ1 Is the methodology usable by FS team members not trained in human-centered practices?
RQ2 How well did the resulting FactSheets address the needs of different consumers?Ā
RQ3 What did consumers and producers of FactSheets see as the benefits and costs?
Key Insights
FactSheets Methodology
At the heart of this study is the FactSheets methodology, which defines seven steps to help teams identify documentation needs, build documentation templates and finished FactSheets, and evaluate them in a human-centered way.
The seven steps of the methodology are:
- Know Your FactSheet Consumers. Understanding the information needs of FactSheet consumers is the first and most important task. Working with even one representative informant from each major process in the AI lifecycle will provide useful insights into the overall set of consumer needs.
- Know Your FactSheet Producers. Some facts can be automatically generated by tooling. Some facts can only be produced by a knowledgeable human. Both kinds of facts will be considered during this step.
- Create a FactSheet Template. A FactSheet template will contain what can be thought of as questions or, alternatively, the fields in a form. Each individual FactSheet will contain the answers to these questions. An example template is shown in Table 1.
- Fill In FactSheet Template. This step is where the creator or creators of the FactSheet template attempt to complete it for the first time.
- Have Actual Producers Create a FactSheet. In this step, actual fact producers fill in the template for their part of the AI lifecycle. For example, if there is a question in the template about model purpose, someone who would actually be providing that information would answer the question.
- Evaluate Actual FactSheet with Consumers. In this step an assessment is conducted of the quality and completeness of the actual FactSheet produced in the previous step. If the FactSheet is intended to be used by multiple roles (not uncommon), it should be evaluated separately for each role.
- Devise Other Templates for Other Audiences and Purposes. This step returns to the beginning and is the only defined iteration in the methodology to build FactSheets for other consumers or use cases.
Study design
To answer our research questions, we partnered with an AI organization in the healthcare domain that was piloting the FactSheets methodology for several models to address their AI documentation and transparency needs. Over the course of 3 months, we interviewed a total of 16 participants involved in the creation of 4 different AI models. The participants included a mix of FS team members, producers, and consumers, to get a holistic perspective. Data from the interviews was qualitatively analyzed for common themes and mapped back to our research questions.
Key Findings
RQ1: Participants were able to integrate the FS methodology into their existing development methods. Despite not being formally trained in human-centered methods, FS team members were able to effectively identify documentation needs, evaluate the documentationās usefulness, and improve it based on the feedback they received from consumers.
Table 1: Example template created by one of the FS teams.
RQ2: We asked FactSheet consumers to evaluate the usefulness of the FactSheet along several quality dimensions previously identified as important from the related fields of software documentation and pragmatics. We confirmed that the FactSheet methodology resulted in documentation perceived to be of high quality that met the needs of different FactSheet consumers. Figure 1 shows the judged quality of the resulting FactSheets on several different dimensions.
Figure 1: Consumer responses for evaluating the FactSheet quality. On the left are the quality dimensions we evaluated. The responses on the right side of the dark vertical line represent responses of āslightly agreeā or higher.
RQ3: Documentation in a single, centralized location that contained pointers to more detailed information, and serving as the single source of truth was lauded as the largest improvement over existing practices in which documentation is scattered over multiple tools and locations or often not captured at all. However, it took somewhere between 6 and 24 hours of work to pull together the information into a single FactSheet.
Between the lines
AI development is not a solitary activity. It involves cross-functional teams with different expertise, iteratively building, testing, refining, deploying, and monitoring AI models and the systems that incorporate them. The FactSheets methodology acknowledges and is built on this truth. Our evaluation of the methodology suggests that it worked as intended, with teams able to use the methodology with few local adaptations to create AI documentation that addressed the specific needs of their consumers despite substantial differences between models and between use cases.
We believe that documentation needs to be designed with a clear understanding of its various consumers. Our study suggests that a multi-tiered structure with a near universal top-level view, supplemented by more detailed and specialized information in further tiers, allows consumers the flexibility to find the specific information they need at the level of detail they require.
Finally, we anticipate that new tooling will be required to fully support the methodology. Automatic collection of model information through the use of suitably instrumented tools, and help for those who need to describe key facts about model development for information that cannot be automatically captured, will be needed.
Notes
[1] FactSheets: Increasing Trust in AI Services through Supplier’s Declarations of Conformity, Arnold, M., Bellamy, R.K.E., Hind, M., Houde, S., Mehta, S., MojsiloviÄ, A. ,Nair, R., Natesan Ramamurthy, K., Reimer, D., Olteanu, A., Piorkowski, D., Tsay, J. and Varshney, K. R., IBM Journal of Research and Development, 63(4/5), July-Sept, 2019.
[2] A Methodology for Creating AI FactSheets, Richards, J., Piorkowski, D., Hind, M., Houde, S., MojsiloviÄ, A., Bulletin of the Technical Committee on Data Engineering, 44(4), Dec, 2021.
[3] Evaluating a Methodology for Increasing AI Transparency: A Case Study, Piorkowski, D., Richards, J. and Hind, M., arXiv, Jan, 2022