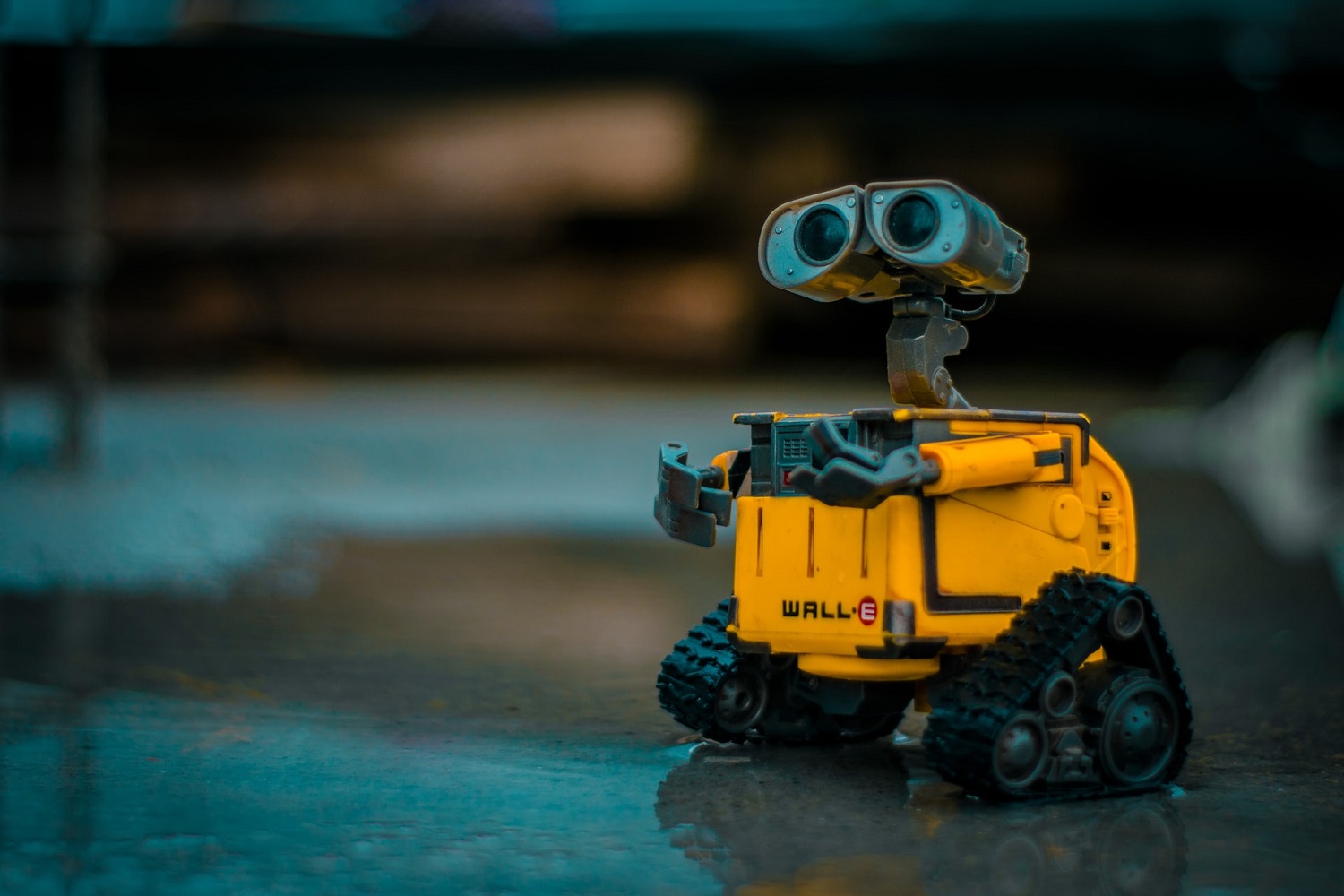
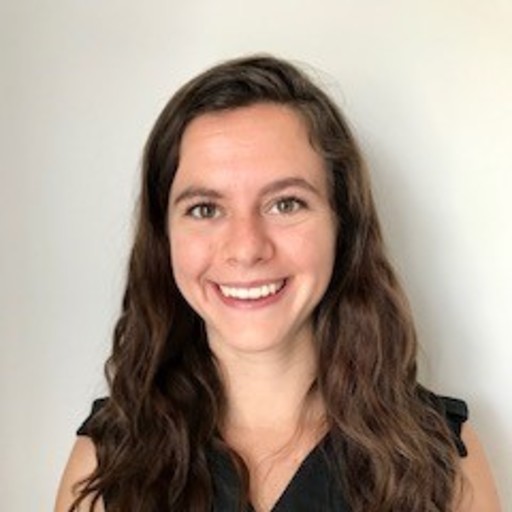
Written by Alexandrine Royer, our Educational Program Manager.
Introduction (Excerpt from full guide)
In the 1950s, computer scientist Alan Turing designed a test; a machine would be considered ‘intelligent’ if a human interacting with it could not tell whether it was a person or a machine. It was the first step in the development of what would become the field of Artificial Intelligence (AI), a term first coined by John McCarthy at the seminal Dartmouth summer research project in 1956. In the short span of seventy years, the production of intelligent machines has evolved beyond the scope of human imagination. No longer limited to sci-fi aficionados and the scientific community, artificial intelligence has become ubiquitous in each of our lives. We interact with AI, whether knowingly or unknowingly, daily when using our phones, digital assistants, applying for loans, undergoing medical treatment, or just browsing the web. Companies across the board are scrambling to adopt AI and machine learning technology. Opinions, hopes, and fears ranging from utopia to catastrophe accompany this growing proximity with artificial intelligence systems – Stephen Hawkings’ infamously prophesied that AI could spell the end of humanity.
The development of technology has brought on a series of significant advances, such as improved medical imaging, new video communication technology, 3-D printed affordable homes, drones for service deliveries in conflict areas, etc. AI has proven it can produce immense social good. However, every new technology comes with considerable caveats, which we tend to observe once set in motion. The rapid expansion of consumer Internet over the past two decades has led to the explosion of algorithmic decision-making and predictions on individual consumers and behaviour. Before we could even agree to the collection of our data, private corporations, banks, and the public sector used it to make crucial decisions on our lives. Over the years, data scientists and social scientists have started to signal incidents where algorithms violate fundamental social norms and values. Algorithms trampled on notions of privacy, fairness, equality, and were revealed to be prone to manipulations by its users. These problems with algorithms have led researchers Michael Kerns and Aaron Roth to state that “it is less a concern about algorithms becoming more powerful than humans, and more about them altering what it means to be human in the first place.”
Over the next few years, society as a whole will need to address what core values it wishes to protect when dealing with technology. Anthropology, a field dedicated to the very notion of what it means to be human, can provide some interesting insights into how to cope and tackle these changes in our Western society and other areas of the world. It can be challenging for social science practitioners to grasp and keep up with the pace of technological innovation, with many being unfamiliar with the jargon of AI. This short guide serves as both an introduction to AI ethics and social science and anthropological perspectives on the development of AI. It intends to provide those unfamiliar with the field with an insight into the societal impact of AI systems and how, in turn, these systems can lead us to rethink how our world operates.
Before delving into anthropology’s contributions to AI, a brief overview of the ethical issues in technology will help situate some of the critical failures of algorithmic design and their integration into high-stakes decision-making areas. Exploring the limitations of ethically fine-tuned, or better-behaved, algorithms in the areas of privacy, fairness, and user model manipulation elucidates how ethical AI requires input from the social sciences. The current controversies in which technology giants are enmeshed show that society cannot entrust Silicon Valley entirely to pave the way to produce ethical AI. Therefore, anthropological studies can assist in determining new avenues and perspectives on how to expand the development of ethical artificial intelligence and machine learning systems. Ethnographic observations have already been used to understand the social contexts in which these systems are designed and deployed. By looking beyond the algorithm and turning to the humans behind it, we can start to critically examine the broader social, economic and political forces at play in the rapid rise of AI and ensure that no population nor individuals are left to bear the negative consequences of technological innovation.
Brief Overview of the Ethical Issues in Tech (Excerpt from full guide)
In the past few years, there has been an explosion of ethical concerns raised by technology and its harm to specific groups of people. Researchers have pointed to repeated algorithmic bias cases, whether it be racial, political or gender, and data discrimination. Human rights organizations, lawmakers, and even practitioners have raised alarm bells over the industry’s pervasive problem. We all come into contact with these biases daily; they impact how we structure our knowledge and view reality. Safiya Umoja Noble, in her book Algorithms of Oppression, documents how our most commonly used search engines, from Google to Yahoo, are biased towards certain population groups. In one example, Noble pointed to how the terms associated with black girls, Latina girls, and Asian girls in search engines differed widely from those related to white girls. The top results for women of colour led to pornography sites and sexualized content. Noble argues that the limited number of search engines, compounded by private interests driving the results page, has led to recommender systems that privilege whiteness over people of colour, thereby reinforcing racist notions.
Blind trust in technology’s merits over human capacities can lead to grave oversights in areas where machines make life-altering decisions. The allure of modernity tends to gloss over entrenched social inequalities. In Weapons of Math Destruction, Cathy O’Neil uncovers how big data and algorithms can lead to decisions that place minorities, people of colour, and the poor at a disadvantage, further reinforcing discrimination. Although these algorithms make high-stakes decisions – such as determining mortgage eligibility and assessing recidivism rates in bail decisions – they operate in opaque, unregulated, and challenging to control. The pernicious feedback loops created by some of these algorithms, such as the ones employed in predictive policing, lead specific populations to suffer in unequal ways without consenting to the use of their personal information. These algorithms not only pose a threat to principles of fairness, privacy, and justice but also hamper the functioning of a healthy democracy. As revealed by the Cambridge Analytica scandal in 2018, microtargeting political ads on social media sway individuals towards a particular candidate by harvesting information from their user profile.
Rampant disinformation weakened democracy, wealth, and racial inequalities, the impact of automation on the labour market, and user mental health are some of the slew of issues that our technology-shifting society needs to address. The answers to these problems should not be placed solely within the hands of the technology companies themselves. Social media platforms have become too big to effectively monitor and are part of a market-based system that encourages relentless growth. Politicians accused Facebook of failing to prevent the genocide in Myanmar when fake pages and sham accounts helped incite violence against the Muslim Rohingya minority. YouTube has repeatedly come under fire for failing to stop the multiplication of conspiracy and alt-right videos on users’ recommendation lists. Furthermore, YouTube’s algorithm rewards videos with high engagement levels, which has popularized controversial content by far-right personalities, making it a pipeline for extremism and hate.
It would be wrong to assume that the original intent of data engineers at Google, Facebook, or Youtube was to amplify biases, incite violence, undermine democracy, or empower autocrats. As several data scientists have indicated, when algorithms reveal themselves to be racist, sexist, or prone to inflaming hateful discourses, it is often the result of good intentions gone awry. One oft-cited example is Amazon’s scraped recruiting tool, where the system, trained on past resumes and CVs that reflected the dominance of men in the industry, had thought itself that male candidates were preferable over female ones. The Amazon engineers did not purposely set out to exclude women from its hiring procedure; it is merely what the machine learning system learned based on the data it was fed. We must not view algorithms as providing objective, neutral and fair results that are more reliable than those produced by their human counterparts. On the contrary, as O’Neil describes, “algorithms are embedded opinions” that “automate the status quo.” Recognizing, addressing, and extracting these biases from machine learning systems is not just a technical problem, but a social one too. Addressing biases is a time-pressing issue as we integrate complex machine learning systems into the domains of medicine, warfare, credit allocation, judicial systems, and other areas in which high-stakes decisions affect human lives. The issues listed above raise ethical concerns for AI operating in democratic countries, but only begin to reflect the potential for abuse of technological power in authoritarian regimes. China has been at the center of international controversies over its use of AI. Beijing’s social ranking system that can subtract citizen rights and its use of facial recognition technology to target and monitor the oppressed Uighur minority is widely criticized. China’s export of its surveillance state apparatus to other dictatorial regimes in Africa and Latin America is the subject of much international criticism. The expansion of tech surveillance and decision-making apparatus is not just a concern for the Western world, but the international community. Just like norms, rules, and regulations vary across countries, the development of ethical AI will need to take into account local specificity in our globalized world.