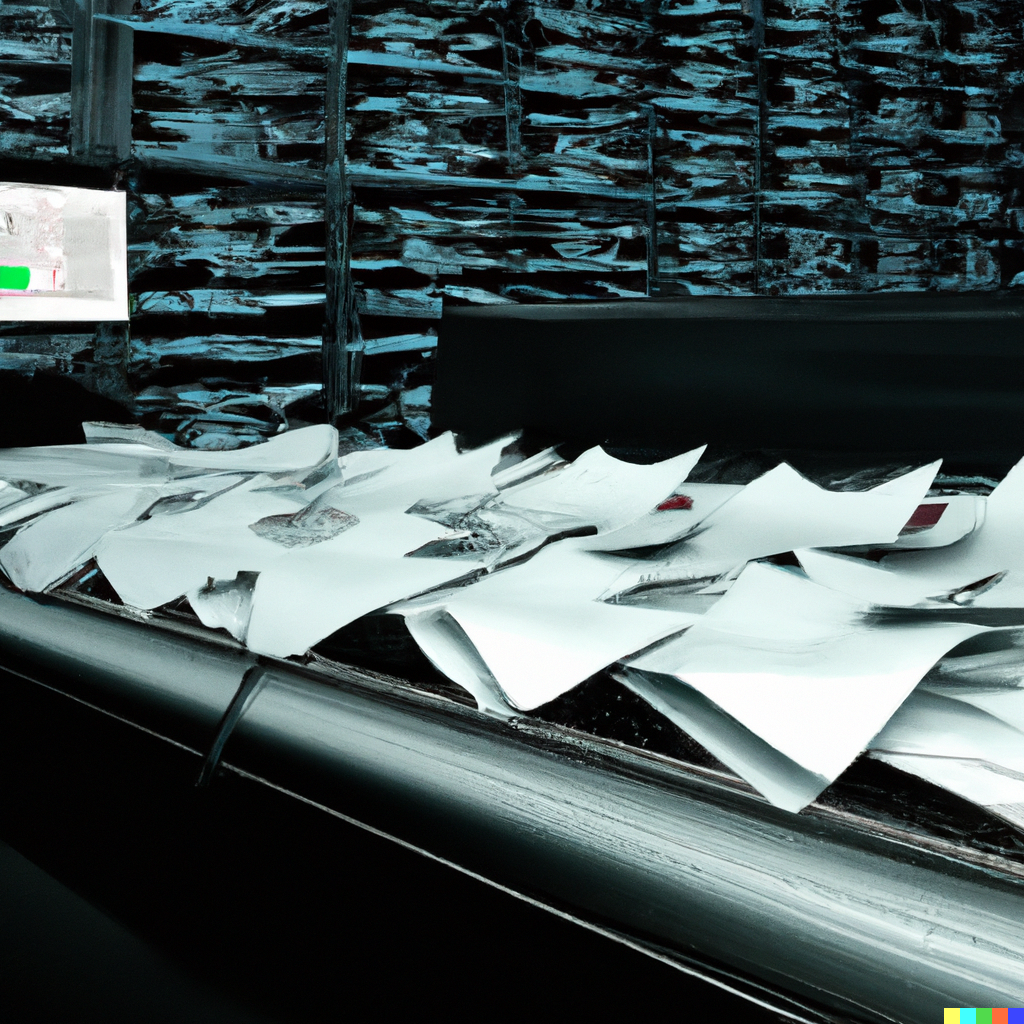
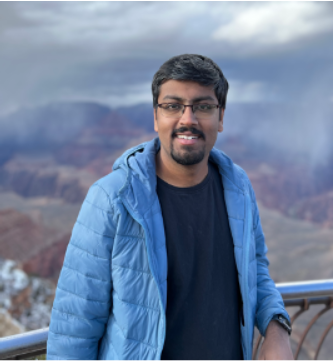
🔬 Research Summary by Abhishek Roy, a post-doc at Halıcıoğlu Data Science Institute, UC San Diego
[Original paper by Abhishek Roy and Prasant Mohapatra]
Overview:
Designing fair Machine Learning (ML) algorithms has garnered significant attention in the recent past to make standard ML algorithms robust against prejudiced decision-making in sensitive applications like judiciary systems, medical applications, and college admissions. Training a fair ML algorithm requires solving an underlying constrained optimization problem. Stochastic optimization algorithms like Stochastic Gradient Descent (SGD) are routinely used to train such a model. The randomness of the training data distribution manifests through the data stream that SGD is trained on and renders the learned model and its fairness properties random. Even if the algorithm is fair on average, a large variation in the algorithm outputs can have far-reaching consequences in the above applications. So, besides the average fairness level, it is important for a practitioner to know the uncertainty in the fairness level of a fair algorithm. This paper takes a step toward quantifying this uncertainty.
Introduction
We start with an example of a college admission system that ideally should not discriminate between two students based on their gender. In other words, the chances of admission for a male (Bob) student and a female (Alice) student, who are identical otherwise, should not be too different. Say ~5% unfairness (difference between the chances of Bob and Alice) is acceptable on average. Say the college has two classification algorithms, A and B, which are trained with incoming student data each year. Say both A and B have 5% on average unfairness, and
- A is 4% unfair half the time and 6% unfair the other half of the time,
- B is 4% unfair 95% of the time but 24% unfair 5% of the time.
Then Algorithm A is preferable because even though B is fairer than A most of the time, 5% of the time, B is too unfair to be acceptable in a life-defining application like a college admission system. In other words, A and B differ considerably with respect to the range of variability of the unfairness. A practitioner must know this uncertainty for the reliable use of these algorithms. This is even more crucial in real-time decision-making problems where the whole data is unavailable at once and arrives in a streaming manner. For example, the classifier used by the college admission systems gets updated with each incoming student. Formally, training a real-time (online) fairness-aware classification algorithm turns out to be an online-constrained stochastic optimization problem. In summary, the paper seeks to answer the following question:
How to quantify the uncertainty present in the fairness of a fairness-aware classifier in the online regime, i.e., with access to a single stream of data, when trained with a stochastic optimization algorithm?
Key Insights
Setting
Even though the paper addresses a more general setting, for better exposition, let us consider the above example of the college admission system. Say the college uses SGD with streaming data to train a logistic regression model to classify the applicants into 2 groups, admissible (1) and non-admissible (0). The goal is to ensure that the classifier does not suffer from Disparate Impact – the probability of the classifier predicting 1 should be the same for Bob and Alice.
Characterizing the uncertainty
In this setting, the view of fairness-aware classification through the lens of optimization reveals that the estimated parameter of the logistic regression model asymptotically follows a normal distribution whose covariance depends on the training data distribution and the solution of the underlying optimization problem. This insight shines a light on the uncertainty of fairness-aware classification. It paves the way to construct a confidence interval for the model’s fairness when this trained model is used to classify previously unseen (test) data.
Quantifying the uncertainty
To construct a confidence interval for the test fairness in real time, one needs to estimate the asymptotic distribution of the model parameter. To practically quantify this randomness, the paper introduces an easily parallelizable online multiplier-bootstrap algorithm. Instead of going into the technical details of the algorithm, let us try to understand it from a high-level. The method works as follows: for each incoming datapoint, the algorithm creates a set of independently randomly perturbed gradients to create a set of perturbed SGD iterates which are essentially random estimates of the model parameter. Using these estimates, a random sample of test-fairness can be computed using a small dataset that is not used for training (referred to as held-out dataset in inference literature). The paper theoretically shows that at any given time-point, the confidence interval constructed using these samples of test-fairness values, is a consistent estimator of the true confidence interval, that is, the uncertainty in the test-fairness can be consistently captured in real-time by this confidence interval.
Between the lines
The paper just takes the first step towards quantifying the randomness in the test-unfairness of a binary classifier, explicitly characterizing the effect of the optimization algorithms. Equipped with these results and method, a practitioner can deploy a fairness-aware classifier trained with a stochastic optimization algorithm with more reliability. An interesting by-product of the proposed method is that, besides test-fairness, one can construct confidence interval for the model parameter as well using the random estimates of the model parameter generated at each time-point by the bootstrap algorithm. This could shed light on the features which are significant for fairness-aware classification leading to better model interpretability.
For wider applicability of the methods, it will be interesting to explore similar results in the multi-class classification setting. The most important extension of this work would be to establish similar results for fairness-aware Deep Neural Network classifiers.