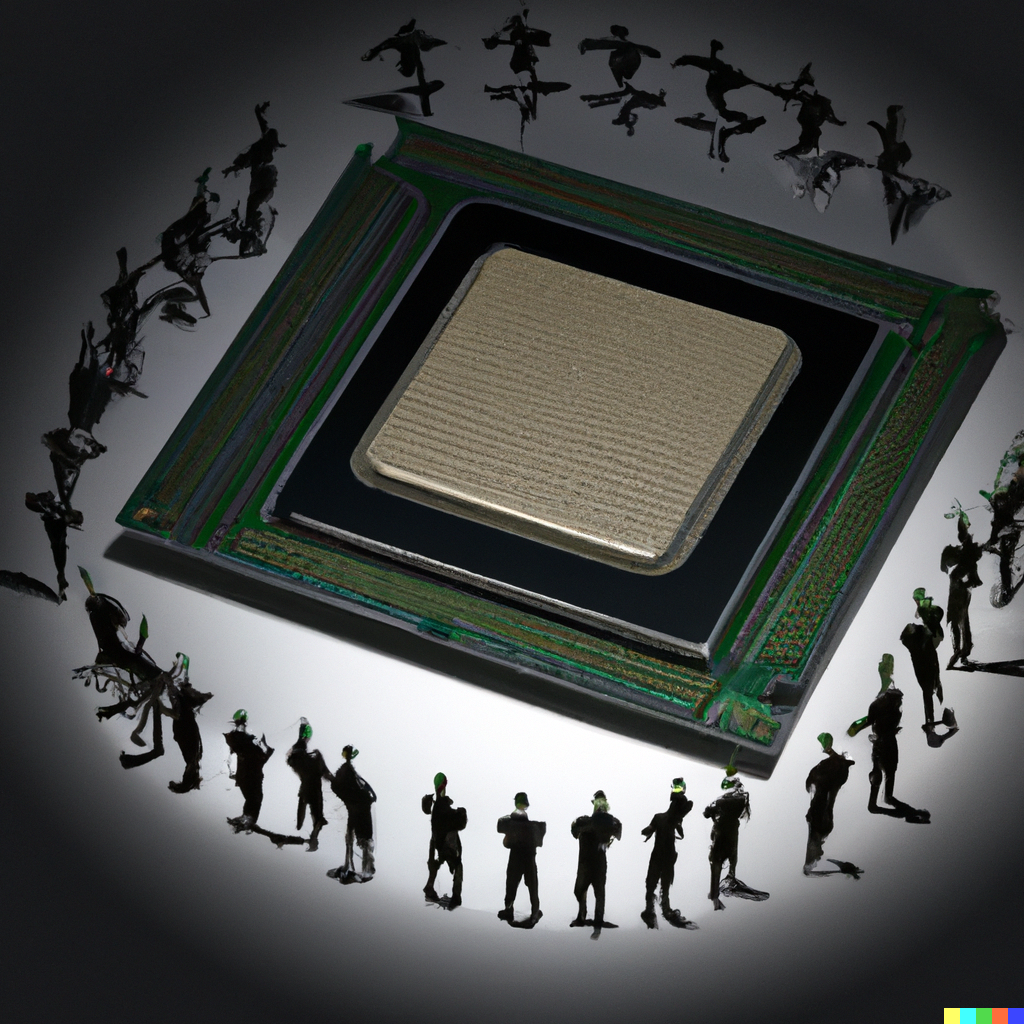
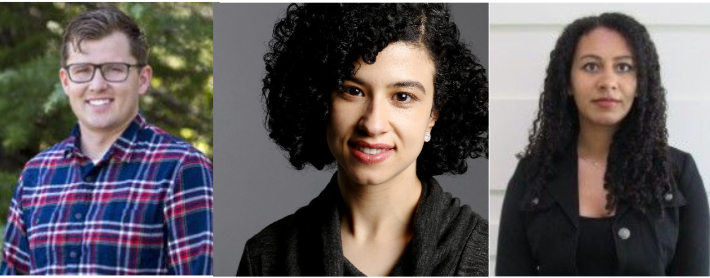
🔬 Research Summary by Andrew W. Reddie, Sarah Shoker, and Leah Walker.
Andrew W. Reddie is an Associate Research Professor at the University of California, Berkeley’s Goldman School of Public Policy, and Founder and Faculty Director of the Berkeley Risk and Security Lab.
Sarah Shoker is a Research Scientist at OpenAI where she leads the Geopolitics Team.
Leah P. Walker is the Assistant Director of the Berkeley Risk and Security Lab at the University of California, Berkeley.
[Original paper by Sarah Shoker, Andrew Reddie, Sarah Barrington, Ruby Booth, Miles Brundage, Husanjot Chahal, Michael Depp, Bill Drexel, Ritwik Gupta, Marina Favaro, Jake Hecla, Alan Hickey, Margarita Konaev, Kirthi Kumar, Nathan Lambert, Andrew Lohn, Cullen O’Keefe, Nazneen Rajani, Michael Sellitto, Robert Trager, Leah Walker, Alexa Wehsener, and Jessica Young]
Overview: As foundation AI models grow in capability, sophistication, and accuracy, and as those models become more broadly deployed, they can impact international security and strategic stability. In the worst cases, these models can introduce or outright cause accidents, inadvertent escalation, unintentional conflict, weapon proliferation, and interference with human diplomacy. To counter these risks, this report examines ideas for confidence-building measures (CBMs) for artificial intelligence technologies that build from a workshop on the same topic involving key stakeholders from industry, government, and academia.
Introduction
When asked to think about artificial intelligence in international security and defense spaces, people often think of the 1983 film WarGames, where a rogue supercomputer initiates a nuclear war. While a striking story, the reality of AI risks to international security is much more murky, and potential mitigation strategies for those risks are much less cut and dry than unplugging a computer (or teaching it the logic of mutually assured destruction).
This paper examines the need for confidence-building measures for foundational AI models, explores AI’s role as an “enabling technology,” and identifies confidence-building measures (CBMs) that limit foundation model risks to international security. This paper draws most of its conclusions from a workshop on the same topic, during which participants drew on personal experience, historical examples, and extrapolation of existing CBMs in other domains to identify potential, viable, foundation model-relevant CBMs.
The resulting six CBMs, to be implemented by AI companies and labs, government actors, and academic and civil society stakeholders, are as follows: (1) crisis hotlines; (2) incident sharing; (3) model, transparency, and system cards; (4) content provenance and watermarks; (5) collaborative red teaming and table-top exercises; (6) dataset and evaluation sharing.
Key Insights
Foundation AI Models and International Security Risks
This paper focuses on mitigating the security risks posed by foundation models applied to generative AI applications like large language models (for a further explanation, see Helen Toner, What Are Generative AI, Large Language Models, and Foundation Models?).
The breadth of potential applications of generative AI models, specifically, and foundational models more broadly, means that the risks they may pose to international security are many and varied. In particular, workshop participants were concerned about accidents, inadvertent conflict initiation and escalation, weapon proliferation and advancement, disinformation campaigns, and interference with human diplomacy. Generative AI models could initiate crises with an incident and, perhaps more likely, worsen ongoing crises that are initially unrelated to AI.
Confidence Building Measures, Past and Present
The paper advocates for confidence-building measures as a way to reduce these risks. Confidence-building measures (CBMs) are not new and have been applied to varied international security-related issues over the past century. Historical examples of confidence-building measures include the hotline between the United States and the Soviet Union, missile launch or military exercise notifications, and voluntary inspections of critical capabilities.
CBMs can be and are often introduced in “trustless” environments as a way to build confidence and predictability as to adversary motives. Generally, CBMs are non-binding and informal, making them easier to stand up than formal treaties or agreements. CBMs are also not limited to government participants: the private sector, civil society, and academic actors can often play a role in CBMs. Given that a substantial amount of AI research and development occurs outside of government, including these non-governmental stakeholders is essential.
Six CBMs to Mitigate Risk
The paper proposes six different confidence-building measures that can be directly applied to foundational models to mitigate international security risks, encourage strategic stability, and better prepare governments and private companies for engaging with an AI-integrated security environment.
- Crisis Hotlines
Crisis hotlines, like existing hotlines between states for deconfliction purposes, would serve as pre-established means of communication during crises. When properly set up, the use of hotlines can signal the importance of the incident while also ensuring that the right counterparts are connected as quickly as possible. However, workshop participants warned that for successful hotline use, state parties would likely have to share common values about the risk of foundation models and the value of communication in a crisis.
- Incident Sharing
Incident sharing of model failures, exploitations, and vulnerabilities between AI companies can raise awareness of frequent incidents and help others identify and respond to them more quickly.
Open-source AI incident-sharing initiatives already exist (see the AI Incident and the AI, Algorithmic, and Automation Incidents and Controversies (AIAAIC) databases). Still, open-source and non-public industry-sharing initiatives face challenges due to the lack of clarity on what constitutes an “AI incident” and concerns about respecting intellectual property rights and user privacy when sharing incident information.
- Model, Transparency, and System Cards
Model cards, transparency cards, and system cards can publicly disclose intended use cases, limitations, risks associated with human-machine interaction and overreliance, and results of red-teaming activities associated with model development. Card disclosure also provides useful information even if the model or other intellectual property is not made publicly available. Workshop participants noted that these cards should be readable, not overly technical, and easily accessible for policymakers and the general public.
- Content Provenance and Watermarks
Watermarks and other content provenance methods can be used to disclose and detect AI-generated or modified content and make that content more traceable. However, while interest in content provenance is high, current methods are not tamper-proof and remain focused on generated imagery. Scaling content provenance will require methods that expand beyond imagery and practices that encourage industry, government, and everyday users to adopt content provenance markers.
- Collaborative Red Teaming and Table-Top Exercises
Collaborative red teaming between public, private, civil society, and academic partners can serve to expose participants to the limitations and flaws in models, identify vulnerabilities and inaccuracies, and test models for resilience against misuse or harmful use. Tabletop exercises with key stakeholders can simulate incidents, allowing participants to practice coordination and incident response in a sandbox before doing so in the real world. Tabletop exercises between governments also clarify intentions and surface national sensitivities that may prove helpful in navigating future crises.
- Dataset and Evaluation Sharing
The last recommended CBM in this paper encourages sharing datasets that focus on identifying and addressing safety concerns in AI models and products. Collaborating on “refusals,” or instances when an AI system refuses to generate potentially harmful content, could lift the floor of safety in the AI industry and be especially helpful to smaller labs and companies unable to dedicate significant resources to red teaming.
Between the lines
While this paper is not the first to discuss confidence-building measures (e.g., Michael Horowitz and Paul Scharre’s “AI and International Stability: Risks and Confidence-Building Measures”), we hope that it expands the understanding of international security risks from those introduced by AI integration in military systems to those that emerge from the broad adoption of AI across a variety of civilian and military applications. We also hope that this paper serves to hone potential CBMs that are well-suited to foundation models rather than simply propose general areas of collaboration and confidence building across AI technologies broadly.
For each of the six CBMs identified in this paper, there is still the need to chart implementation pathways. A hotline does not appear overnight, nor does incident sharing happen without careful preparation. Roadmaps for these CBMs should include timelines for adoption, distinctions between the public and private sector roles, identification of potential governance regimes, and clear delegation of authority to the personnel tasked with maintaining them. Separately, we welcome research into potential CBMs for other AI model types, given this paper’s focus on foundation models and generative AI applications.