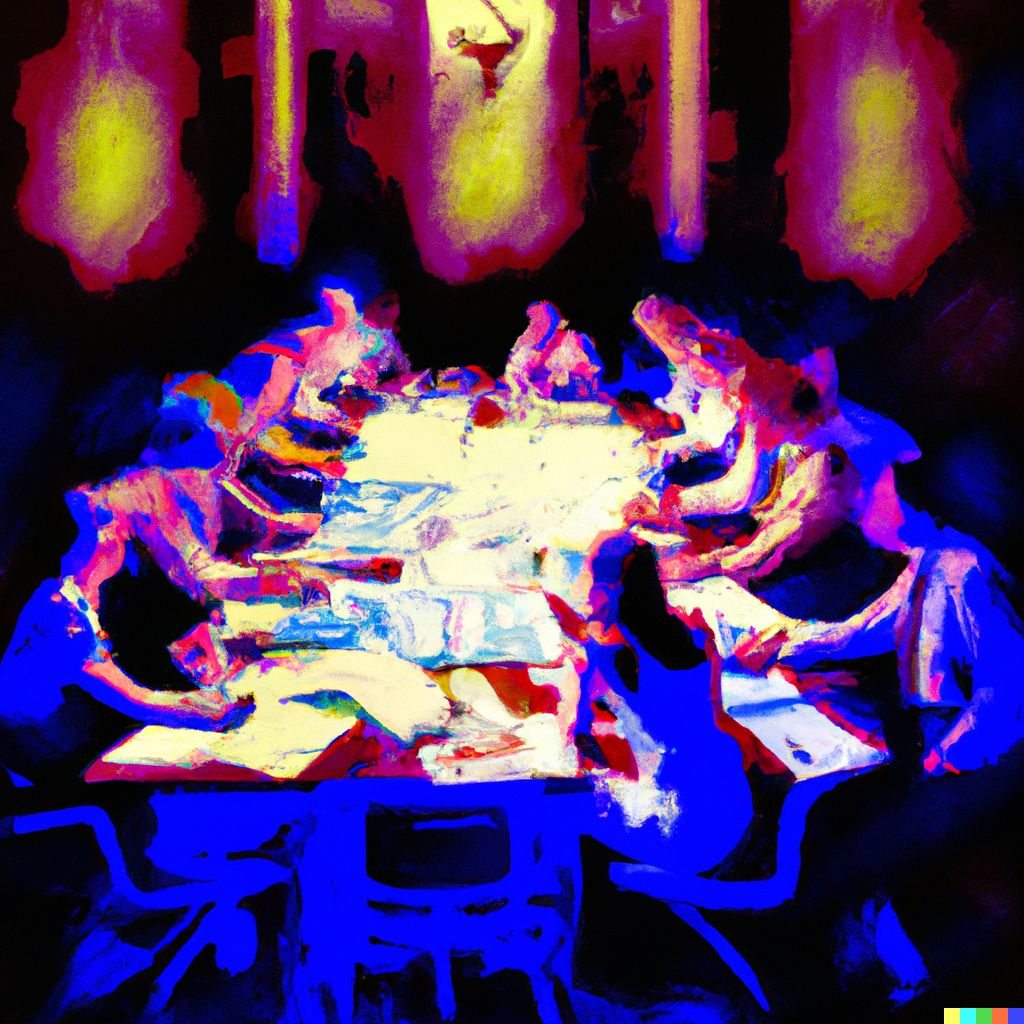
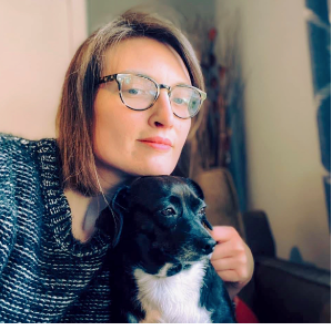
🔬 Research Summary by Sara Kingsley, a researcher at Carnegie Mellon University, and an expert in A.I. system risk assessments, having built A.I. auditing tools, as well as red teamed multiple generative A.I. systems for different technology companies.
[Original paper by Rena Li, Sara Kingsley, Chelsea Fan, Proteeti Sinha, Nora Wai, Jaimie Lee, Hong Shen, Motahhare Eslami, and Jason Hong.]
Overview: There is an emerging phenomenon of people organically coming together to audit AI systems for bias. This paper showcases four cases of user-driven A.I. audits to offer critical lessons about what type of labor might get authorities to address the risks of different A.I. systems. Equally, the paper calls on stakeholders to examine how users wish authorities to respond to their reports of societal harm.
Introduction
The White House, technology companies, and researchers have called for auditors to assess the potential and unknown risks of A.I. systems, but should we necessarily only rely on experts and scientific methods to unearth the risks of A.I.? Everyday users have a wealth of knowledge, also from their lived experience and interactions with automated decision and content generation systems, including those currently making critical decisions about our lives. However, not much is known about how everyday users audit A.I. systems — what tactics do they use? What is their division of labor? Based on our investigation of participation in and the labor of everyday user audits, we present our results on how users document A.I. biases. Our findings have implications for developing tools, systems, and frameworks (including for A.I. governance) to support people in addressing A.I. biases.
Key Insights
The Four User-Driven Auditing Cases
In our paper, “Participation and Division of Labor in User-Driven Algorithm Audits,” presented at the Association for Computing Machinery Conference on Human Factors in Computing (CHI), we report common roles and the patterns of engagement users displayed in four different audits of A.I. systems. The first case, the Twitter Image Cropping case, involved users auditing a feature on Twitter that automatically resized and centered images that users uploaded to the social media platform. Twitter users claimed the cropping algorithm was racist after they noticed it tended to center white people, removing Black people from view.
The second case, ImageNet Roulette, involved users testing out an algorithm that automatically applied labels (e.g., secretary, software developer, etc.) to images they uploaded to a website. Notably, the ImageNet Roulette website had been designed as an art project to raise peoples’ awareness of the biases that the underlying dataset (and the algorithms built on it) could produce.
The third case, the Apple Card case, involved Twitter users sharing the outcome of their applications to receive financial credit from Goldman Sachs (e.g., the Apple credit card). After a tweet by a famous person called attention to the matter, users questioned whether Goldman Sachs approved women for less credit than men; their outcries on Twitter eventually led the New York state government to audit Goldman Sachs.
Finally, the fourth case involved Portrait AI, an app enabling users to upload their ‘selfie’ photos, which the app would then automatically transform into 19th-century portraits. On Twitter, a few users noted they felt the Portrait AI app changed the race of people, erasing the representation of users from marginalized demographics. Unlike the other three user audits, though, most users sharing their Portrait AI-generated self-portraits did not claim the app was biased, possibly because there was a lack of bias awareness since neither news media nor celebrities on Twitter had reported the algorithm was possibly biased.
These four user-driven auditing cases unearthed critical and unexplored lessons about how everyday people perceive and assess the societal risks and potential harms of A.I. systems. Particularly, these audits included generative A.I. image systems and systems designed for content classification and resizing, e.g., automated tasks that might heavily influence the output of other A.I. systems.
Many Blasting One Tweet About A.I. Risks Might Do More Than A Comprehensive Statistical Audit
Unlike comprehensive statistical audits, where typically a few experts invest a lot of labor and resources toward analyzing datasets over a long period of time, in three user auditing cases (i.e., Twitter Cropping, ImageNet Roulette, Apple Card), we found user participation in various auditing activities typically surged immediately after initial reports of A.I. system risks circulated on Twitter.
We found user participation also defied common notions about Slacktivism (e.g., the idea being people blast off a few tweets about a social issue, but this does not lead authorities to address the problem). In three auditing cases, users typically contributed only a single tweet. However, in these cases, the algorithm operator or a government authority responded by auditing the algorithm in question. Thus, users are generating collective awareness and consensus that some authority should address the biases of an A.I. system by amplifying and spreading the word among their followers. By doing so, many users sharing only one tweet can lead to an intervention.
Discovering Ways to Help Crowds of People Conduct User-Driven Audits
By engaging in conversation threads on Twitter, our paper shows users built on their own and others’ hypotheses, evidence, and techniques for auditing A.I. biases. In this way, we believe crowds of people sharing information about A.I. behaviors and auditing activities offer an important way for communities to discover A.I. biases.
A long line of prior research even suggests the collective intelligence of crowds of people sharing and making sense of information can perform as well as experts. Our paper calls attention to additional ways of investigating A.I. biases by supporting crowds of people conducting user-driven audits. Particularly, our paper suggests crowds of people auditing A.I. biases could benefit from tools specifically designed to support user-driven audits.
For one, in the user-driven auditing cases we investigated, each case had several hundred unique conversations, and this highlighted a need to develop tools to help users share information in a centralized space, as this would enable users to more easily access and build on the works of others. To be clear, we found many distinct conversation threads about the same A.I. bias case, indicating that access to all the information shared by users was fragmented and decentralized. As such, our paper suggests the fragmented nature of information sharing might have made it difficult for users to understand the complete history and evidence generated about a particular A.I. bias case. Similarly, our paper discusses how crowds of people conducting user-driven audits might benefit from tools enabling them to compare different sets of evidence.
Between the lines
Along these lines, we found access to algorithmic systems is important for enabling and supporting user-driven auditing. For example, the Twitter Image Cropping, ImageNet Roulette, and Portrait A.I. algorithms were easy for users to test; they simply uploaded their images to the platforms and then shared the algorithm response with other users on Twitter. In contrast, the algorithm in the Apple Card case was not accessible, users were not interacting with it directly, but instead, if married, they were submitting their information in pairs through a web form and then observing the credit limit each person was approved to receive. A breadth of prior research has documented that A.I. biases (e.g., gender bias in credit) can take different forms for different demographics (e.g., unmarried, married women and non-binary people). Providing access to software for users to test for A.I. biases directly is critical for understanding A.I. biases comprehensively.
Ultimately, from these and additional findings, our paper shows the benefits of studying how crowds of people already audit for A.I. biases: users can help us understand how to design better tools and ways to support A.I. assessments.