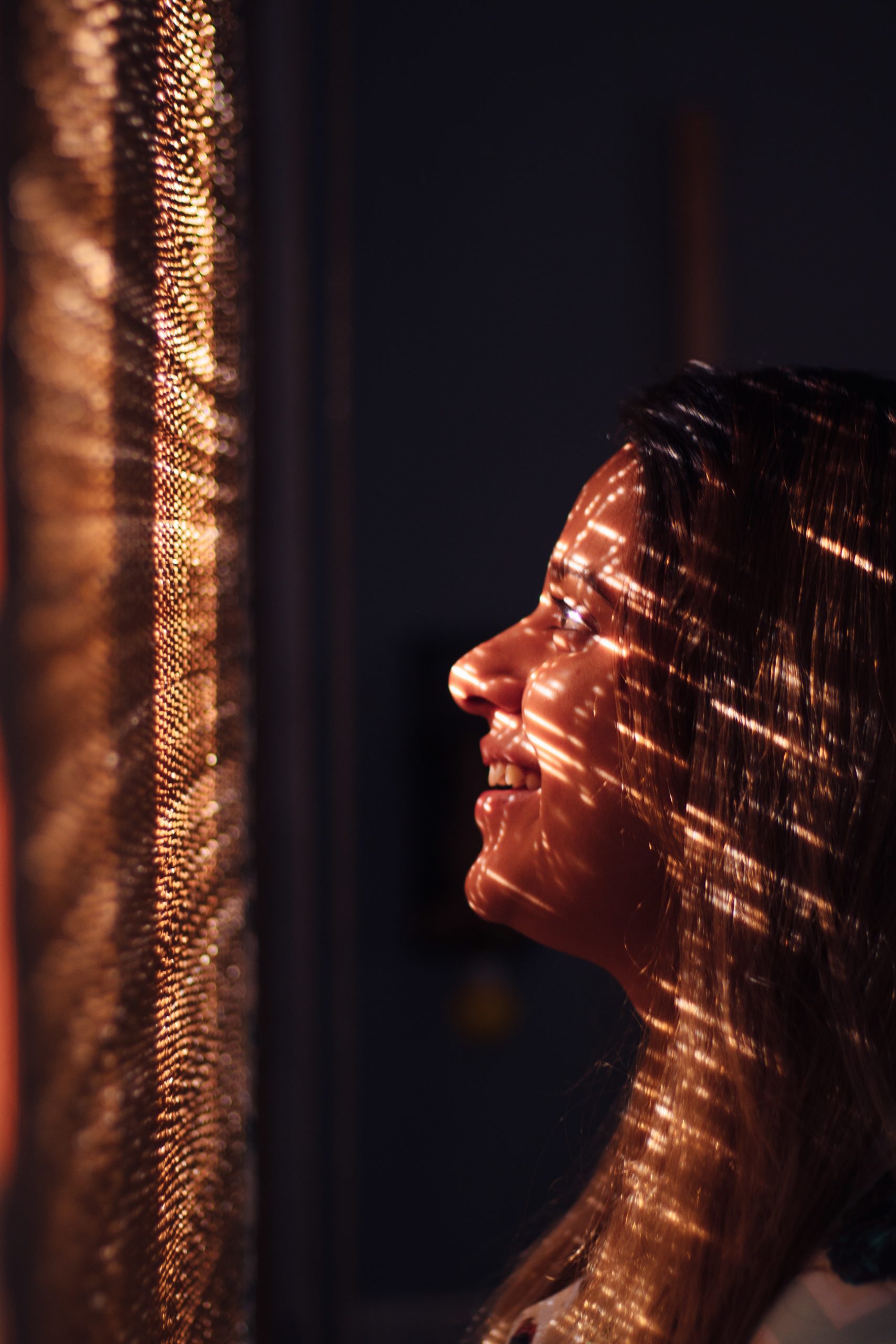
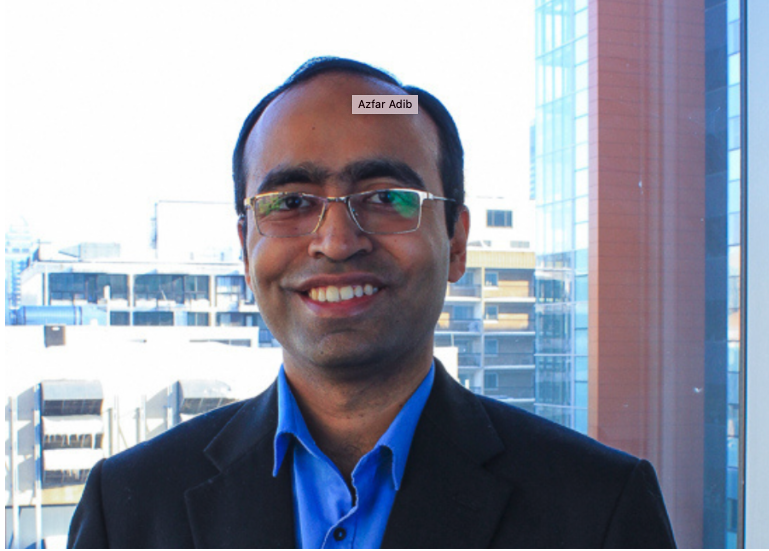
🔬 Original article by Azfar Adib, who is currently pursuing his PhD in Electrical and Computer Engineering in Concordia University in Montreal. He is a Senior Member in the Institute of Electrical and Electronic Engineers (IEEE).
A recent announcement by Meta about terminating the face recognition system in Facebook sparked worldwide attention. It comes as a sort of new reality for many Facebook users, who have been habituated for years to the automatic people recognition feature in Facebook photos and videos.
Since the arrival of mankind on earth, facial outlook has remained as the most common identifier for humans. Facial recognition is a dominating technology being used in numerous applications. Its benefits have been amazing. At the same time, it has been one of the most debated topics, particularly from ethical perspectives.
Drawbacks of facial recognitions often get mentioned as examples of misusing artificial intelligence in general. Such examples are numerous, like- violating basic privacy of people, failure to identify racially marginalized people, or being used in some countries for malicious detection of political opponents. Facial Recognition Technology has even been mentioned as “ one of the biggest threats to our privacy”. In the service-closing statement, Meta (Facebook) also mentioned societal concerns and lack of clear regulations regarding this technology. However, they did mention about the boundless prospects of facial recognition for other applications.
Along with its basic purpose of identity verification, facial analysis remains as a powerful tool for various innovative solutions. Let us consider an example in the medical domain – detecting pain when patients cannot express it. Particularly, infants may not always cry in pain, and untreated pain in newborns can lead to further neurological and behavioral issues. To address this challenge, a group of researchers in University of South Florida have developed a facial recognition software termed as “Neonatal Convolutional Neural Network” (N-CNN), It contains an algorithm for analyzing the facial expression of newborns with postoperative pain. It also analyzes visual -vocal cues and vital signs to sense and predict pain.
This scheme aims to ensure that it will not miss a signal of pain regardless of factors like infant’s position or sedation. Facial analysis remains as the key focus, as mentioned by one of the researchers there- “Our experiments in procedural pain showed that facial expression is the most important indicator of pain in most cases.” The group is now working on monitoring and predicting pain in infants after surgery.
Such technology can ensure better treatment by automatically alerting caregivers about pain in infants. It is worth mentioning that other factors, such as culture and gender, may also affect pain assessments by humans. So, this facial recognition scheme also helps to overcome human observers’ bias. That is an interesting aspect, demonstrating that facial analysis can also assist to counter bias.
Another good example of using facial recognition for clinical purposes is a smartphone App called Face2Gene, developed by a Boston based digital health company. This App uses neural networks to classify distinctive facial features to diagnose congenital and neurodevelopmental disorders, which are quite difficult to identify through plain eye. Since its launch in 2018-19, this App has been significantly assisting clinicians, in fact outperforming them in terms of fast and accurate diagnosis.
These are some inspiring examples of using facial recognition for life-saving purposes. It is worth mentioning that adequate diversity in training data is utmost crucial to ensure bias-free performance of these applications also. In general, biasedness in AI algorithms (particularly in sensitive applications like facial recognition) can be successfully overcome only by training these with sufficiently diverse data. That’s actually much easier said than done. Various realistic challenges exist in this regard.
For instance, most of the publicly available datasets used by researchers contain data from only developed parts of the world. A significant portion of the global population are still not represented there. The reasons are obvious- lack of contextual need, proper procedure and supportive regulation to collect, store and share data in many countries. This needs to be gradually overcome through effective collaboration, trust and technical support among concerned ends.
Regulatory challenges exist in developed regions as well. For example: “Illinois Biometric Information Privacy Act” in the state of Illinois, which is the oldest biometric regulation in the United States, contains following clauses which are not quite favorable for health-based applications- “Biometric identifiers do not include information captured from a patient in a health care setting or information collected, used, or stored for health care treatment…”.
This scenario is obviously improving. Last year the U.S. Food and Drug Administration released their first Artificial Intelligence/Machine Learning (AI/ML)-Based Software as a Medical Device (SaMD) Action Plan. This is considered as a significant regulatory step to facilitate AI in the health industry.
Despite numerous drawbacks (most notably the issue of bias), technologies like facial recognition will continue expanding across our lives, and its improvisation will continue utilizing cutting-edge algorithms and advanced datasets. In certain applications, it can act as a useful tool against human bias as well. Moving ahead, it will be interesting to observe how much facial recognition technologies can evolve from a “source of bias” to a “tool against bias”.
References
1. https://about.fb.com/news/2021/11/update-on-use-of-face-recognition/
3. https://www.embs.org/pulse/articles/detecting-faces-saving-lives/
4. https://www.nature.com/articles/d41586-019-00027-x
5. https://www.ilga.gov/legislation/ilcs/ilcs3.asp?ActID=3004&ChapterID=57