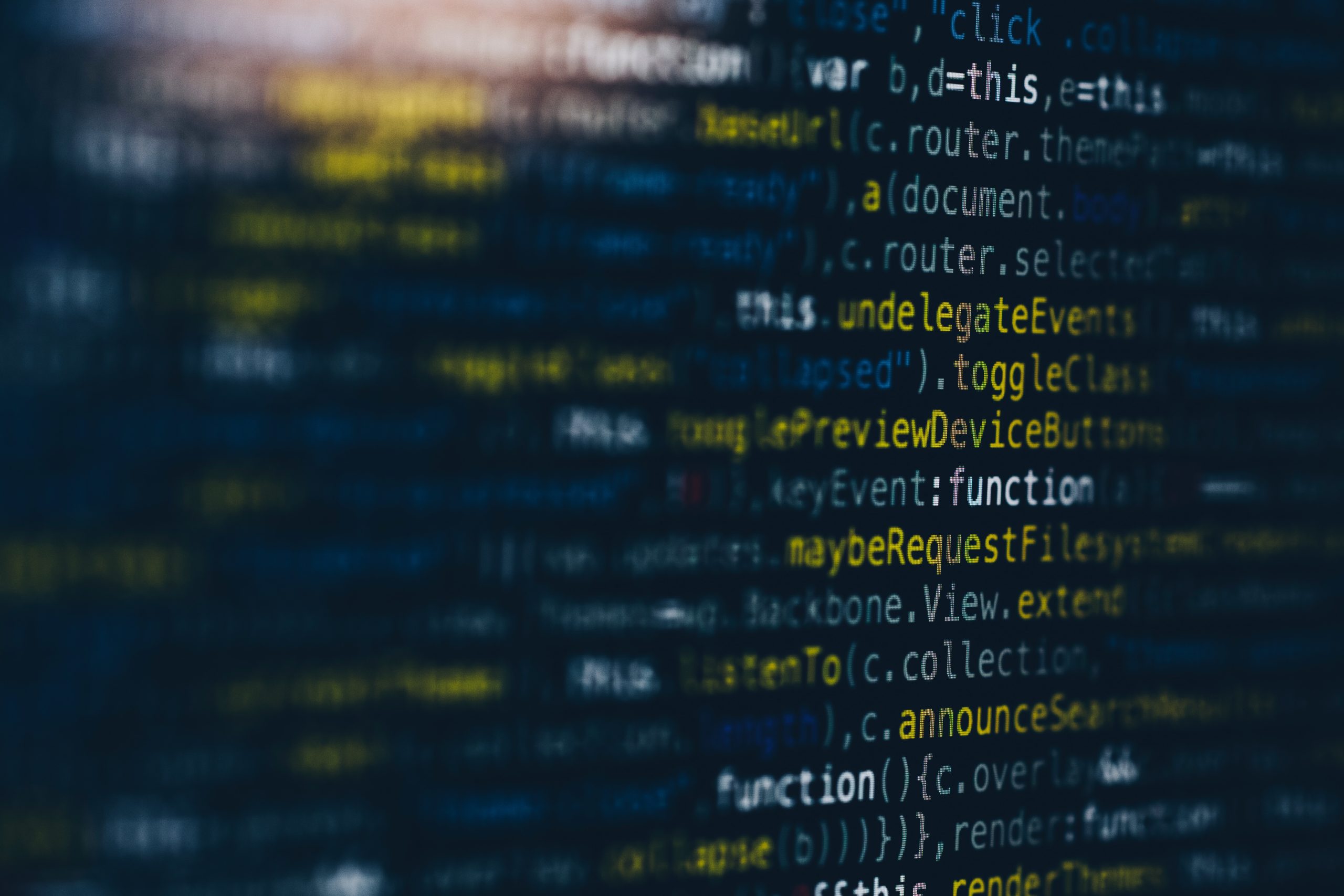
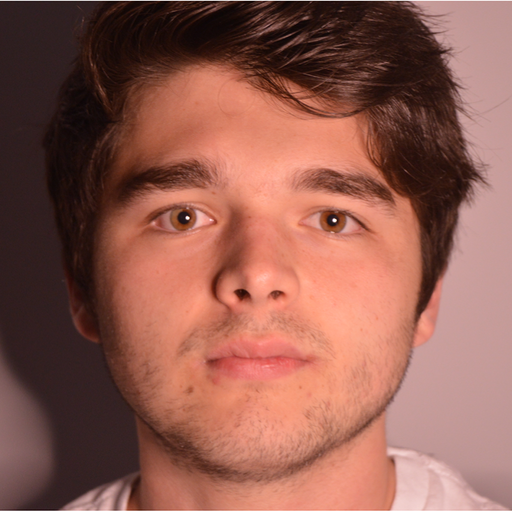
🔬 Talk summary by Connor Wright, our Partnerships Manager.
Overview: In a talk given to the Montreal Integrity Network, Connor Wright (Partnerships Manager) introduces the field of AI Ethics. From an AI demystifier to a facial recognition technology use case, AI is seen as a sword that we should wield, but only with proper training.
Introduction
In a talk given to the Montreal Integrity Network, I set about offering an overview of the AI Ethics field and the issues it contains. Stretching from defining AI, to doughnuts, to facial recognition technology (FRT) and current laws, I aimed to provide a fruitful introduction to the field. Like any good presentation, it all starts with some definitions.
Key Insights
An AI demystifier
I mentioned how AI is not just limited to the stereotypical view of killer terminator robots or anthropomorphic AI. Instead, depending on how you define AI (which is highly fluid), you can possess the technology in your very hand.
So, I wanted to gauge what the audience thought of when they heard the words “Artificial Intelligence”. The following word cloud resulted:
With some central themes of my talk emerging, I set about defining what AI is.
How can AI learn?
Machine learning
As a subset of AI, machine learning provides the more technical explanation of how an AI makes its predictions. Here, machine learning describes AI as algorithms, whereby a human sets the parameters and desirable features of the data that it will receive as input. For example, let’s say we’re designing an AI with the goal of identifying pictures of cats. I would set the parameters (like the ‘rules of a game’) for the AI to act towards this goal by identifying the desirable features (such as whiskers). The algorithm will be able to improve its predictions with the more input data (photos of cats) that I provide it.
Deep learning
As a subset of machine learning, deep learning algorithms set the desirable features themselves, unlike machine learning itself. Requiring large data sets and computing power, the algorithm goes about learning which features of the data it receives are conducive to achieving its goal.
For example, if we started a doughnut-ranking business, we could set the deep learning algorithm to try and discover the most popular Krispy Kreme doughnut in the world. The data is supplied to it would contain every different type of doughnut sold in the world, and it would then set about identifying which features help it best to come to a decision. In this way, it would start eliminating all the doughnuts that Krispy Kreme doesn’t supply, considering that popularity means how many are sold, etc.
Machine learning vs deep learningIn this way, we can come to a critical difference between machine learning and deep learning. Here, machine learning requires ‘structured data’ (data with labels set for the algorithm to learn and then use to identify objects pertaining to its goal). On the other hand, deep learning uses unstructured data (data without labels, which it then creates to identify the objects conducive to its goal). Here, machine learning needs to have it pointed out that cats have whiskers to recognize images of them, whereas deep learning creates its own label for whiskers to identify images as cats.
AI in business
Chatbots
I touched upon how chatbots (through their use of natural language processing which allows them to recognise words) are a straightforward way to leverage AI technology in business. They help free up resources to be dedicated elsewhere and can act as part of a 24/7 customer response service.
I then tackled the question of what makes a good chatbot? Part of the answer process is the right balance between anthropomorphism and truth. Here, a more ‘natural’ sounding conversation will help keep customers engaged (such as asking what the customer’s name is), but this should not be taken to the point where the chatbot is confused with being a human. Furthermore, a decent knowledge of colloquialisms and the ability to adapt to typos are also key. At times, these two aspects may flumox the chatbot and that’s alright, so long as a human agent can be introduced into the conversation quickly.
The hiring process
AI can also be used in business to help streamline the hiring process. Top businesses such as Hilton use AI to help deal with the thousands of applications they receive a day. For example, Hilton uses the end to end AI recruitment software of AllyO to help schedule final interview calls for call centre applications. AI in this capacity can again help dedicate finite resources to other tasks which require a more personal touch, instead of having to manually send thousands of emails and schedule thousands of calls. However, this is not without its problems.
Issues and concerns
Problems with learning
An unfortunately popular avenue for problems within AI is contained within the learning process of AI itself. I mentioned how with machine learning and deep learning, the sourcing of data is vital. Large data sets are required in order to better train the models being designed and create a more accurate product at the end of it. However, how this data is sourced can be problematic, with the consent of the ‘producer’ of the data (such as a Facebook user) not always being achieved.
AI bias
I took AI Bias to be the systematic prioritization of arbitrary characteristics in a model that leads to unfair outcomes. An AI is then biased if it makes decisions that favour or penalize certain groups for reasons that are not valid criteria for decision-making or for factors that are spuriously correlated with the outcome. For example, within predictive policing, an unrepresentative data set fed into the algorithm (such as featuring more criminal records of one race over another) would be more likely to predict a disproportionately higher likelihood to commit a crime for some races over others.
AI fairness
I commented how algorithmic fairness is the principle that the outputs of an AI system should be uncorrelated with certain characteristics such as gender, race, or sexuality. There are many possible ways to consider a model fair. Common approaches include equal false positives across sensitive features, equal false negatives across sensitive characteristics, or minimising “worst group error”, the algorithm’s number of mistakes on the least represented group. Being able to best evaluate an AI’s fairness is to know where and how it went wrong, preventing the proliferation of “black box” algorithms.
Facial recognition technology (FRT) use case
What are the kinds of ethical issues involved in FRT? I was able to mention the following:
FRT needs specifics
FRT does not like any “noise” present when it’s trying to study photos (such as loads of different objects in the background). This doesn’t bode too well for society, which is a busy place and isn’t always posing for a clear photo.
FRT is liable to bias
What you give FRT, you get out. As with all AI, the dataset given to the algorithm or software is what it feeds off and learns from (like a newborn baby). For example, if we taught a newborn that the first letter of the alphabet is Z, it will continue to treat it as such. Likewise, if we present the FRT with a dataset that only comprises of white male faces, it will only be able to accurately identify white male faces. This could be a result of human error, or just a lack of awareness of what the database consists of.
The level of trust
It has become a ‘malpractice’ to question decisions made by technology. The technological mindset that has shaped our searches for solutions to problems, I argued, made it almost frowned upon to question the results of the technology. Technology is seen as something that, and with proof, is more accurate than humans can ever wish for. However, statistical accuracy is different to contextual accuracy and the human experience in general. In this sense, technology has definitely proven it can be trusted, but it must also warrant the trust.
Difficulty with opting out:
Just like with website cookies, where it’s a lot easier just to “Accept all” or opt-in to whatever ad analysis they want to do to get rid of that annoying pop up, it’s a lot easier just to consent to the use of FRT. If you do not opt-in, you are very much seen as an inconvenience.
How FRT affects our social behaviourA talk of mine would not be so without a little bit of philosophy. Here, I made sure to mention how the vigilance aspect of FRT could end up affecting how we conduct ourselves in social spaces. We could begin to become hyper aware of how we act, and whether this attends to the standards of the people behind the vigils. Here, we could now be treated as something ‘to be monitored’ and ‘to be tracked’ like with FRT being used in Myanmar to track protestors earlier this year.
The need for industry ethics
In this section, given the ethical issues at play, I highlighted the need for industry ethics. I mentioned Amazon’s Rekognition moratorium in 2020, as well as IBM and Facebook cancelling their ventures into FRT.
Current laws surrounding AI
I made sure to give my audience a flavour of what AI regulation is currently active. I specifically mentioned the Bolstering Online Transparency Act in California, as well as the Illinois video analysis law. I also mentioned how age-old laws like the Civil Rights Act can still play a part in algorithmic design, making sure the technology can’t discriminate on age, race, marital status etc.
Between the lines
My conclusion centres in how while AI is a sword to be wielded, it requires training to be used correctly. AI in the business world can serve as a great tool, but its potential issues are clear for all to see. However, through the study of the AI basics and the technology’s current state, I believe that the right training can be provided in order to appropriately utilize AI.