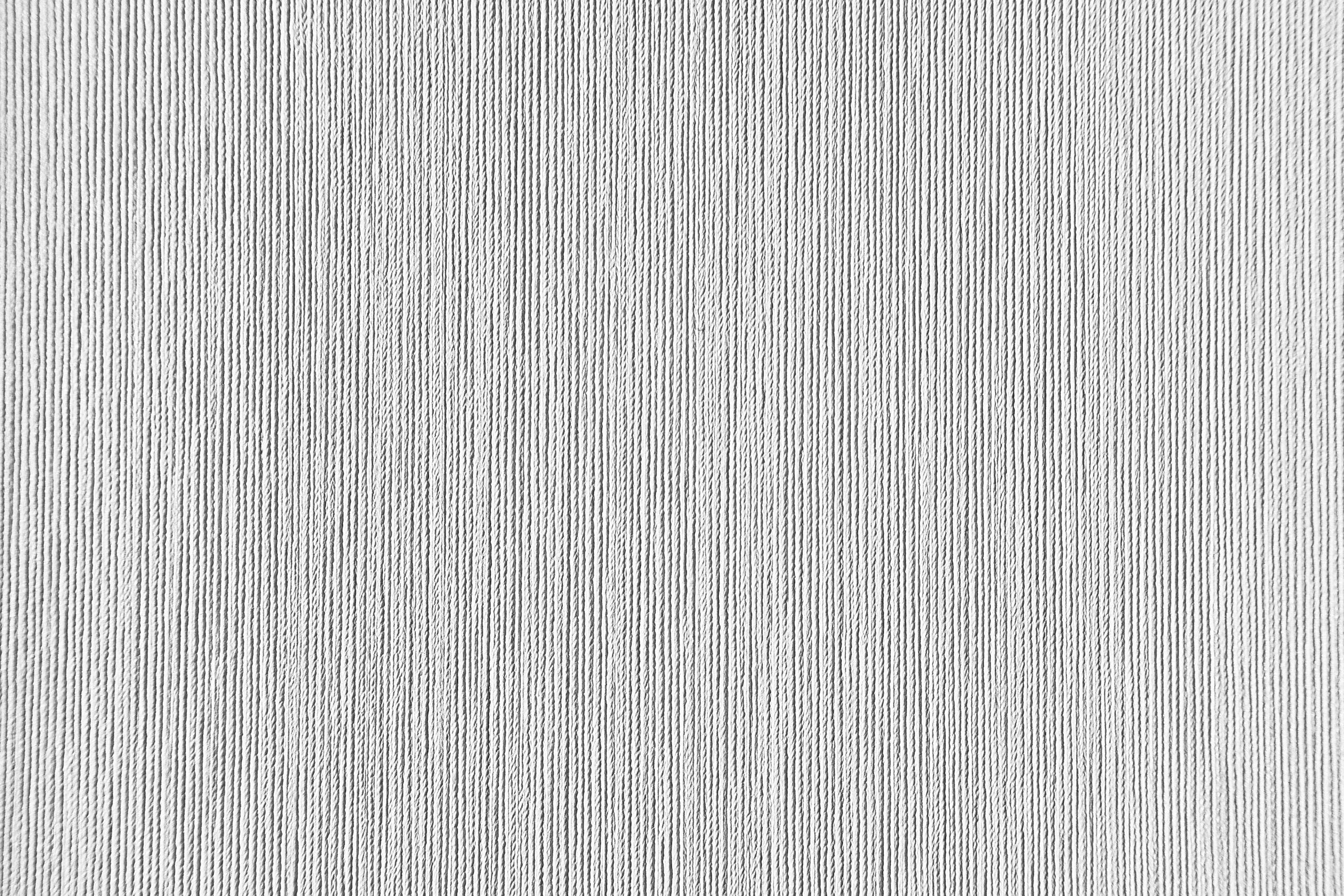
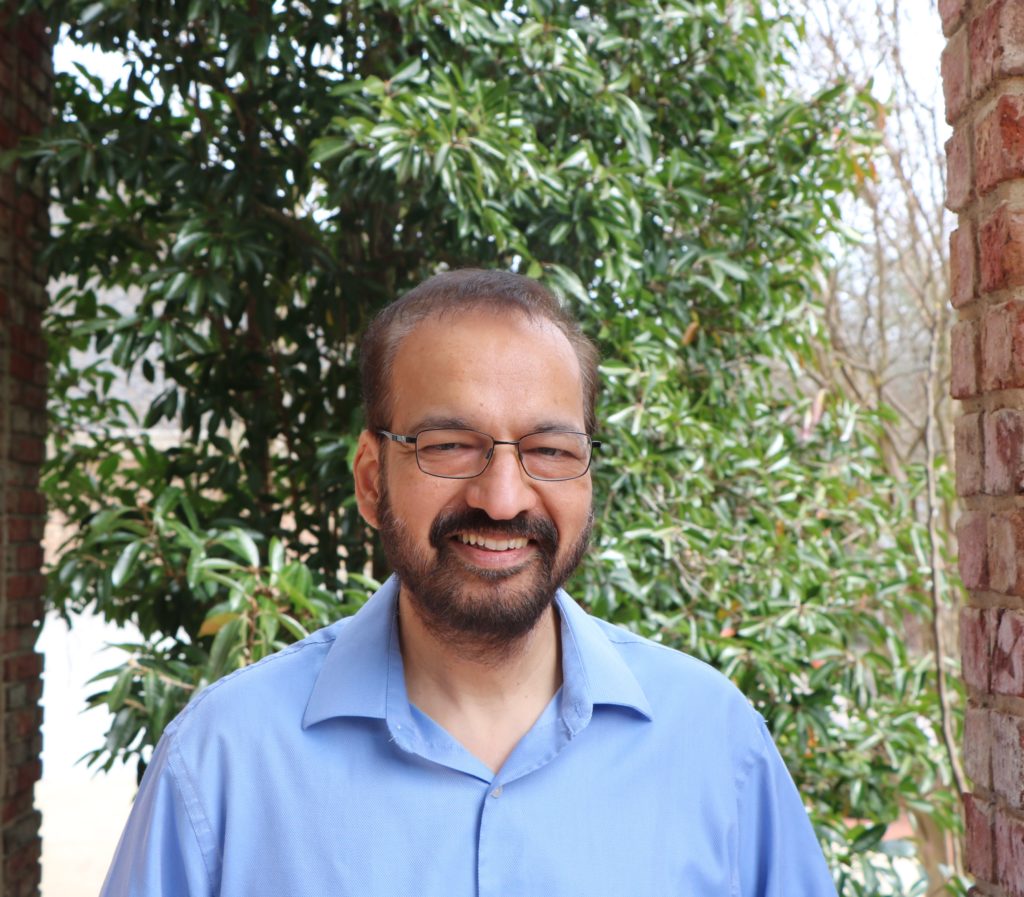
🔬 Research Summary by Munindar P. Singh, an Alumni Distinguished Graduate Professor in the Department of Computer Science at North Carolina State University.
[Original paper by Munindar P. Singh]
Overview: Consent is a central idea in how autonomous parties can achieve ethical interactions with each other. This paper posits that a thorough understanding of consent in AI is needed to achieve responsible autonomy.
Introduction
We all face having to give “consent” to get virtually anything done or obtain virtually any service–both in the virtual and the physical worlds. These include having to consent to install software, allow a software application to access some folder, use a website, or receive medical help. These interactions are becoming more important and more fraught with risk as AI is spreading.
User interface researchers are studying how to obtain consent more naturally. Others have objected to the prevalent notice and choice doctrine in computing. But consent is not inherently a bad idea, nor are its challenges primarily at the user interface level.
This paper steps back and takes a deeper look at consent, which surprisingly hasn’t been done in computer science. It takes a quick look at the literature in the law, political science, contracts, and computing. It places consent in a framework of governance for responsible autonomy.
The main contribution of this paper is to identify criteria for valid consent motivated by validity claims as proposed by the philosopher Habermas. It closes with research challenges for AI to ensure valid consent as a way to realize responsible autonomy.
Key Insights
Consent has been identified as a basis for autonomy from the time of Aristotle. Going through the development of political liberty and on to individual decision making, consent is a central idea in legitimizing the interactions of autonomous parties. Consent is often all that distinguishes illegal from legal acts and moral from immoral acts. It is, for example, the difference between being a guest and an invader.
So it’s not surprising that consent is used as a gating requirement for various interactions. For example, hospitals provide healthcare treatments to patients only under consent and banks obtain credit reports of loan applicants only under consent. In computing, obtaining consent is a step in installing software and storing cookies. However, many of these approaches, especially with computers where the processes are automated, are fraught with risk. Major pitfalls include the consenting party (the consenter) facing a power or information imbalance and having no practical choice but to give consent.
But what would valid consent be like? Consent is difficult to pin down. It has elements of a mental act – the consenter must have certain beliefs and intentions for their consent to be valid. And, it has elements of a communicative act – the consenter relies on social and legal norms to grant a power to the consentee. Both these approaches have shortcomings. The mental approach doesn’t explain that consent is public in that it changes the legitimacy of another party’s actions. The communicative approach doesn’t explain that consent can be erroneously given, e.g., if the consenter has false beliefs.
In contrast, this paper adopts the notion of validity claims as proposed by the philosopher Habermas in his theory of communicative action. Habermas proposes that three kinds of validity claims can be made about a communication. Objective validity concerns the empirical reality, e.g., is it true? Subjective validity concerns the states of the minds of the participants, e.g., is the speaker sincere? Practical validity concerns the context of the communication, e.g., is it justified?
When we view consent from the lens of the Habermas validity claims, we can identify key criteria for valid consent that reflect his classification. The objective validity of a consent maps to the consent being based on an observable action, granted with free will, and based on beliefs (i.e., assumptions about the consentee) that are true. The subjective validity of a consent maps to the consent being granted when the consenter is mentally capable, believes and intends to grant the consent, and while paying full or adequate attention. The practical validity of a consent maps to the consent being granted in a way that respects applicable laws and statutes, when the consenter is not under the power or influence of the consentee, and when the consentee doesn’t mislead the consenter. Some of these criteria can’t be evaluated “atomically” in that we can’t directly read them off a sensor or directly ask the user. To evaluate them, we would need to carry out extensive dialog with the user. But that doesn’t mean we can avoid them. Instead, we must expand our understanding of AI so that a responsible autonomous agent would be able to represent and reason about the relevant nuances that distinguish valid from invalid consent.
Between the lines
If we are ever going to field AI agents that exercise their autonomy responsibly, we must make sure that they obtain valid consent from users and grant valid consent to each other on behalf of their respective users. Only then can their interactions meet the moral standards we have as humans.
However, AI principles and techniques today are poorly equipped to support valid consent. Accordingly, this paper advocates research advances in five broad directions: (1) new models of legal and social norms and their relationship with communication, (2) bridging AI ethics and law to develop expressive models of duty and discretion, (3) design methods incorporating values to create AI, (4) formal verification techniques for AI ethics that go beyond quandaries such as the trolley problem to model how an AI agent facilitates legitimate interactions with users and between users, and (5) ways to develop AI agents that carry out user dialogs to continually adapt their standards of consent to user contexts.