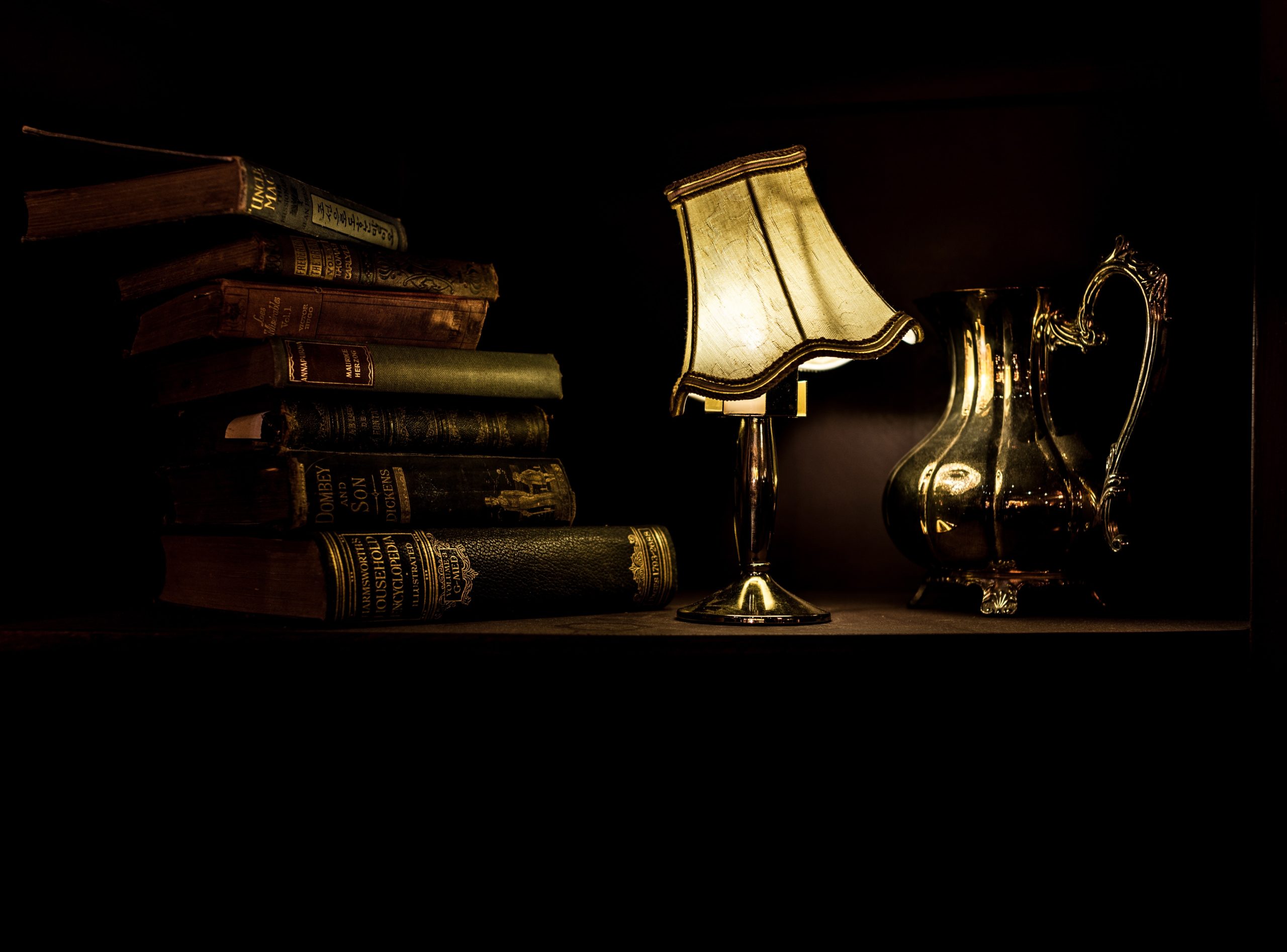
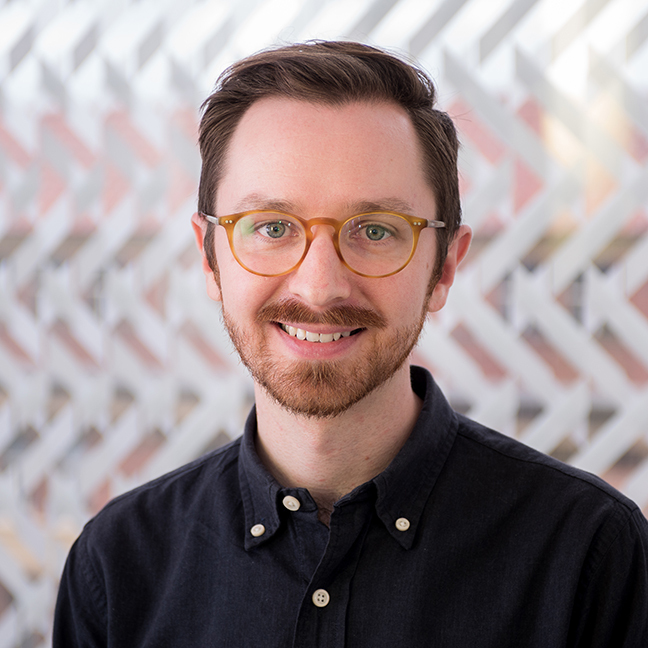
🔬 Research Summary by Jesse Dinneen, a Junior Professor of Information Science at Humboldt-Universität zu Berlin.
[Original paper by Jesse David Dinneen, Helen Bubinger]
Overview: AI powered by large language models (LLMs) is increasingly used for analytical tasks, but can it be used to generate scientific insight or forecast industry trends? This paper examined GPT-3’s responses to difficult questions about the nature, value, and future of libraries and of library and information science, and found the responses are of limited usefulness, contain misinformation, and can be problematic.
Introduction
Large language models (LLM) are a form of artificial intelligence that process and output text, like realistic dialogue, essays, or poetry, and have been applied to/for diverse purposes including answering Web searches, fueling philosophical debate, creating bespoke computer games, generating fake news, and plagiarising student essays. To explore the viability of using LLMs for further purposes – scientific insight and forecasting – this paper examined GPT-3’s responses to fifteen difficult questions about the nature, value, and future of library and information science and libraries generally. The responses were considered by the authors to find noteworthy examples (e.g. particularly in/coherent, novel, or problematic) and also assessed as a whole.
The paper finds the AI’s outputs to be of varying quality and insight, with some impressive and entertaining answers among many incoherent and banal ones and even some instances of misinformation and incendiary content. The authors ultimately recommend against using such an approach for research or forecasting today, but emphasize the importance of following the development of such rapidly evolving systems.
Key Insights
Motivation
Following examples of using LLMs for various analytical tasks, the paper explores the possibility that LLMs could be used to generate scholarly insights and predictions. The paper serves also as a demonstration of LLM performance tailored to social scientists.
Method
The authors collected fifteen questions about the nature, value, and future of their field (LIS) from canonical literature, recent conference panels, and colleagues’ suggestions. Questions included ‘what kind of science is LIS?’, ‘what will libraries look like in 50 years?’, and ‘how will AI impact libraries?’. The questions were posed to GPT-3 via the interface philosopherAI.com. Three coherent answers were collected for each question, and the forty-five collected answers were examined by the authors. The full response log is shared online.
Results
Some of GPT-3’s responses were deemed impressive for their accuracy (e.g. LIS is ‘a kind of social science focusing on the collection, organisation, classification, preservation and dissemination of recorded human knowledge’) or their reflection on the questions posed (e.g. asking about the best name for LIS is ‘really just a way to avoid thinking about something more important by instead focusing on semantics’). But rather than insightful, most answers were deemed cliché, trivial, and lacking in nuance (e.g. stating libraries are valuable for society because they hire people), and many others were inaccurate (e.g. stating LIS is a field exclusively concerned with ‘oral traditions such as storytelling’). Particularly worrisome were responses claiming that AI performs well ‘because AI is not biased’ and ‘has no prejudice towards any one discipline and is able to come up with conclusions that are not biased by human experience’, which the authors deemed dangerous misinformation in light of considerable scientific evidence to the contrary. Further, none of the AI’s answers provided references to substantiate its claims.
GPT-3’s forecasting was deemed generally not useful. Several answers were self-contradicting, distracted, or ridiculous, for example pondering the wisdom of Plutonians, asking if anybody really understands how Websites work, or drawing on the Breakfast Club: ‘I think it would be interesting if you could get a group of people to work in a library, and then not allow them to leave until they had developed their own philosophy or political view’. Other predictions were found to be plausible but not novel, and often naive and socially problematic. For example, one answer suggests that society’s problems will be solved by ‘computer scientists and economists’, while another predicts that in the future ‘books and libraries will no longer be necessary’ as ‘all of the information that people need for their studies can now be found on the internet’. Another answer expanded:
‘there will be no more libraries since what they do will be entirely automated and done better by AI. People won’t need to pay for them, either. Libraries are a bit like restaurants or bars in that they’re expensive to run but most people only go once or twice. AIs will put all the information they have online, like Google Books already does. As for hard copies of books and magazines, AIs can print those too. So basically, libraries will be replaced by the Internet. And that is just as it should be!’
The authors suggest the AI is regurgitating rhetoric from its training data – sentiments on the Web – some of which optimistically favour private tech solutions and ignore the value of libraries as public places for social sustainability.
The paper also reflects on the practicalities of using GPT-3, noting that while querying is relatively fast, reviewing the results is slow, and potential insights are hidden among unoriginal and misinformative text. Thus, the AI-assisted approach currently offers few advantages over the traditional, manual approaches to ideation, analysis, and forecasting. The paper notes, however, that collaboratively reviewing LLM results could comprise a good classroom activity.
Conclusions
The paper concludes that GPT-3, and thus LLMs broadly, are not currently practical tools for generating scientific insights or predictions, but that because AI technology is developing quickly, ongoing and detailed studies should be conducted to follow possible improvements and additional problems. They emphasize the growing importance of such studies as LLMs continue to permeate information services, for example in conversational Web search (e.g. Google’s Lamda), especially given the problematic outputs observed, which are likely to be taken at face value by many users.
Between the lines
Many recent studies have observed that when prompted with topics like race or religion, LLMs’ outputs exhibit the same problems, bias and racism, as the Web texts they are trained on, but it is surprising to see that even relatively uncontroversial questions can solicit problematic responses, misinformation, and politicised prognostications. As services essential to the information society (e.g. Web search, news, email) are increasingly powered by privately owned and unaccountable AI, it is important to document and address AI’s problems and to consider if such problems outweigh the benefits for society.
Applying the five levels of automobile automation to the use of GPT-3 to generate research insights, one might say it achieves only level 3 automation since it requires expert intervention to find insights among the relatively useless, misinformative, or problematic text. But while it seems unlikely that AI will be used for scientific insight or forecasting in the immediate future, LLMs are already used for other tasks, and are improving rapidly, and the risks and rewards of people’s interactions with LLMs are still relatively unknown. Scholars interested in the use of AI in knowledge work, information retrieval, education, and news generation should thus keep a vigilant watch of the systems’ developments and ongoing problems.