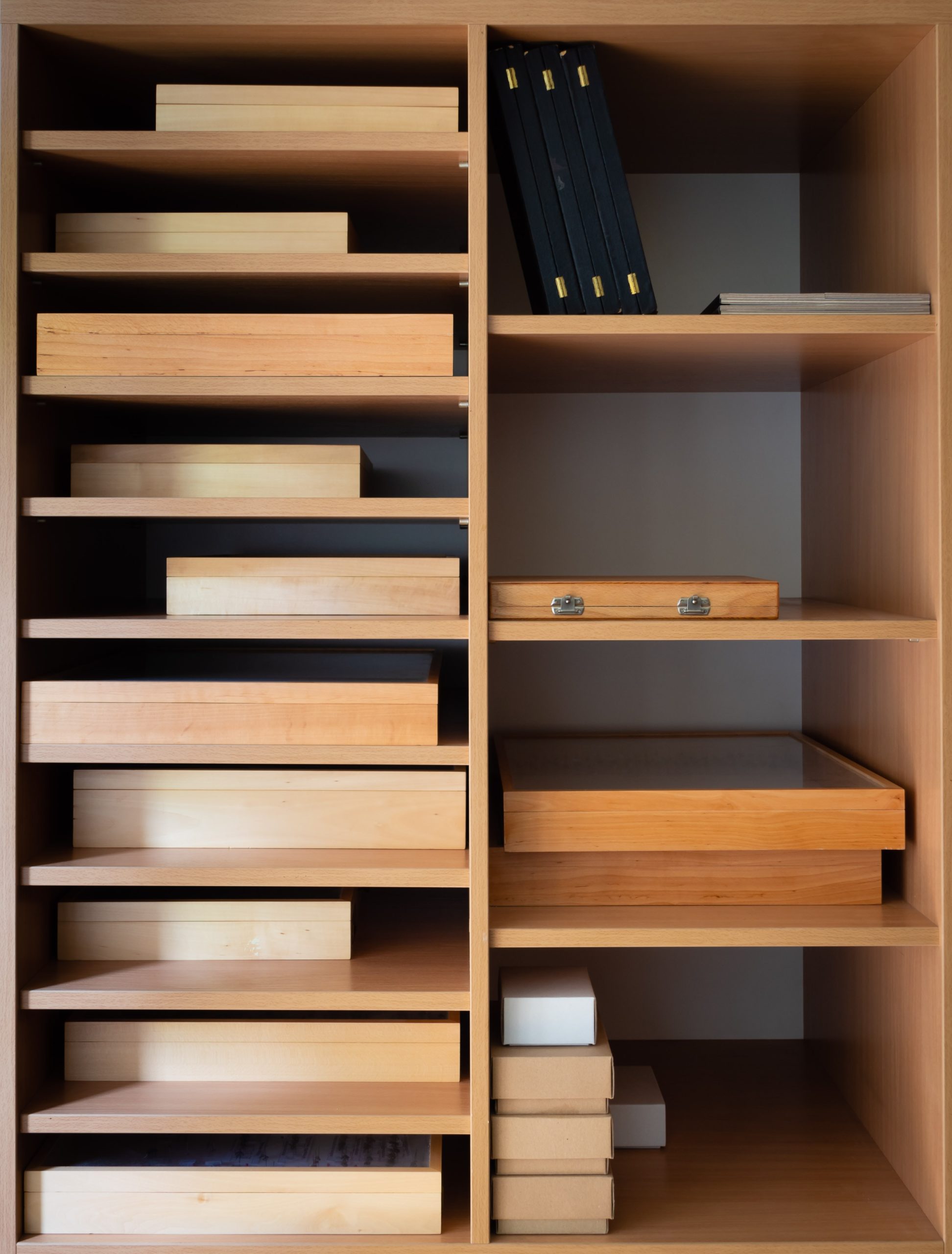
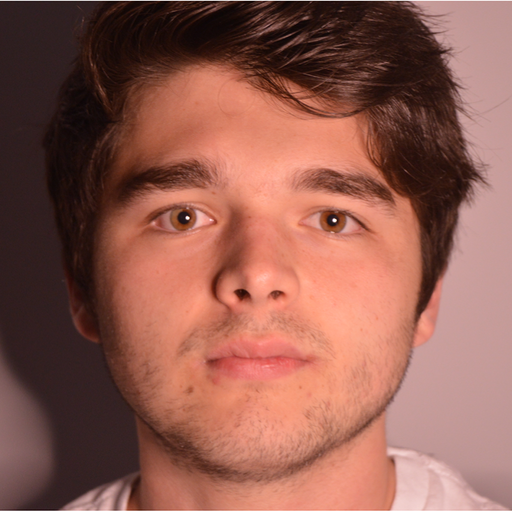
đŹ Research summary by Connor Wright, our Partnerships Manager.
[Original paper by Gonzalo GĂŠnova, ValentĂn Moreno Pelayo and M. Rosario GonzĂĄlez MartĂn]
Overview: While the Turing test significantly influenced machine intelligence, it didnât harbour much ethical consideration. With this in mind, we must be careful not to treat ethics as a neatly packaged set of rules we can input into a machine.
Introduction
The Turing test was hugely influential on considerations about machine intelligence and the subsequent emergence of âprogrammed ethicsâ (p. 75). Yet, given the lack of emphasis on ethical issues within the experiment, âlearned ethicsâ (p. 75) and considerations on explainability and bias also arose. Here, experiments such as MITâs Moral Machine emerged. Yet, while this allowed for public engagement in moral issues with AI, ethics must not consist in simply replicating majority behaviour. Bearing this in mind will help us decipher between what is merely a personal preference and what is societal value. But first, let us consider some initial thoughts on machine intelligence.
Key Insights
Initial considerations on machine intelligence
Initially, the Turing test aimed to demonstrate machine intelligence through the solving of âclosedâ problems, such as chess and imitating a human. In other words, machine intelligence was measured on how it could find the known solution to a problem. While there was not much consideration of the ethical implications of this experiment, Turing of course cared about ethics. Nevertheless, with inspiration drawn from the Turing test, âprogrammedâ and âlearnedâ ethics (p. 75) emerged.
âProgrammedâ vs âlearnedâ ethics
Programmed ethics is all about breaking down the solution to a problem into sequential parts to be followed. Concerning the Turing test, the end goal for the machine to deceive a human was broken down into steps such as âgreeting the humanâ, or âasking questionsâ, amongst others. However, this approach requires the problem to have a clear desired outcome or solution in the first place, which is certainly not the case with complex ethical issues.
Consequently, the practice of learned ethics arose. Treating ethics in a boxed-up, rigid and sequential fashion did not appropriately account for the nature of ethical problems as constantly evolving and their outcome largely unknown. Said development tied in nicely with the developments made in the AI space seen in deep and machine learnings.
MITâs Moral Machine
As a result of these developments, moral considerations on autonomous technologies have become more frequent. For example, MITâs Moral Machine dilemma encourages users to decide for an autonomous car within the experiment between the lesser of two evils. Here, the user can choose between two options for the vehicle, such as running over a man or a woman. The user then relies on their moral faculties to make what they think is the most appropriate decision. These responses are recorded and can then be viewed by those in charge of the experiment.
Experts try to learn from the general responses made by the population that underwent the experiment. However, should they programme a machineâs ethics accordingly, this risks a tyranny of the majority scenario where whatever the majority says is right. What is the majority’s opinion is not always the same as societyâs opinion. For example, we know that bias within AI is terrible, even if the effects of a biased algorithm only affect a minority of the population.
For this reason, being aware of the prominence of explainability and bias within AI ethics is starting to become a best practice. Should we not be able to explain the decision of an AI algorithm within a highly divisive ethical situation, we wonât be able to mitigate any adverse outcomes appropriately. The power of explainability also minimises the potential of bias, such as analysing whether the dataset is genuinely representative.
In the spirit of this previous point, the Moral Machine was left open to a relatively diverse audience. Answers were collected in 10-different languages and in 233 countries and territories (p. 77). Yet, drawing on such a variety of responses perhaps simply reflects cultural practices rather than ethical maxims. On the one hand, âethicsâ is used in a normative sense, describing what is right and wrong. On the other hand, âmoralâ takes on a prescriptive sense, detailing social customs. Hence, MITâs Moral Machine certainly helps to reflect cultural traditions. However, to become normative laws, considerations of what these cultural practices mean in terms of how we act must be considered. This will help us decipher between what is simply a personal preference and what should be a societal value.
Between the lines
In my opinion, the phrase âprogrammed ethicsâ (p. 75) almost sounds like an oxymoron. It presents ethics as a nicely packaged set of rules that we know will work at all times, which we can slot into an AI. Given how this is not the case, machines still have to rely heavily on human input for guidance. For example, despite talk about artificial general intelligence, such technology is still being modeled on human activity. To teach an algorithm how to recognise faces, we ask humans to do it first. Hence, while the integration between AI and ethics rightly deserves attention, the human role in this process must not be underestimated.