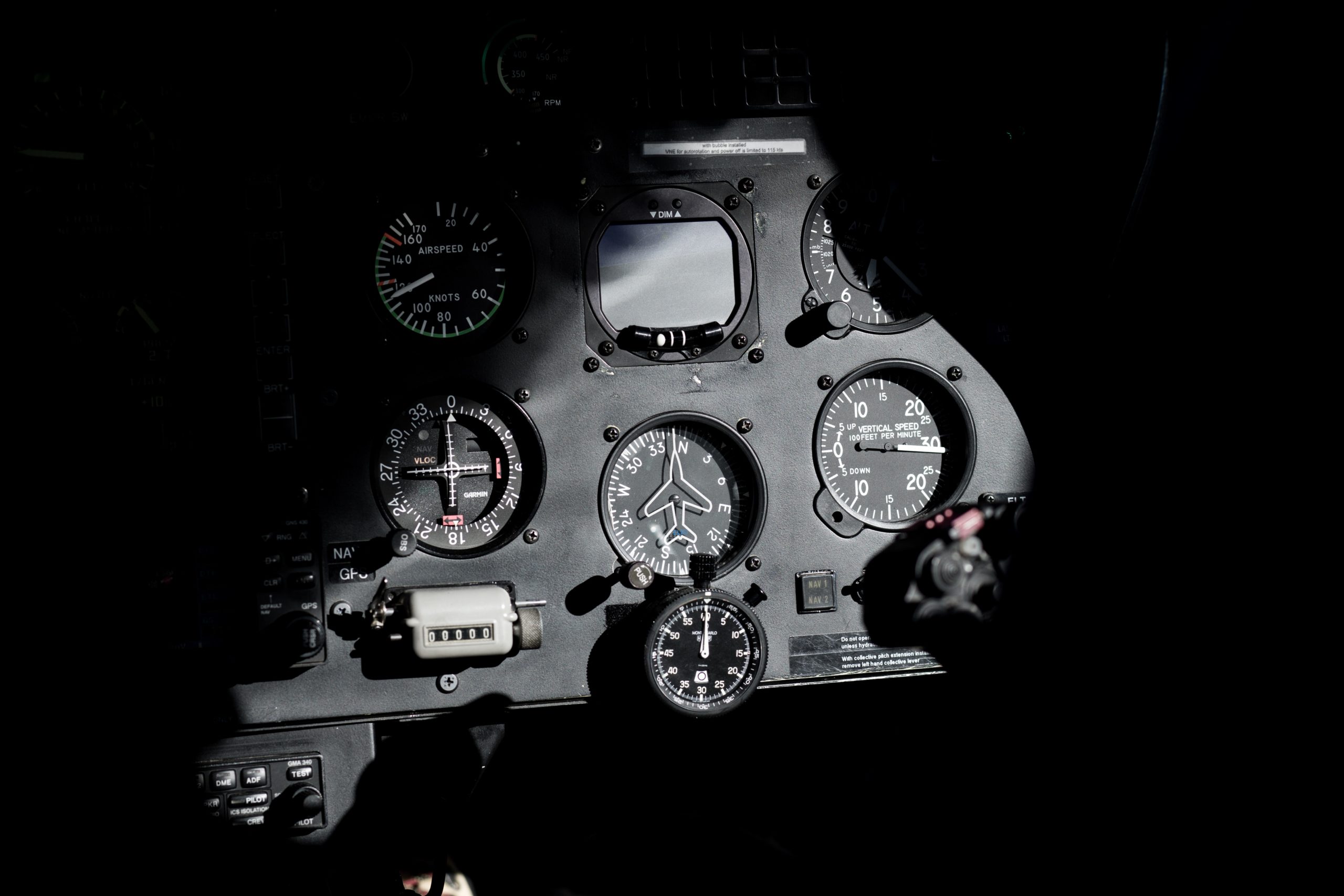
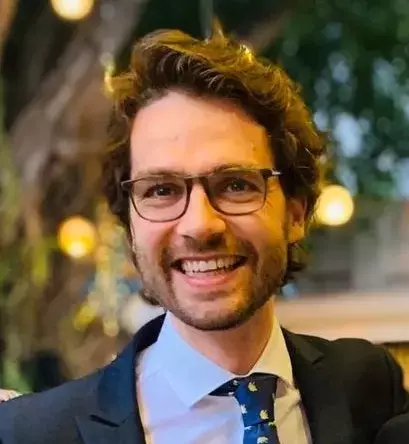
š¬ Research Summary by Roel Dobbe, an Assistant Professor working at the intersection of engineering, design and governance of data-driven and algorithmic control and decision-making systems.
[Original paper by Roel Dobbe]
Overview: The governance of Artificial Intelligence (AI) systems is increasingly important to prevent emerging forms of harm and injustice. This paper presents an integrated system perspective based on lessons from the field of system safety, inspired by the work of system safety pioneer Nancy Leveson. For decades, this field has grappled with ensuring safety in systems subject to various forms of software-based automation. This tradition offers tangible methods and tools to assess and improve safeguards for designing and governing current-day AI systems and to point out open research, policy, and advocacy questions.Ā
Introduction
Have you ever thought about what it takes to keep an airplane in the air and ensure you can fly around the world safely and comfortably? In addition to a well-designed plane, we rely on skilled pilots, stewards, ground personnel, regular maintenance, a spectrum of standards and procedures, various safety audits, complex air traffic management, infrastructure across the airspace, and intimate political agreements across the globe.
Now think about how we portray artificial intelligence these days. Typically: as a āthing on its ownā; as a robot or chatbot that can independently execute tasks and deliver services; as the magical solution to many current-day problems.
How do we keep AI systems safe? The dominant thinking goes like this: āAs long as we feed it enough data; provide technical fixes for things like explainability or fairness; think carefully about ethical choices and implications, and provide some documentation to ensure transparency, we must be safe, right?ā
Unfortunately, the answer is āno ā we need a lot more.ā But also: āwe can and should learn from the history of aviation and other safety-critical domains.ā This paper covers lessons from system safety and sketches what we can do to prevent harm and ensure safety in current-day AI and algorithmic systems.
Key Insights
Safety in AI is complex and āsocio-technicalā
System safety arose in the 1950s in aviation and aerospace when engineered systems became too complex to safeguard with traditional safety engineering methods. Thinking in terms of ācausal event chainsā leading up to a possible accident and the typical quantitative risk methods could no longer account for or capture the kinds of complex situations that could lead to a failure or accident.
Central to this complexity was the realization that accidents emerge from intimate interactions between technology, human operators or users, and the surrounding context of formal rules and norms and informal culture and behavior. Safety is, therefore, inherently a āsociotechnicalā property that can only be understood and controlled across a broader system of social, technical, and institutional components.
A weighty example
To make this concrete, letās consider a recent example; the two crashes with the Boeing 737-MAX in 2018 and 2019. These were quickly reported to have emerged from the redesign of the airplane with larger and more efficient engines to compete with a competitorās new airplane model.
While the crashes could be understood as resulting from faulty sensors and an ill-designed automation system, the US House of Representatives found that there were other critical factors at play that can largely be attributed to failing management and governance, namely: (1) giving in to financial pressure to compete with the rivaling Airbus A320neo plane; (2) faulty assumptions about critical technology and pilot performance; (3) a culture of concealment internally at Boeing and towards the authorities; (4) conflicts of interest between Boeing and the authorities; and (5) disproportional influence of Boeing over its oversight.
Unfortunately, these factors are well known to be constitutive of accidents, in particular for systems in which software-based automation plays a central role. Their social and managerial nature underlines the necessity of a system-theoretic lens to understand and prevent accidents and harm.
Looking at the emerging landscape of AI safety, AI ethics, and AI policy and governance, the worrying conclusion is that most efforts need to interpret safety in this systemic manner sufficiently. There is work to do to ensure we think about safety in effective ways to prevent AI systems go awry in similar ways across the many domains in which these are increasingly integrated and dominating.
Levesonās Lessons for system safety
System safety pioneer Nancy Leveson outlined the core learnings from system safety into seven important assumptions. In my paper, I interpret these for AI systems today, providing various examples and pointing to practical tools and methods to apply system safety analysis in AI system design and governance.
- Shift Focus from Component Reliability to System Hazard Elimination: A well-performing AI model is not sufficient, nor is it always necessary for safety. To prevent safety issues, one needs to look at how that model is embedded in a context of use and its broader impacts and implications.Ā
- Shift from Event-based to Constraint-based Accident Models: To understand (possible) accidents, look at the entire sociotechnical system in which the AI operates, including the environment enabled and governed by management and authorities.
- Shift from a Probabilistic to a System-theoretic Safety Perspective: Rather than touting your AI model by its performance, assume that your AI system will fail and show that you know what to do when it happens to prevent harm.Ā
- Shift from Siloed Design and Operation to Aligning Mental Models: Accidents are often unfairly attributed to operator error. Instead, designers are responsible for the environment in which such errors occur and need to assume that their mental model of what the AI system can and cannot do and how to operate it will differ from the people they design it for.
- Curb the Curse of Flexibility in AI Software Development: Perhaps the most pressing for modern AI systems (especially those relying on deep learning) ā the most serious safety problems arise āwhen nobody understands what the software should do or even what it should not doā (quote from Leveson). AI models and software are a prime source of safety hazards and should be treated as such.
- Translate Safety Constraints to the Design and Operation of the System: AI systems tend to migrate to states of higher risk as safety defenses degenerate, often under financial or political pressure, as we saw in the Boeing case. Therefore, ongoing feedback mechanisms are needed to learn from mistakes and anticipate potential safety hazards transparently and professionally.Ā
- Build an Organization and Culture Open to Understanding and Learning: Culture is critical. Without the ability to safely share possible issues or factors that could lead to safety problems and an effective follow-up by the organization, an AI system is doomed to fail.
Between the lines
The stakes of AI systems are high, especially where these establish unsafe conditions in social domains, thereby often reifying historical power asymmetries. In many ways, the harms that emerge are externalized, i.e., not treated as part of the AI system design.
A system safety perspective could help empower those subjected to either ill-willed or unprincipled faulty AI systems by better understanding how such harms emerge across the technical, social, and institutional elements in the broader context, thereby bringing responsible actors and powerful players into view. We have done this for aviation and medicine, too: when things go wrong, there are procedures to carefully understand what happened to prevent more harm in the future.
Often, a full spectrum of actors is involved, from the users and developers of technology to supervisory bodies, auditors, regulators, and civil society. This tells us that it often takes much more to responsibly and sustainably safeguard complex systems subject to software-based automation. As such, the lessons and tools from system safety are useful to understand what is needed to prevent new forms of harm in contexts where AI is relatively new and to inform standards that the design and governance of such systems should meet based on decades of experience.