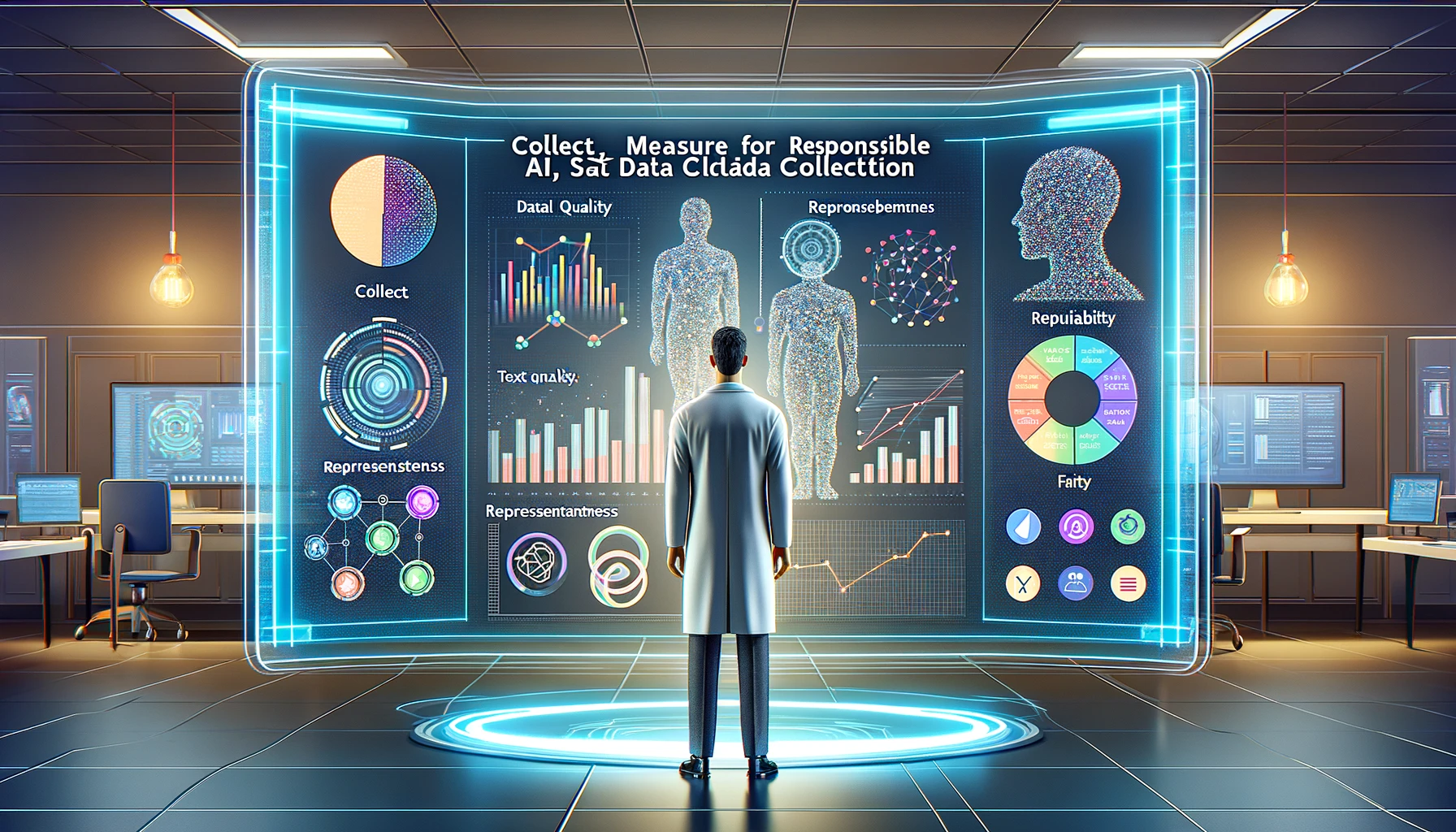
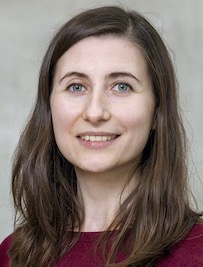
🔬 Research Summary by Oana Inel, a Postdoctoral Researcher at the University of Zurich, where she is working on responsible and reliable use of data and investigating the use of explanations to provide transparency for decision-support systems and foster reflective thinking in people.
[Original paper by Oana Inel, Tim Draws, and Lora Aroyo]
Overview: Recent research has shown that typical one-off data collection practices, dataset reuse, poor dataset quality, or representativeness could lead to unfair, biased, or inaccurate outcomes. Data collection for AI should be performed responsibly, where the data quality is thoroughly scrutinized and measured through a systematic set of appropriate metrics. In our paper, we propose a Responsible AI (RAI) methodology designed to guide the data collection with a set of metrics for an iterative, in-depth analysis of the factors influencing the quality and reliability of the generated data.
Introduction
Despite developing numerous toolkits and checklists to assess the quality of AI models and human-generated datasets, the research landscape still needs a unified framework for cross-dataset comparison and measurement of dataset stability for repeated data collections. Our approach complements existing research by proposing an iterative metrics-based methodology that enables a comprehensive analysis of data collections by systematically applying reliability and reproducibility measurements.
The reliability metrics are applied to a single data collection and focus on understanding the raters. We propose that data collection campaigns be repeated under similar or different conditions. This allows us to study in-depth the reproducibility of the datasets and their stability under various conditions using a set of reproducibility metrics. The overall methodology is designed to integrate responsible AI practices into data collection for AI and allow data practitioners to explore factors influencing reliability and quality, ensuring transparent and responsible data collection practices. We found that our systematic set of metrics allows us to draw insights into the human and task-dependent factors that influence the quality of AI datasets. They also provide the necessary input for a dataset scorecard, allowing for thorough and systematic evaluation of data collection experiments.
Key Insights
Reliability and Reproducibility Metrics for Responsible Data Collection
Our proposed methodology brings together, systematically, a set of measurements typically performed ad hoc. However, observing their interaction allows data practitioners to provide a holistic picture of the data quality produced by these studies. The chosen metrics provide input for a scorecard, allowing for thorough and systematic evaluation and comparison of different data collection experiments.
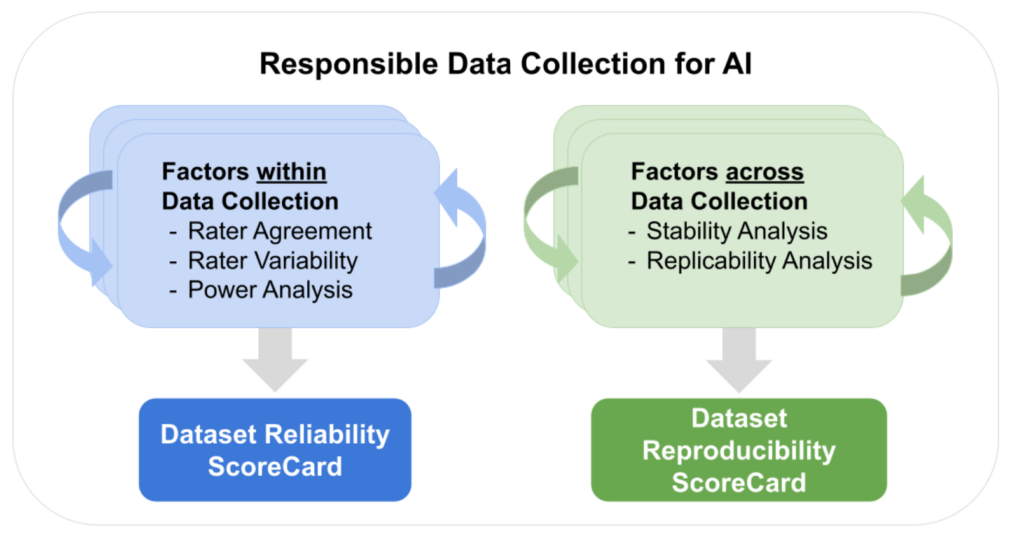
We address the reliability of human annotations by looking at raters’ agreement (i.e., measuring their inter-rater reliability), raters’ variability (i.e., measuring the variability in raters’ answers distribution), and power analysis (i.e., determining the sufficient number of raters for each task). These analyses equip us with fundamental observations and findings for characterizing annotations’ quality and reliability.
To investigate how rater populations influence the reliability of the annotation results, we propose repeating the annotations at different time intervals and in different settings, thus identifying the factors influencing their reliability. However, we need to use the aforementioned reliability measures to perform a proper comparison of the collected annotations. For instance, high raters agreement values in several repetitions indicate highly homogeneous raters’ populations within each repetition, but it does not necessarily mean that the experiments are highly reproducible. For this, we perform two additional measurements: 1) stability analysis (i.e., measuring the degree of association of the aggregated raters’ scores across two data collection repetitions) and 2) replicability similarity analysis (i.e., measuring the degree of agreement between two rater pools, making two data annotation tasks comparable) to understand how much variability the raters bring and how much we can generalize the results.
In sum, our proposed methodology provides a step-wise approach as a guide for practitioners to explore factors that influence or impact the reliability and quality of their collected data. Ultimately, the proposed reliability and reproducibility scorecards and analyses allow for more transparent and responsible data collection practices. This leads to identifying factors influencing quality and reliability, thoroughly measuring dataset stability over time or in different conditions, and allowing for dataset comparison.
What are the factors influencing the quality of data collection?
We validated our methodology on nine existing data collections repeated at different time intervals with similar or different rater qualifications. The annotation tasks span different degrees of subjectivity, data modalities (text and videos), and data sources (Twitter, search results, product reviews, YouTube videos). By following our proposed methodology, we were able to identify the following factors that influence the overall quality of data collection:
- Intrinsic task subjectivity: This is the case of tasks with low observed inter-rater reliability in each repetition but high stability and high replicability similarity across repetitions. Such scorecard interpretations indicate that raters are similarly consistent within each repetition and across repetitions and that the disagreement indicated by the low IRR scores is, in fact, intrinsic to the subjective nature of the task.
- Region-specific and time-sensitive annotations: High variability for certain annotated items across different repetitions of a data collection indicates that data collection practices are affected by temporal, familiarity, and regional aspects. In such cases, our analysis shows consistently low stability and replicability similarity. This has serious implications for when data collections are reused, as certain annotations may become obsolete or change in interpretation over time. Furthermore, we posit that diverse raters should not be expected to produce a coherent view of the annotations. We advise repeating the data collection by creating dedicated pools of raters with similar demographic characteristics and comparing their results.
- Ambiguity of annotation categories: High variability for certain annotated items and power analysis indicating that even a very high number of raters (around 90) can exhibit high levels of consistent disagreement is typically caused by the subjectivity of the task. In this case, we recommend optimizing the task design to decrease additional ambiguity in the annotation categories. Lower inter-rater reliability (IRR) values for certain annotation categories indicate that some categories may not be as clear as others or are only seldom applicable. This suggests that careful attention should be given to the annotation task’s design, instructions, and possible answer categories. Furthermore, the high number of raters needed to obtain stable results indicates that the task might benefit from a more thorough selection of raters and training sessions.
Between the lines
Our proposed methodology for responsible data collection does not pose any requirements on how data is structured or formatted. What we propose does, however, affect the current practice and assumes a significant adaptation to using reliability and reproducibility metrics. We recommend the following:
- Systematic piloting: The proposed methodology is primarily suitable as an investigative pilot of data annotation studies. Pilot experiments could identify factors that influence the data collection and be ultimately mitigated for large-scale data collection.
- Capture raters, task, and dataset characteristics: Borrow guidelines for reporting human-centric studies from psychology, medicine, and HCI, where human stances, opinions, and other meaningful characteristics are thoroughly recorded. This would facilitate informed decisions on the proper process of collecting raters’ annotations and possible reuse.
- Cognitive biases assessment: We recommend using existing checklists to identify and subsequently measure, mitigate, and document cognitive biases that may present an issue in the data collection tasks between each data collection iteration. This allows for proper mitigation of biases.
- Provenance for data collection: Data documentation and maintenance approaches should thoroughly record provenance, including quality scorecards. This would alleviate issues regarding data handling, reuse or modifications of annotation tasks, and platform selection.