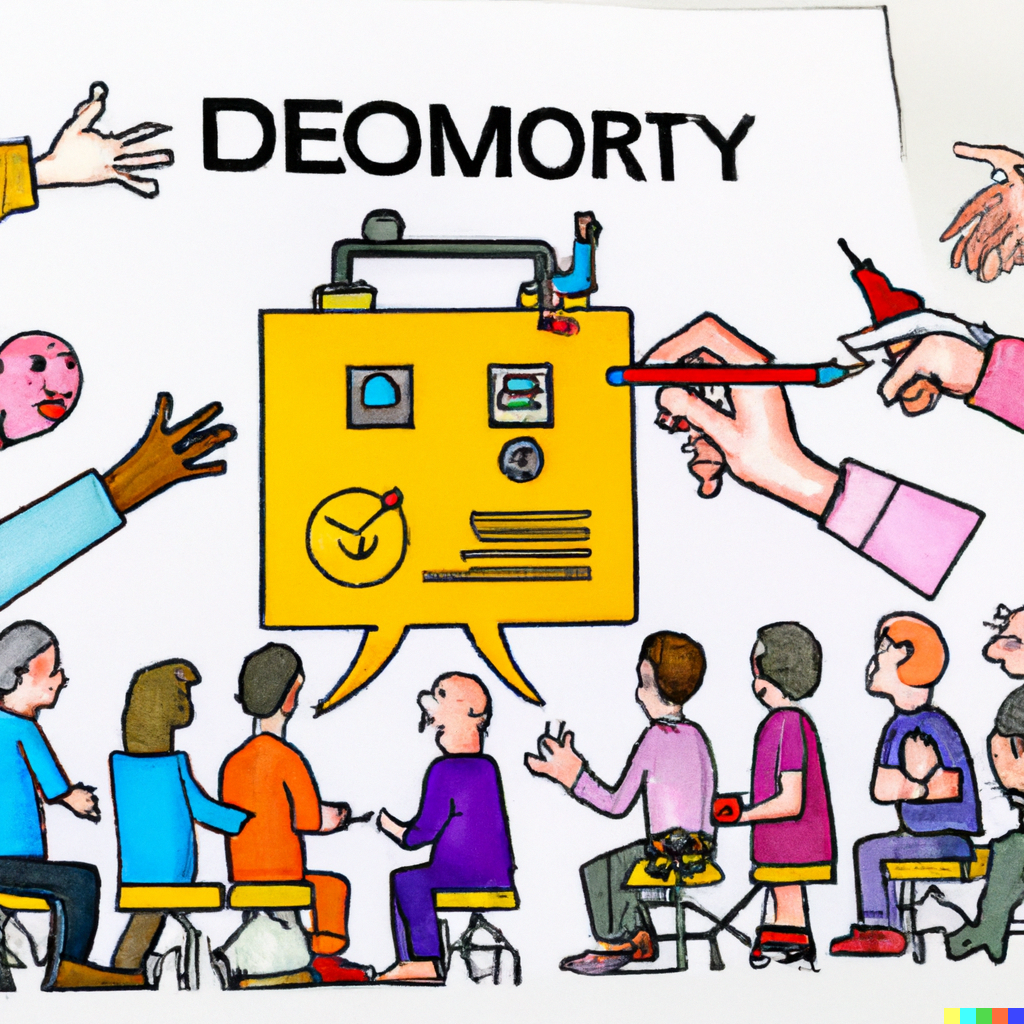
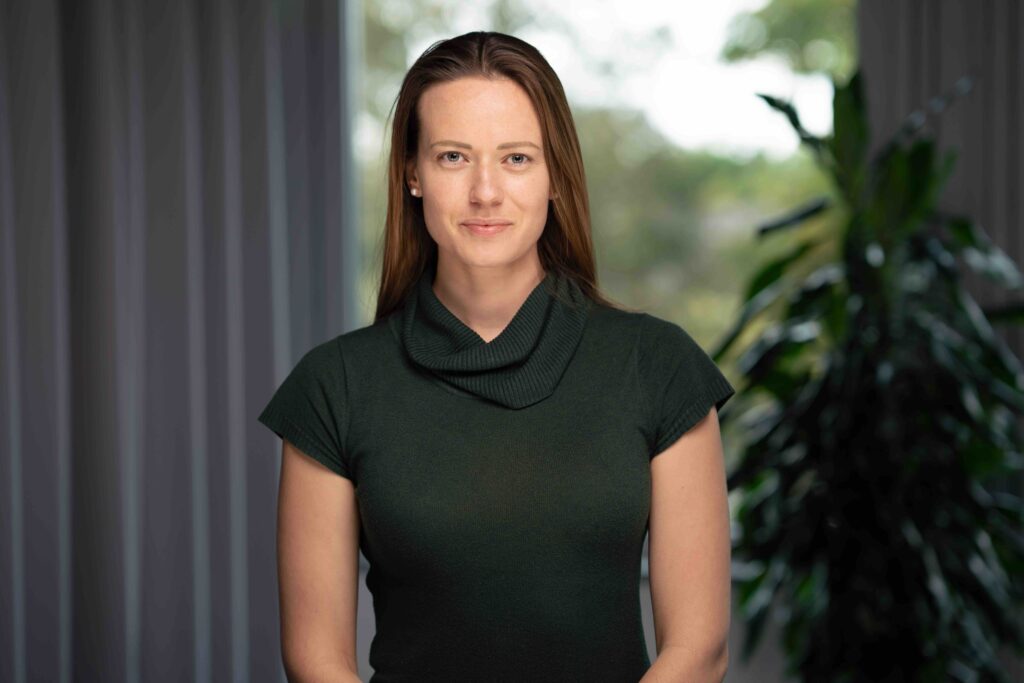
🔬 Research Summary by Elizabeth Seger, PhD, a researcher at the Centre for the Governance of AI (GovAI) in Oxford, UK, investigating beneficial AI model-sharing norms and practices.
[Original paper by Elizabeth Seger, Aviv Ovadya, Ben Garfinkel, Divya Siddarth, Allan Dafoe]
Overview: Numerous parties are calling for “the democratization of AI,” but the phrase refers to various goals, the pursuit of which sometimes conflict. This paper identifies four kinds of “AI democratization” that are commonly discussed—(1) the democratization of AI use, (2) the democratization of AI development, (3) the democratization of AI profits, and (4) the democratization of AI governance—and explores the goals and mentors of achieving each. This paper provides a foundation for a more productive conversation about AI democratization efforts and highlights that AI democratization can not and should not be equated with AI model dissemination.
Introduction
In recent months, the discussion of “AI democratization” has surged. AI companies worldwide—such as Stability AI, Meta, Microsoft, and Hugging Face—are talking about their commitment to democratizing AI, but it’s unclear what they mean. The term “AI democratization” seems to be employed in various ways, causing commentators to speak past one another when discussing the goals, methodologies, risks, and benefits of AI democratization efforts.
This paper identifies four different notions of “AI Democratization” commonly used by AI labs—the democratization of AI use, the democratization of AI development, the democratization of AI profits, and the democratization of AI governance—which often complement each other, though sometimes conflict. For instance, if the public prefers for access to certain kinds of AI systems to be restricted, then the “democratization of AI governance” may require access restrictions to be put in place—but enacting these restrictions may hinder the “democratization of AI development” for which some degree of AI model accessibility is key.
This paper illustrates the multifaceted nature of AI democratization. It drives home two important points: (1) AI Democratization is not the same as model dissemination, and (2) the positive value of AI democratization is rooted in how well they respond to the interests and values of those impacted.
Key Insights: Kinds of AI Democratization
Democratization of AI Use
When people speak about democratizing some technology, they often refer to democratizing its use—that is, making it easier for a wide range of people to use the technology.
Goals:
The most common goal of democratizing AI use is to distribute the benefits of AI use for many people to enjoy. Benefits include entertainment value (e.g., generating poems with ChatGPT), health and well-being applications, productivity improvement, and other utility functions (writing code, analyzing data, creating art). Many of these benefits could be translated into financial gains for those who effectively integrate AI tools into their workstreams.
However, it is important to recognize that for some AI applications, the benefits of making the technology available for anyone to use can be relatively minor while the risks are significant. For example, the circle of individuals who would greatly benefit from access to an AI drug discovery tool is relatively small (mainly pharmaceutical researchers), however, these tools can be easily repurposed to discover new toxins that might be used as chemical weapons.
Methods:
Efforts to democratize AI use involve reducing the costs of acquiring and running AI tools and providing intuitive interfaces to facilitate human-AI interaction without extensive training or technical know-how.
Democratization of AI Development
When the AI community talks about democratizing AI, they rarely limit their focus to democratizing AI use. Much of the excitement is about democratizing AI development—that is, helping a wider range of people contribute to AI design and development processes.
Goals:
Often, the idea is that tapping into a global community of AI developers will accelerate innovation and facilitate the development of AI applications that cater to diverse interests and needs. It is also argued that involving more people (e.g., academics, individual developers, smaller labs) in AI development processes provides a critical external evaluation and auditing mechanism.
Methods:
Various activities can enable productive participation in AI design and development processes. Some strategies provide access to AI models and resources to facilitate AI community engagement—e.g., model sharing, improving compute access, providing project support and coordination. Other strategies help to expand the community of people capable of contributing to AI development processes—e.g., via educational & upskilling opportunities or through the provision of assistive tools.
But again, it should not be assumed that all methods of democratizing AI development are universally desirable. For example, open source model sharing, in particular, may enable more numerous and diverse contributions. However, it also opens a door for malicious use and model modification, and controls are difficult to enforce.
Democratization of AI Profits
A third sense of “AI democratization” refers to democratizing AI profits—facilitating the broad and equitable distribution of value accrued to organizations that build and control advanced AI capabilities.
Goals:
A few sub-aims of democratizing AI profits are to avoid widening a socioeconomic divide between AI-leading and lagging nations, to ease the financial burden of job loss to automation, to smooth economic transition in case of the rapid growth of the AI industry, and to provide mechanisms for labs to demonstrate their commitment to pursuing advanced AI for the common good.
Methods:
Profits might be redistributed, for instance, through philanthropic giving (e.g., via a commitment to a “Windfall Clause“) or the state via taxation.
Democratization of AI Governance
Finally, some discussions about AI democratization refer to democratizing AI governance. AI governance decisions often involve balancing AI-related risks and benefits to determine if, how, and by whom AI should be used, developed, and shared. The democratization of AI governance is about distributing influence over these decisions to a broader community of stakeholders and impacted populations.
Goals:
The overarching goal of the democratization of AI governance is to ensure that decisions around questions such as AI usage, development, and profits reflect the interests and preferences of the people being impacted.
Important subgoals include decentralizing control over AI away from big tech, navigating complex normative questions about AI that may vary between cultures, and ensuring the benefits and burdens of AI development and deployment are distributed justly and fairly.
Methods:
Proposed methods for democratizing AI governance decisions include harnessing existing democratic government structures, convening international multistakeholder bodies to deliberate on complex AI governance challenges, and employing promising modern participatory and deliberative governance approaches enabled by deliberative tools and digital platforms.
Between the lines:
AI Democratization is a multifarious term with numerous goals and methods by which those goals might be achieved. This observation highlights two important insights.
- AI Democratization is not the same as open-source model sharing.
An AI model is open source if the developer decides to allow anyone to download, modify, or build on the model on their computer so long as they agree to the terms of use. In popular discourse, “AI democratization” and “Open Source” are often uttered in the same sentence. The implication is that open-sourcing is somehow a necessary step toward democratizing AI. But this is not the case. The close association between AI democratization and open source needs to be broken.
The discussion above illustrates that there are many methods for achieving the goals of each kind of AI democratization (see full paper for more detail). Open-sourcing might facilitate the pursuit of some goals (mainly of democratizing AI use and development) but is not the only mechanism or the most important. A decision to open source would counter the democratization of AI governance, for example, if it does not respond to the interest and values of those likely to be impacted.
- AI democratization efforts are not inherently good.
This leads to the last point. Any AI democratization effort—whether model-sharing, distributing profits, eliciting stakeholder input, or building intuitive user interfaces—is not inherently good; its value is derived from alignment with the interests and preferences of those who will be impacted.
For this reason, the democratization of AI governance takes precedence over the others as the source from which the moral and political value of the “democratization” terminology is derived; the invocation of the “democratization” terminology implies that any decision (to share, restrict, distribute, develop, etc.) is that which a democratic governance process would select.