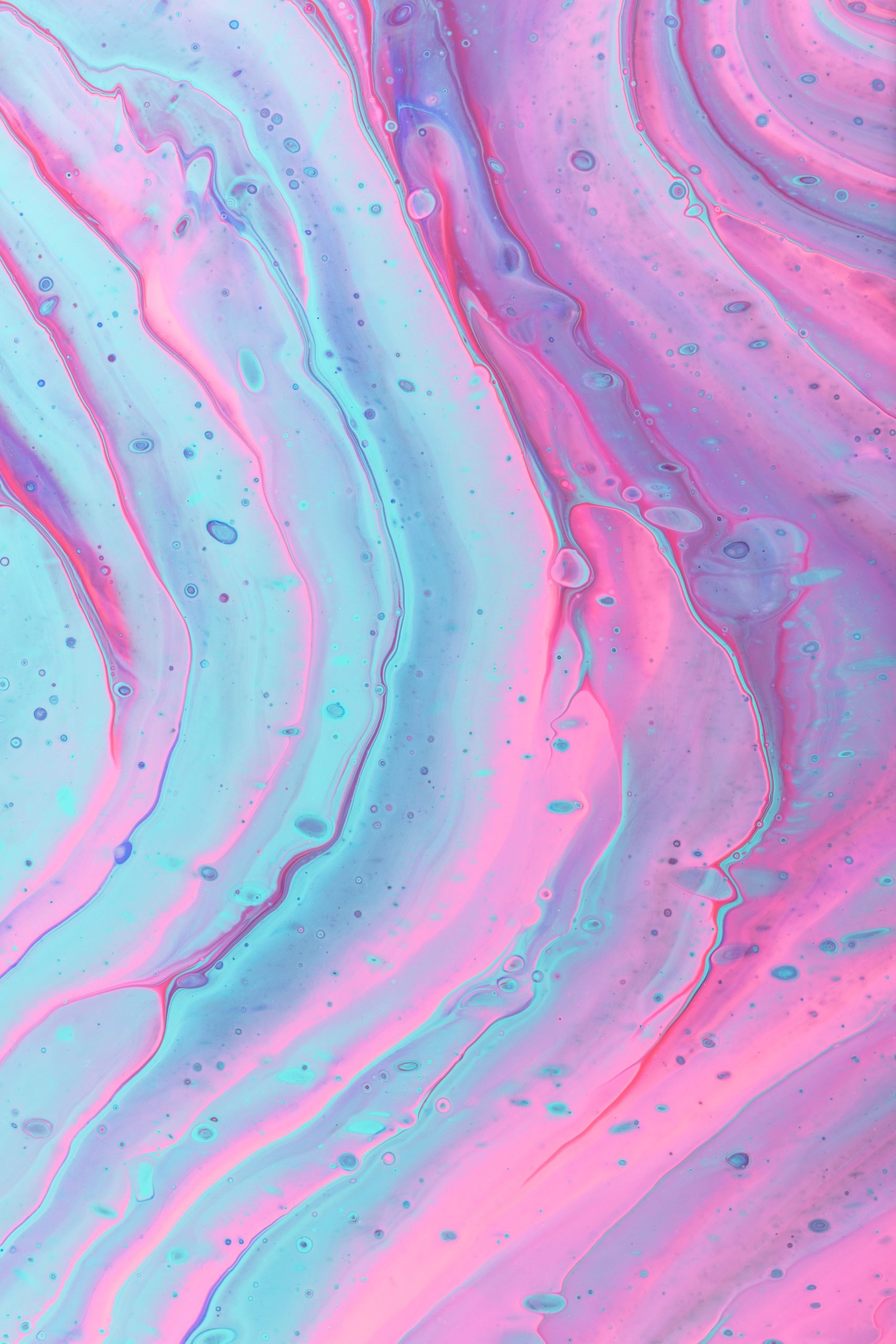
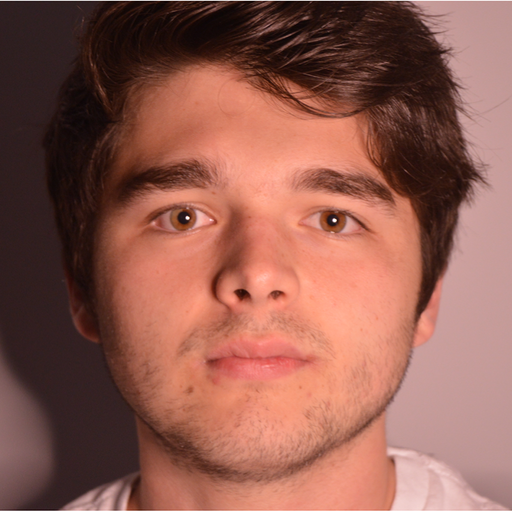
š¬ Video summary by Connor Wright, our Partnerships Manager.
[Original video by Jack Clark]
Overview: Who currently leads the way in terms of AI hiring? Is investment in AI simply concentrated in the same companies? Jack Clark guides us through the Stanford HAI Index, presenting the highlights and diving deeper into its individual chapters.
Introduction
Currently, 1 in 5 Computer Science PhD students specialise in AI. With the index in its 5th year, the perception of AI has clearly changed. Industry takeover has slowly started to erode the trust citizens place in the technology, while the capabilities of the technology have only expanded. Consequently, it becomes increasingly harder to keep on top of AI developments as it gets more and more integrated into the economy. Navigating us through the index, Jack Clark presents the highlights of the findings before taking a deeper dive into the chapters themselves.
Key Insights
The key highlights are as follows:
- Investment – thereās more money in AI now than ever before. For example, in 2013, between $1-2 billion was invested, with 2021 racking up $94 billion.
- Collaboration – despite the tensions, the US and China are the leaders in cross-country AI collaborations.
- Language models – they work. Yet, this means it can facilitate both positive and negative ends.
- The rise of AI Ethics – people are studying AI ethics more. Industry is investing more into the study, meaning the domain no longer just considers intangible and uninteresting problems.
- AI training is more efficient – thanks to todayās improved underlying compute, AI is cheaper to build. Training an AI model can cost you as little as $5.
- More global legislation – there is more legal involvement in the space than in previous years.
With the highlights as a backdrop, examining the chapters in more depth became the next move.
Chapter 1: Research and Development
According to the index, China is now leading the amount of AI publications published. However, quantity doesnāt mean quality, with the US leading in citations. Included in these citations is the steady rise in collaborations between academia and non-government, as well as academia and company collaborations.
Chapter 2: Technical Performance
AI has not gotten comfortable with complex language tasks, performing below the human baseline despite tremendous strides being made. Nevertheless, natural language processing has blown past the human baseline on the more straightforward tasks. Given this mastery, reinforcement learning takes on more complex tasks, becoming more competent at various activities. Consequently, the efficiency has allowed robotic arms to become cheaper.
Chapter 3: Technical AI Ethics
While language models have improved, they still suffer from the recurring problem of bias which cannot be solved on a technical basis alone. To quote Clark, āethical challenges are not the same as technical challengesā. The category of problems like bias requires a socio-techno attitude, especially when the problems are multimodal. Biases of this ilk are harder to deal with, showing how as AIās capabilities have increased, so has the complexity of its problems.
Chapter 4: The Economy and Education
New Zealand, Hong Kong, and Ireland lead the way in terms of hiring. Given their relatively more minor economies than the AI giants China and the US, they can specialise their economy and become a talent pool. Within the US, Texas, New York, Virginia, and California have the highest number of AI-related jobs. The country is leading the way in terms of newly funded companies and private AI investment, whereby 1 in every 5 Computer Science PhD graduates specialises in AI or machine learning.
Practically all areas measured (bar Ed Tech) have increased in investment since 2020. However, while private investment has soared, so has its concentration. The same companies are receiving the lion’s share of investment, with ādata management processing and cloudā acquiring the most AI private investment in 2021. Consequently, between 2010-and 2020, the majority of AI PhDs in the US went into industry.
Chapter 5: AI Policy and Governance
Bills containing āAIā passed into law went from 1 in 2016 to 18 in 2021, serving as an indication of a rising interest in the legal space. The state level has proved the most successful breeding ground for passing AI legislation within the US context.
Between the lines
I agree with Clark that one of the most surprising statistics within the report was how many Computer Science PhD candidates specialised in industry within the US. For me, given how the talent and interest are there, intertwining the importance of at least considering ethics within computer science work becomes even more significant. With AI becoming a priority in academia, receiving the necessary funding and being deployed, a so-called AI āfarm to forkā narrative can now be drawn. We have a better idea of where it is being developed, who is undertaking the task and what potential incentives they could be acting upon (such as pressures from industry). Hence, while the AI space has matured and now produces working models, acting upon the positive incentives placed on this dual-use technology will prove crucial.