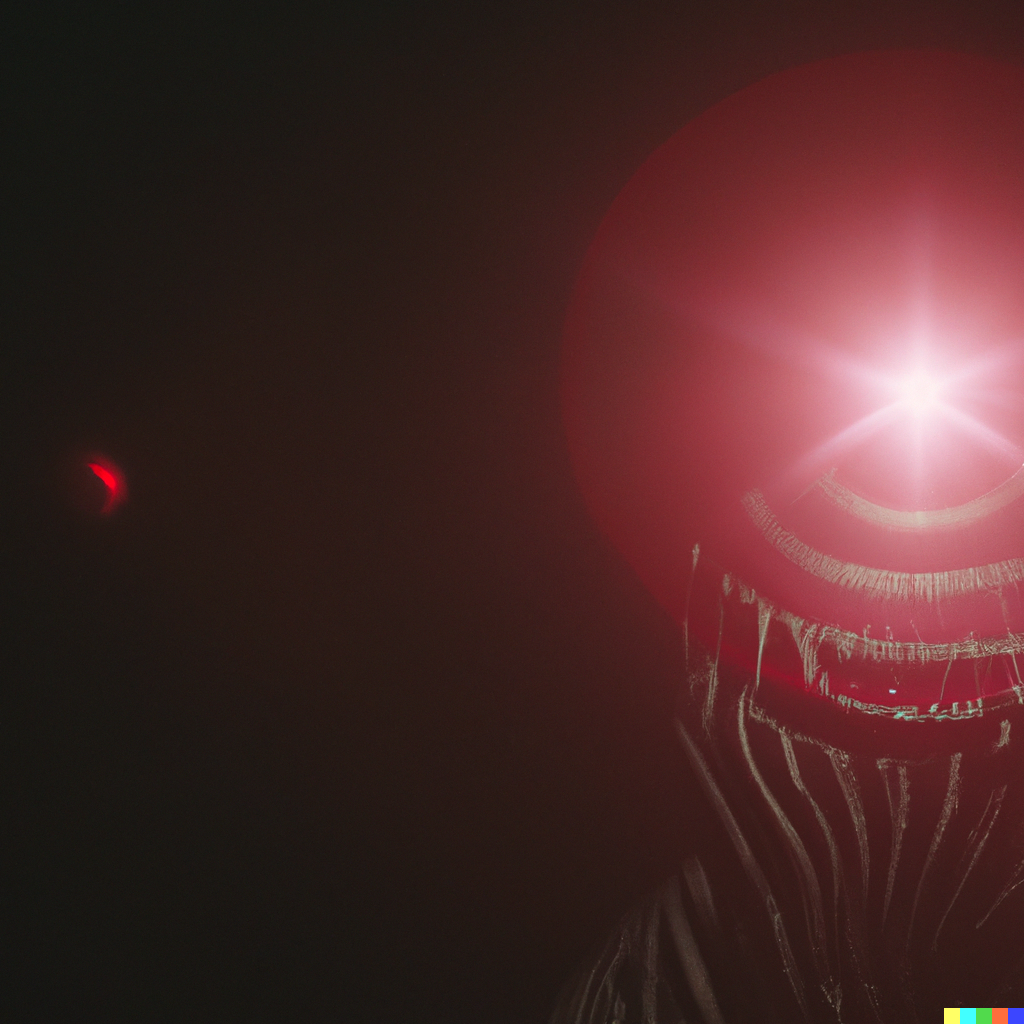
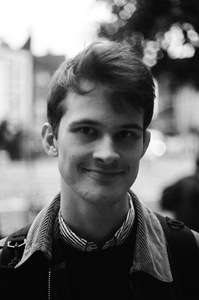
🔬 Research summary by Konrad Kollnig, an Assistant Professor at the Faculty of Law of Maastricht University, a leading institution for European law.
[Original paper by Konrad Kollnig and Qian Li]
Overview: The concentration of power among a few technology companies has become the subject of increasing interest and many antitrust lawsuits. In the realm of generative AI, we are once again witnessing the same companies taking the lead in technological advancements. Our short workshop article examines the market dominance of these corporations in the technology stack behind generative AI from an antitrust law perspective.
Introduction
Antitrust law has long been on the books. As early as 1890, the Sherman Antitrust Act sought to restrict anti-competitive and monopolistic corporate behavior in the US. It famously led to the break-up of Standard Oil in 1911, which was the largest and most influential oil company of its time.
In digital markets, the assessment of market dominance and abuse of this dominance in tech is still an evolving area of scholarship and not finally settled. Major challenges remain the complexity of digital ecosystems and the resulting long duration that antitrust cases take. In the European Union, both the Google Android (leading to a €4.125bn fine for Google) case and the Google Shopping case (leading to a €2.42bn fine for Google) took many years to be developed by the European Commission, and then to be settled before the Court of Justice of the European Union (about four years each).
These past fines highlight the risk of abuse of dominance by a few players in new technology markets, like those around machine learning and generative AI, and thus motivated us to look deeper in a short workshop paper that is to be presented at the inaugural Workshop on Generative AI and Law in late July.
Key Insights
Elements to success in generative AI
In generative AI, various elements are part of market success. The most important among them is access to large amounts of quality data, top talent and expertise, vast financial resources, suitable infrastructure for development and training, and cutting-edge models trained upon those elements. A further aspect cutting across these is participation in, leadership of, and providing funding for (academic) research.
The dominance of data access
The foundation of any model is a large source of data. To a large extent, generative AI in vision and text has been using data from the public domain. Thus far, such data can be gathered with relatively few resources. However, the incumbents still have some competitive edge. While Microsoft, the owner of GitHub, is unclear about whether it has used private repositories to train its Copilot code completion tool, it might – in any case – have used them for model testing. Similarly, BloombergGPT is an example of using NLP for finance and training on Bloomberg’s proprietary data that has been amassed over many decades.
The dominance of access to talent and resources
Once the relevant data is obtained, a suitable model architecture must be conceived and developed. Since deep learning remains more an engineering discipline than actual science with strong theoretical foundations, this requires access to many highly skilled engineers. The salaries for these engineers are beyond imagination for many individuals and usually go into six-digit territory, if not beyond. Only some of the most well-funded companies, like tech companies, can pay those salaries. Significant financial resources are also necessary to train the very machine learning models. According to Sam Altman, the CEO of OpenAI, the cost of training the GPT-3 model ran into tens of millions of US dollars. The training of such models often happens in proprietary infrastructures (e.g., Amazon AWS or Google Cloud), on proprietary hardware (e.g., Google’s TPU), and using industry-dominated frameworks (e.g., Google’s TensorFlow).
Influence on and independence of research
A further aspect, that is underlying the previous ones, is the fact that a few companies have a major influence on academic research. For example, one study found that 97% of computer science faculty with a focus on ethics at top universities had received funding from Big Tech companies. [1] At the top machine learning conference, many reviewers and authors work for those same companies.
Promises of open-sourcing
In the end, the result is the trained model, of which the most powerful are currently proprietary. While open sourcing is currently discussed as a solution, this might also create further risks and may not address the issues of a concentration of capabilities with a few actors in GenAI.
Google’s vertical integration in (generative) AI
Based upon the above analysis, Google stands out as a company with a high level of vertical integration in the (generative) AI stack. It might be argued that despite this dominance, Google has not (yet) managed to deploy an LLM that can compete with OpenAI/Microsoft’s GPT-4. However, it has also been argued that Google has more to lose in terms of its reputation, being one of the most trusted sources of information on the internet and with generative AI commonly spreading false information.
Vertical integration may not be a problem – experts disagree
Vertical integration is traditionally seen as a concern for antitrust intervention. Companies that are vertically, but not horizontally, integrated still have to compete with various other companies in each of the different markets along which this vertical integration occurs. Apple is the perfect example of such a vertically integrated company since it controls many market aspects in the iOS ecosystem, stretching from the development of the raw components for iPhones to the development of the iOS operating system. However, this theory has been questioned, most notably by scholars like Lina Khan [2] and Tim Wu [3], and is currently being challenged in court.
Between the lines
The translation between law and tech remains a key challenge in regulating digital ecosystems. As we continue to produce new technology laws with a limited deep and practical understanding of those technologies, we should not be surprised if this mainly serves the most well-resourced players and goes against the aims and fundamental rights and freedoms that such laws seek to protect. Indeed, the upcoming EU AI Act may worsen inequities around AI technologies and reinforce existing market dominance in tech. The latest draft adopted by the EU Parliament includes, among other aspects, stringent obligations for generative AI, even if free and open-source, provisions concerning API access to foundation models, extraterritorial scope, and high potential fines for non-compliance. This might then profit those companies that already have vast resources and may let everyone else pay higher prices than necessary. It remains to be seen if this law will go ahead as it currently stands (since it just left the committee stage) and whether it will have much of a tangible effect or will merely be another paper tiger without much immediate practical relevance like the EU’s General Data Protection Regulation (GDPR). [4]
References
[1] Abdalla, M. and Abdalla, M. 2021. The Grey Hoodie Project: Big Tobacco, Big Tech, and the Threat on Academic Integrity. Proceedings of the 2021 AAAI/ACM Conference on AI, Ethics, and Society (Virtual Event USA, Jul. 2021), 287–297.
[2] Khan, L.M. 2017. Amazon’s Antitrust Paradox. The Yale Law Journal. 126, 3 (2017), 96.
[3] Wu, T. 2018. The Curse of Bigness: Antitrust in the New Gilded Age. Columbia Global Reports.
[4] Kollnig, K. 2023. Regulatory Technologies for the Study of Data and Platform Power in the App Economy. PhD Thesis, University of Oxford. https://kollnig.net/phd-thesis