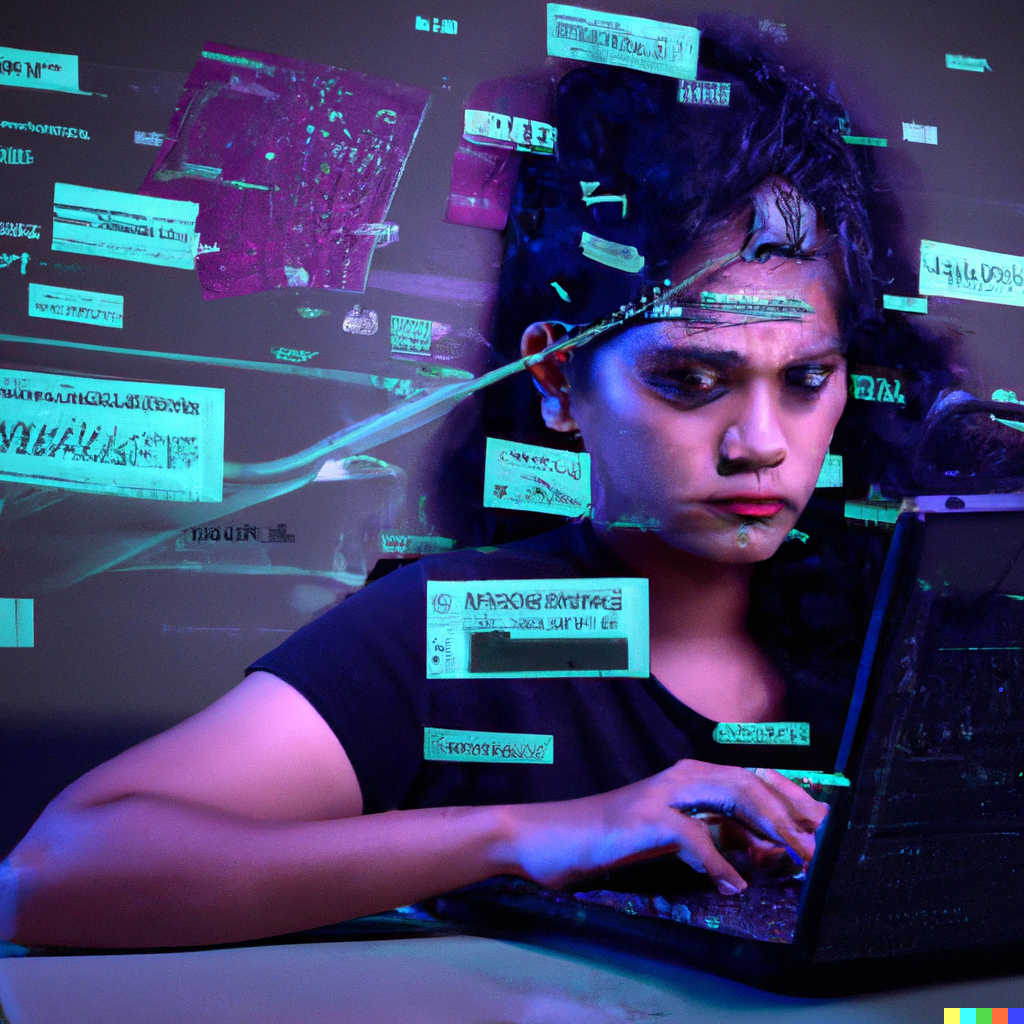
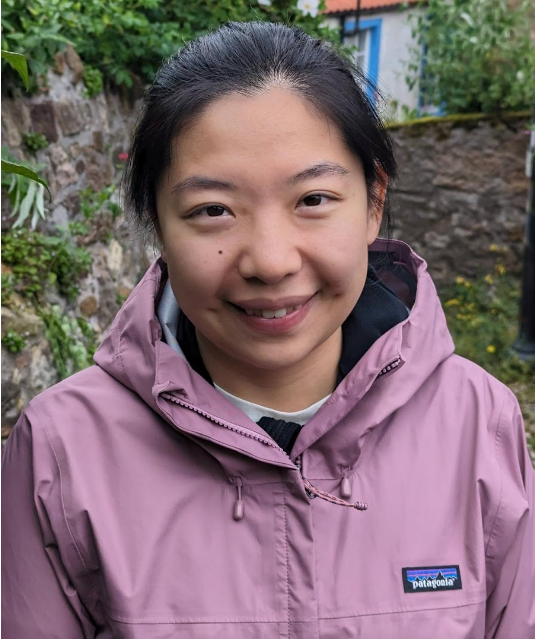
🔬 Research Summary by Ding Wang, a senior researcher from the Responsible AI Group in Google Research, specializing in responsible data practices with a specific focus on accounting for the human experience and perspective in data production.
[Original paper by Ding Wang, Shantanu Prabhat, and Nithya Sambasivan]
Overview: This paper delves into the crucial role of annotators in developing AI systems, exploring their perspectives, aspirations, and ethical considerations surrounding their work. It offers valuable insights into the human element within AI and the impact annotators have on shaping the future of artificial intelligence.
Introduction
This research provides valuable insights into the experiences of data annotators in India, who have played a significant role in developing AI systems worldwide. The study involved interviews with 25 data annotators working for third-party annotation companies. It sheds light on their daily work and provides a glimpse into their aspirations. Despite holding undergraduate degrees in STEM subjects, the annotators found themselves attracted to data annotation as part of their involvement in AI development, even though their initial dreams may have been to become machine learning engineers. The research highlights that despite transitioning from platform work to organized employment, the job still carries a sense of precarity.
Key Insights
While data annotation was historically done on crowd-work platforms, there has been a rise in private annotation firms employing full-time workers. These firms provide various services beyond annotation, such as project management and quality control. However, the human labor involved in data annotation remains under-recognized compared to the market value of the annotation. The paper focuses on the work practices and experiences of data annotators in India, revealing challenges such as work pressure, lack of career progression, and precarity despite professionalization. The findings contribute to understanding the organization of data annotation work and its implications for stakeholders, particularly the annotators.
The paper addresses the significance of crowd-sourcing platforms employing numerous workers for essential AI model training tasks. It highlights the challenges related to enforcing norms and cultural sensitivity in annotation work, emphasizing the hidden nature of this labor. Smaller platforms prioritize worker training and local expertise, favoring project-oriented contractual hiring and individual expertise. The evolving landscape of annotation work intertwines with the gig economy. Despite efforts to document sociological aspects of data and promote ethical AI practices, the focus tends to neglect the practice of data annotation and the role of data workers. However, recent regulations in China acknowledge the importance of protecting workers’ rights. The paper asserts that data annotation practices should be integral to ethical and responsible AI discussions.
Becoming an annotator
The recruitment process for annotators in the study revealed a common practice of requiring high educational qualifications, such as undergraduate degrees in technology and engineering. Previous experience in annotation was not necessary and was even seen as a disadvantage, as it would lead to higher salary expectations. Referrals played a significant role in finding employment, with friends, classmates, or alumni referring nearly half of the annotators. The rapid growth of annotation companies and the promise of a bright future in the technology industry, particularly in autonomous vehicles, attracted participants to become annotators. Job advertisements portrayed annotation as a well-paid and prestigious part of the AI industry, reinforcing the AI dream narrative. The interview process for annotation positions included technical assessments, adding complexity and a sense of technicality to the role.
Training for annotators involved orientation training and pre-project training. Orientation training focused on familiarizing annotators with tools and processes but often lacked the connection between annotation and AI. Pre-project training provided specific instructions on datasets and guidelines. However, tight deadlines sometimes led to skipping pre-project training, impacting knowledge transfer and annotation quality.
The emphasis on client satisfaction and fast delivery overshadowed annotators’ training needs and interests. Data quality was primarily measured by accuracy rates, prioritizing client requests. The annotators discovered shortcuts and techniques through experience, improving productivity but not formally included in the training.
Overall, the recruitment, training, and work conditions reflected the rapid growth and demand in the annotation industry, highlighting the importance of education, referrals, and better alignment between training and annotators’ needs.
Being an annotator
Annotators worked long hours, often exceeding official reports, without compensation for the extra work. They typically started the day with a status check led by team or project leads, reviewing pending tasks and receiving data to label. They used company-issued laptops with limited access to relevant websites and software. Communication tools like Microsoft Teams, Google Chat, and WhatsApp (in some cases) were essential for work-related queries. Access to unauthorized websites and software was blocked. Annotators had experience with various annotation tools, including in-house and open-source ones.
Target-setting for annotators was determined by team leads or project managers based on experience or average completion rates. Targets could increase over time, and annotators were expected to meet them without negotiation. Analysts and managers conducted quality control checks to ensure high-quality data delivery. Accuracy rates, often around 98% or aiming for zero errors, were monitored through automated and manual checks. Annotators reported tool issues through separate channels, with resolutions and client communications determined by company hierarchy.
The data annotation process extends beyond technical aspects, involving organizational structure and power dynamics. Annotation companies prioritize high-quality data delivery at a low cost, with strict work quality monitoring. Annotators navigate these dynamics while striving to meet targets and deliver accurate annotations.
An annotator’s aspiration
Data annotation is often seen as a stepping stone to a career in AI and ML. However, the skills and experiences gained in annotation do not necessarily translate to technical roles. The concept of expertise in annotation is unclear, and breaking into more technical positions can be challenging. Promotion opportunities within annotation are limited, and the retention rate is low, with annotators typically staying for 12-18 months. The compensation system does not incentivize annotators to stay; job stability is uncertain. The pandemic has further exacerbated job insecurity, with many annotators experiencing job losses. Despite the challenges, annotators take pride in their contribution to AI and ML, recognizing the importance of data annotation in these fields. However, the current state of data annotation highlights the irony of overqualified individuals performing repetitive tasks to support the development of AI technologies.
In conclusion, Whose AI Dream?
Data annotation is a crucial process performed by full-time annotators within well-structured working environments. While the third-party annotation industry has grown alongside AI and ML systems, our research shows that individual annotators have not reaped their benefits. Annotation companies provide defined roles and hierarchies to handle complex tasks and meet client expectations, yet this rigid structure limits data interpretation and stifles annotators’ skills and perspectives. Performance metrics narrowly define data quality, overlooking annotators’ unique contributions. Annotators seldom question power dynamics, accepting predetermined notions of success. To support their aspirations, stakeholders must understand the annotation’s context and consider design implications. Our study reveals limited stability and career progression within annotation, hindering annotators from pursuing technical roles. Recognizing expertise beyond educational credentials is crucial. Articulating fundamental annotation skills and providing appropriate training can enhance annotators’ employability. Promoting career growth within the industry requires shared knowledge, ML/AI systems exposure, and ethical practices. Regulatory discussions address fair working conditions while documenting data labor practices informs policy improvements. Annotators’ expertise, adaptability, and responsiveness should be valued, positioning them as agile experts. Collaborative efforts among researchers, practitioners, legal experts, and policymakers are vital for systemic changes prioritizing annotators’ well-being and career development in the AI and ML field.
Between the lines
Despite the work that came out a year ago, the relevance of it, instead of decreasing, has increased on the backdrop of the release of large language models such as ChatGPT and Bard. Several articles discuss the working conditions of the annotators, and their experience came out this year. We have a fuller picture of the lives of annotators across the world. Yet, changes in how we as an industry engage with the annotators, their work, their perspectives, and their aspirations have yet to be followed up.