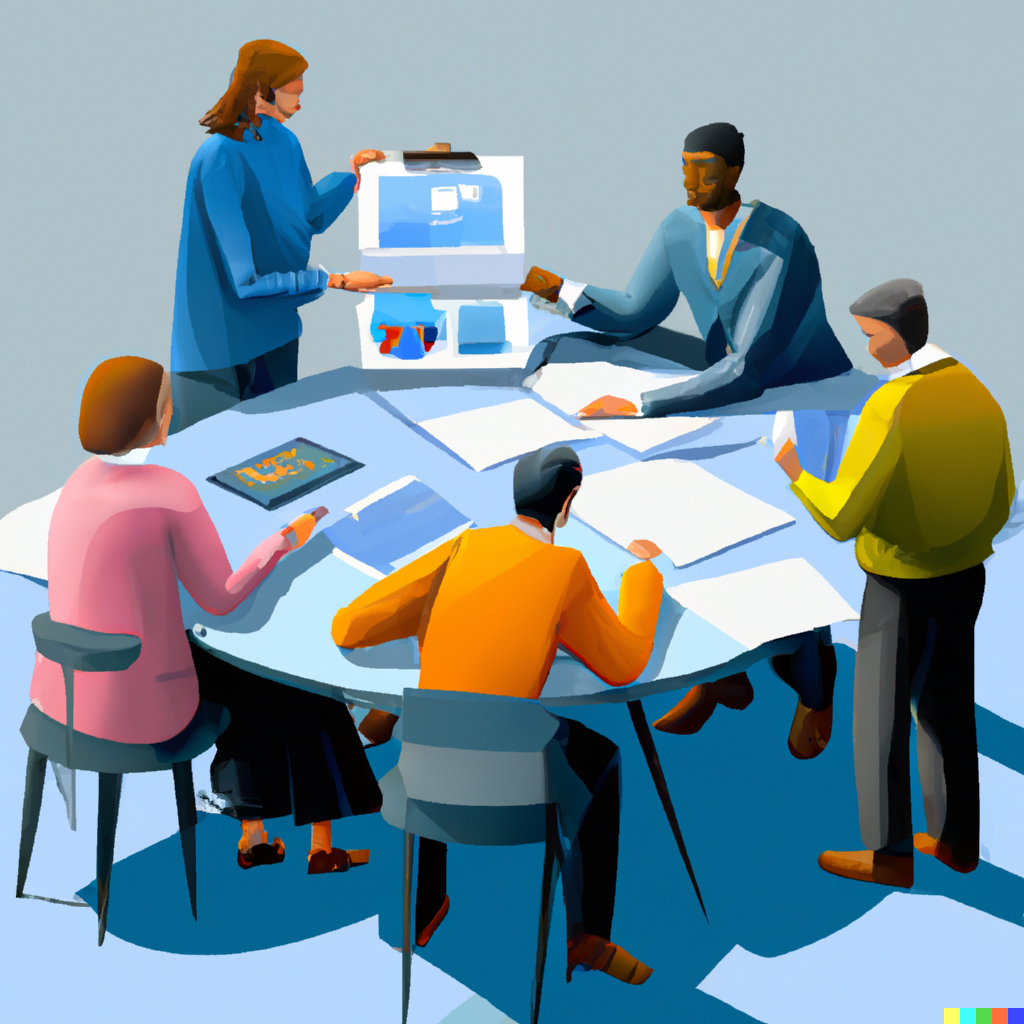
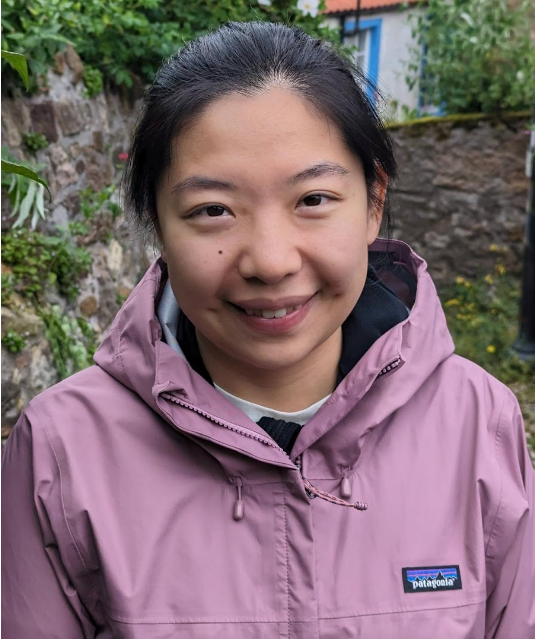
🔬 Research Summary by Ding Wang, a senior researcher from the Responsible AI Group in Google Research, specializing in responsible data practices with a specific focus on accounting for the human experience and perspective in data production.
[Original paper by Shivani Kapania, Alex S. Taylor, and Ding Wang]
Overview: Diversity in datasets is a key component to building responsible AI/ML. Despite this recognition, we know little about the diversity among the annotators involved in data production. This paper takes a justice-oriented approach to investigate how AI/ML practitioners envision the diversity of data annotators both conceptually and practically. Drawing upon the feminist critique on objectivity, we explore alternative ways of accounting for annotator subjectivity and diversity in data practices.
Introduction
This paper employed mixed-methods research through a series of interviews and surveys with machine learning practitioners from academia and industry we set out to understand whether and how these practitioners approach diversity embedded in data annotators that work on their data. This work aimed to contribute to the ongoing discussion on AI diversity (e.g., mitigating AI bias on disability) as we recognize that diversity in AI systems is more than just what is encoded in the data but also who might encode them. While practitioners described nuanced understandings of annotator diversity, they rarely designed dataset production to account for diversity in the annotation process. The lack of action was explained through operational barriers: from the lack of visibility in the annotator hiring process to the conceptual difficulty in incorporating worker diversity. We argue that such operational barriers and the widespread resistance to accommodating annotator diversity surface a prevailing logic in data practices—where neutrality, objectivity, and ‘representationalist thinking’ dominate. By understanding this logic as part of a regime of existence, we explore alternative ways of accounting for annotator subjectivity and diversity in data practices.
Key Insights
A growing effort has been to investigate data diversity and its implications for machine learning development. Our research contributes to this ongoing discussion on data diversity with a specific focus on the diversity of the ones who work to annotate datasets. Surveying 44 practitioners and conducting 16 interviews, we found that while practitioners understood the subjectivity of annotators, diversity considerations were often neglected in practical tasks. The focus was on reducing costs and complexity, leaving diversity as a low-priority concern. We analyzed dataset development and AI/ML model building and found an “algorithmic idealism” that assumes neutrality in data representation. To address this, we propose a shift in epistemic orientation, emphasizing justice-oriented and intersectional perspectives. The paper offers an empirical account of annotator diversity, examining underlying logic and suggesting a deeper exploration of diversity in data practices.
Critical and analytic orientation
This paper adopts a critical orientation, drawing on the concept of “representation” and influenced by scholars like Hacking, Barad, Goodwin, and Haraway. Representationalist thinking assumes objective observation and representation of phenomena applied across contexts. Data practices, especially in dataset annotation, reflect this thinking, treating data as representations of an objective world. The paper explores how this shapes ML practice and its implications for diversity and subjectivity.
Teil’s concept of “regimes of existence” is also cited, where subjectivity is marginalized in favor of mechanized quality measures in wine-making. This notion extends to data practices, where diversity and subjectivities may be overlooked for practical goals. Recognizing objectivity as non-neutral opens doors for alternative approaches to diversity and data practices.
Furthermore, the paper draws inspiration from justice-oriented feminist and intersectional theory, particularly intersectionality. Three approaches—inter-categorical complexity, intra-categorical complexity, and anti-categorical complexity—are employed to address the complexity of intersectionality. This critical framework invites examination and shifts in epistemic orientation.
Ultimately, we advocate for criticality, challenging prevailing logic, and exploring alternative imaginings to promote equity and inclusivity in data practices.
Approaches to Annotator Diversity
In the data annotation workflow, practitioners identify data needs, select annotation platforms, and design tasks with iterative improvements. Internal infrastructures were preferred over external marketplaces. Language-based ML tasks were prominent, with various applications like semantic parsing and translation. Other projects included anomaly detection and image segmentation.
Participants in the study had varied perspectives on annotator diversity; some considered it irrelevant, while others made partial efforts to accommodate it. Many practitioners acknowledged the role of annotator subjectivities but prioritized achieving a quality threshold. Although most respondents recognized diversity’s influence on dataset quality, few utilized diverse factors in the recruitment process. Interviews revealed a contrast between the concept of annotator diversity and its implementation. Annotators were often chosen based on proxies like language or location, while experiential knowledge and expertise were rarely considered. Participants tended to view diversity through categories and metrics rather than lived experiences and expertise.
The pursuit of objective annotations
Practitioners often deprioritized annotator diversity, justifying it based on the belief that certain annotation tasks were objective, with definitive answers. Tasks with ground truth data or requiring subject expertise were considered less relevant for diversity. Quality checks and training sessions ensured objective annotations, breaking tasks into simple, repeatable sub-tasks to standardize work. Practitioners saw objectivity as a trainable skill, guiding annotators to make unbiased judgments following explicit instructions.
The attempt to remove bias
Practitioners justified avoiding complexity by prioritizing useful and testable AI/ML outcomes. They designed workflows for consistent evaluation across data, tasks, and annotators, limiting ambiguity to assess AI/ML model performance. Annotator subjectivities were often framed as bias, addressed through interventions to reduce disagreement and ensure uniformity. Data quality and disagreement were attributed to flaws in guidelines, task ambiguity, or annotator attributes like knowledge and motivation.
The quest for neutral representation
Only a few participants actively incorporated annotator diversity in data production and model building, recruiting annotators from diverse backgrounds. They aimed to capture various perspectives to achieve neutral AI/ML models. However, challenges in determining relevant social categories and justifying the resources needed hindered these efforts. The current data practices prioritized consensus over individual diversity, limiting the exploration of diverse annotator perspectives and resulting in data that lacks true diversity.
Barriers to Incorporating Diversity
Lack of information about annotators
Many practitioners lacked specific knowledge about annotators working on their tasks, relying on third-party platforms for recruitment. Limited access to annotator information due to legal constraints and project timelines hindered efforts to understand their backgrounds. Privacy concerns made collecting sensitive information challenging. Legal, ethical, and corporate considerations created obstacles to recruiting diverse annotators, making it difficult to incorporate diversity effectively. Protecting the annotator’s well-being was also crucial, especially in tasks involving harmful content. These complex structures posed challenges to achieving true annotator diversity.
Separation of operations
Most practitioners relied on third-party platforms for data annotation, introducing communication barriers and a disconnect between practitioners and annotators. Challenges in building trust with annotators and limited power to address platform issues hindered direct communication. Geographical distance and time differences amplified the separation between practitioners and annotators. Tight turnaround times and business pressures led to practitioners independently resolving inconsistencies in data labels. Due to limited information and communication channels, annotators were often seen as interchangeable workers and only a few practitioners considered the impact of annotator identities and the importance of diversity.
Competing priorities in machine learning development
The ML workflow’s status quo poses challenges to considering annotator diversity. Short-term development timelines prioritize curating larger datasets and building better-performing models over diverse annotators. Emerging and niche application areas prioritize reaching an MVP before addressing annotator diversity. Data annotation is integrated into ML pipelines designed for definitive answers, conflicting with diverse subjectivities. Reducing annotation costs and competition shape annotator recruitment. Setting up annotation pipelines is effort-intensive and costly, making diversity-related concerns secondary to building models quickly and cost-effectively.
Conclusion and implications
This paper argues that practitioner views of annotator diversity are influenced by representationalist thinking, seeking objectivity and neutrality. Annotators are treated as apparatuses for achieving neutral representations of the world. Practices minimize annotators’ subjectivities and reduce diversity to stable categories. We draw parallels with Teil’s analysis of terroir in wine-making, where subjectivities are marginalized in favor of objectivity. We call on practitioners to be accountable for marginalized perspectives and challenge the prevailing thinking. We can move towards a more inclusive and diverse approach to data annotation by rethinking ground truth, bias, and diversity.
Rethinking Ground Truth
The findings show how diversity among annotators is deprioritized in favor of practical considerations in building AI/ML models. The convergence-driven machine learning workflow minimizes the importance of annotators’ subjectivities. Disagreements among annotators are often seen as undesirable and resolved by experts, limiting the impact of diversity. To manage annotator subjectivities, practitioners should acknowledge data production as an interpretive task and consider approaches that preserve minority perspectives. Tools and processes that support diverse annotator positions can foster a pluralistic approach to data annotation.
Rethinking Bias
Our research highlights the influence of individual annotator identities on the annotation task and calls for a critical examination of the framing of “bias” in data annotation. The pursuit of annotator diversity should not solely be for better-performing models but also for justice-oriented goals and broadening ways of knowing. Documentation artifacts can promote transparency but may not effectively address representationalist thinking. Instead, we propose participatory and collaborative approaches to data annotation, encouraging practitioners to explore co-created labeling setups with annotators.
Rethinking Diversity
Our research highlights the limitations of representationalist thinking in treating annotator diversity and calls for a justice-oriented approach inspired by intersectionality. The current pursuit of diversity for representativeness often relies on static social categories and overlooks the intersections of identities and experiences. To address this, we propose recommendations for exploratory modes of engaging with intersectionality, such as tools for visualizing different annotator distributions and exploring the impact of weighted marginalized identities on models. We also encourage practitioners to consider neglected points of intersection and resist predefined categories by examining lived experiences and organizational contexts.
Between the lines
Despite the work that came out a year ago, the relevance of it, instead of decreasing, has increased on the backdrop of the release of large language models such as ChatGPT and Bard. Several articles discuss the working conditions of the annotators, and their experience came out this year. We have a fuller picture of the lives of annotators across the world. Yet, changes in how we as an industry engage with the annotators, their work, their perspectives, and their aspirations have yet to be followed up.