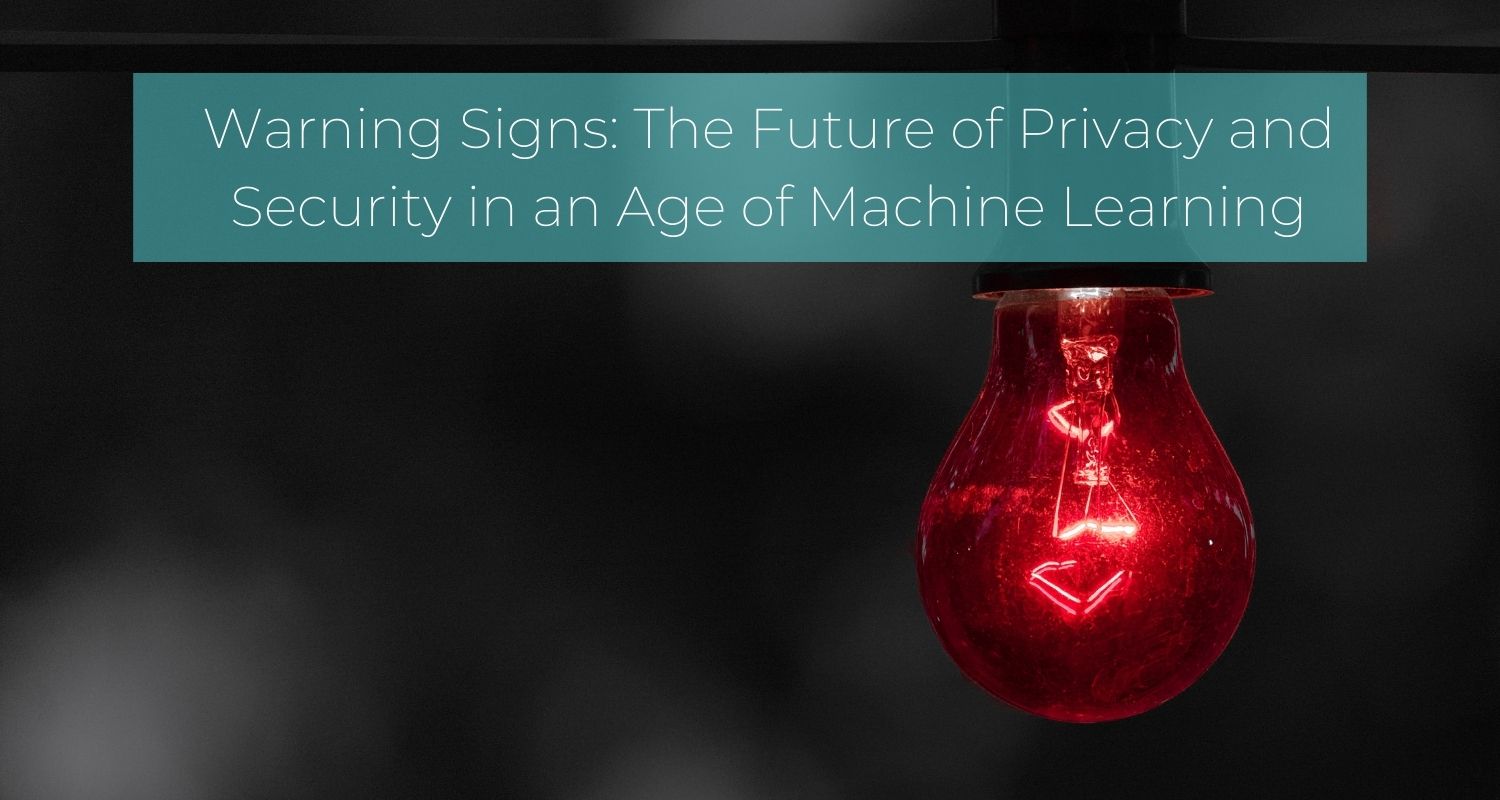
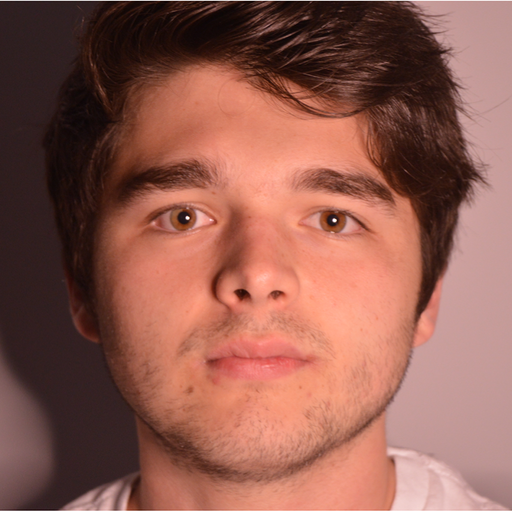
Summary contributed by our researcher Connor Wright (Philosophy, University of Exeter)
*Link to original paper + authors at the bottom.
Overview: Machine learning (ML) is proving one of the most novel discoveries of our time, and itās precisely this novelty that raises most of the issues we see within the field. This white paper serves to demonstrate how although not for certain, the warning signs appearing in the ML arena point toward a potentially problematic privacy and data security future. The lack of established practices and universal standards means that solutions to the warning signs presented are not as straightforward as with traditional security systems. Nonetheless, the paper demonstrates that there are solutions available, but only if the road of inter-disciplinary communication and a proactive mindset is followed.
Full summary:
Machine learning (ML) is proving one of the most novel discoveries of our time, and itās precisely this novelty that raises most of the issues we see within the field. This white paper serves to demonstrate how although not for certain, the warning signs appearing in the ML arena point toward a potentially problematic privacy and data security future. Having presented such warning signs, the paper suggests the approaches currently available to minimise them. Hence, Iāll discuss the ML environment and how its novelty presents some of the problems at hand, the splitting of the warning signs into informational and behavioural harms, and then Iāll pick out the solutions that grabbed my attention the most.
Given the infancy of the field, ML hasnāt benefited from having established best practices to guide decision making and design. Environments such as medical practice have an established history with multiple different instances of modern-day problems for which to refer to, with widely accepted standards of conduct (such as the Hippocratic oath), which ML just doesnāt possess. In this sense, when AI practitioners are having to make a decision on how to construct the model, there are no established standards nor any examples to guide them. In this sense, without any universal standards or reference points, it becomes up to the practitioners themselves to determine how they are to assure the privacy and security of their model. As the paper points out, such an individualistic approach to data security and privacy insurance will not be able to inspire widespread adoption of ML algorithms due to the need to observe that the standards employed by the practitioners are sufficient for the party who wants to employ the product. As a result, the paper states how the need for universally accepted best practices and, above all, standards for data security and privacy in ML models is imperative for the mitigation of MLās warning signs.
Partly the reason why warning signs currently exist is to highlight how different ML is to traditional cybersecurity systems. No longer is access to the source code the only way to manipulate a security system, with ML models able to be altered without such access at all. This opens up a myriad of ways to manipulate the system, which are encapsulated by the warning signs presented in the paper.
Such warning signs are split into two broad groups: informational harms and behavioural harms. Informational harms are where confidential information (data about participants) is unintentionally leaked or ācoaxed outā of the system by malicious actors. Mainly, such harms include the use of the output produced by the ML algorithm. For example, inferences on the characteristics of the members involved can be generated from the output, whereby the paper mentions a study where genetic markers of the individual patients were able to be inferred from the model¹. The output of the algorithm could also be used to reconstruct the model itself to be used for malicious purposes.
Both of these risks serve to show how an overall warning sign of the ML arena is that those within the data set arenāt the only ones that an be affected. As ML predictions become more and more powerful, while being increasingly trusted by professionals, the likelihood of its predictions affecting others not included in the training set increases. What this brings is now the risk of a generalisation fallacy of sample to population, whereby the inferences generated by the ML algorithm within the data set is applied to the wider public, where they may no longer be valid.
Behavioural harms, on the other hand, are where such actors alter the behaviour of the model itself. Acts such as poisoning (implanting malicious data to alter how the model performs) and evasion (introducing data intended to be misclassified by the model and influence the output as a result) are both captured within this group. With many algorithms not being fully transparent, the AI practitioners may not be able to adequately identify such data being implanted, nor how to remove it. One potential consequence mentioned by the paper is how evasion techniques could be used in the autonomous-vehicle field, resulting in the vehicles mis-identifying stop signs and putting more lives in danger. As a result, the paper suggests how these harms could best be mitigated, my highlights of which Iāll share now.
Not training the model on the raw data of those involved (such as in differential privacy) is currently proving a widely adopted method to counteract the warning signs presented. The data the model trains on is slightly perturbed thanks to an injection of noise, meaning that the outputs produced donāt completely reflect the data used (preventing model construction and data inference). Furthermore, keeping model documentation on how the model is to perform, as well as potential worst-case scenarios for how it could misbehave, will allow current and future practitioners to know when to act swiftly to identify the source of the problem. In this way, transparency is maintained as paramount. The elimination of the āblack-boxā nature of some algorithms will mean that practitioners are able to fully know how everything is supposed to operate, meaning there would be no possibility of malicious actors knowing more about the algorithm than its actual designers. Creating this form of shared understanding of the models at large will serve not only as reference points, but also the basis of a shared history in the practice, eventually creating established standards of practice.
Above all, the call for inter-disciplinary teams within the data security and privacy field made by the paper is my most important take-away. The privacy and security experts need the expertise of legal teams to assess the modelās societal viability, while the legal teams need the expertise of privacy and security experts to adequately explain their model. ML models do not exist in solely data privacy vacuums, meaning there are other elements in play (whether legal or societal) which require different sources of expertise to adequately deal with. For me, this will prove key in developing ML models that are to be most beneficial to future society.
While ML produces new problems completely separate to traditional examples, the solutions being produced are also presenting a novelty of their own. Dealing with model inversion or model poisoning is not something that had to be considered beforehand, but the fact that they have arisen now gives the ML community time to be proactive in their design, rather than reactive. The setting of universal standards through the help of shared experience and expertise from different professions will prove key in this proactive approach, and ultimately, how ML will affect our future.
¹ Matthew Fredrikson, Eric Lantz, and Somesh Jha, āPrivacy in Pharmacogenetics: An End-to-End Case Study of Personalized Warfarin Dosing,ā available at https://www.usenix.org/system/files/conference/usenixsecurity14/sec14- paper-fredrikson-privacy.pdf
Original paper by Sophie Stalla-Bourdillon, Brenda Leong, Patrick Hall, Andrew Burt: https://fpf.org/wp-content/uploads/2019/09/FPF_WarningSigns_Report.pdf