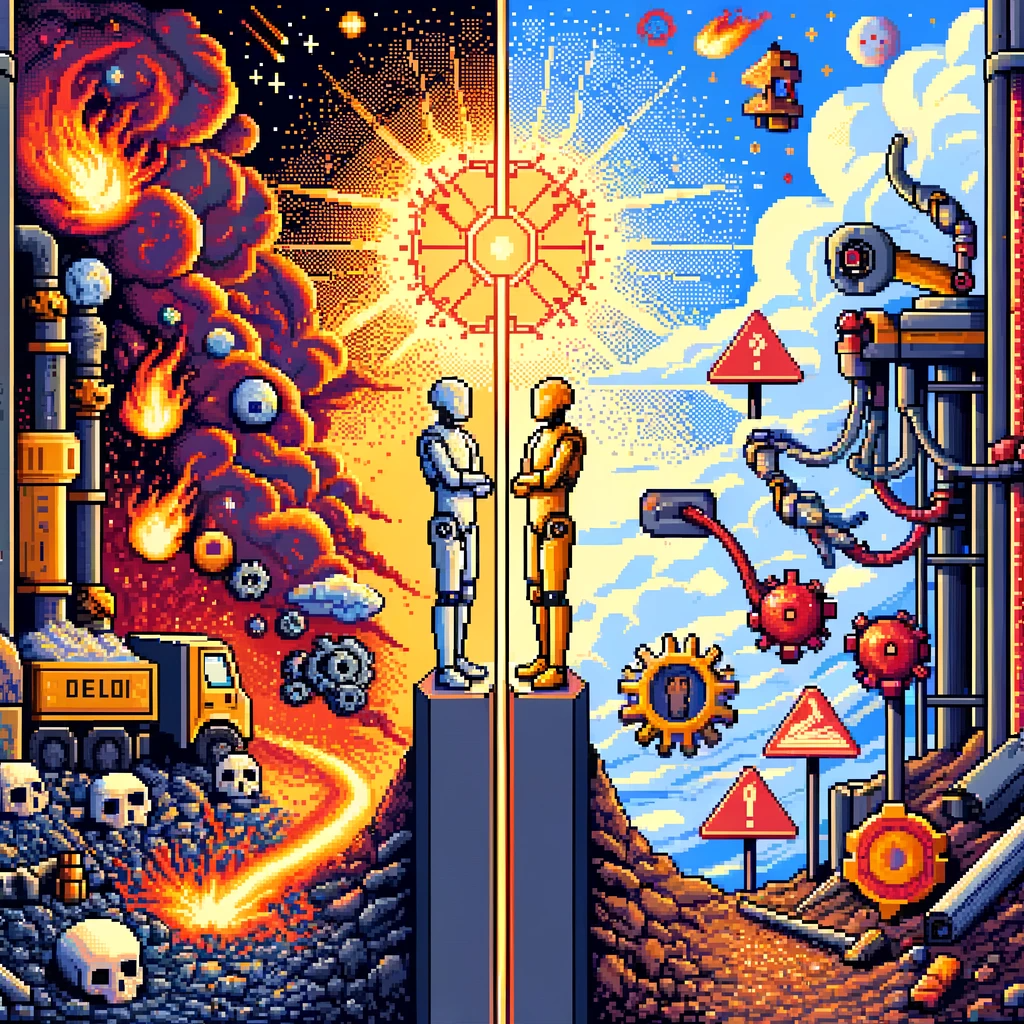
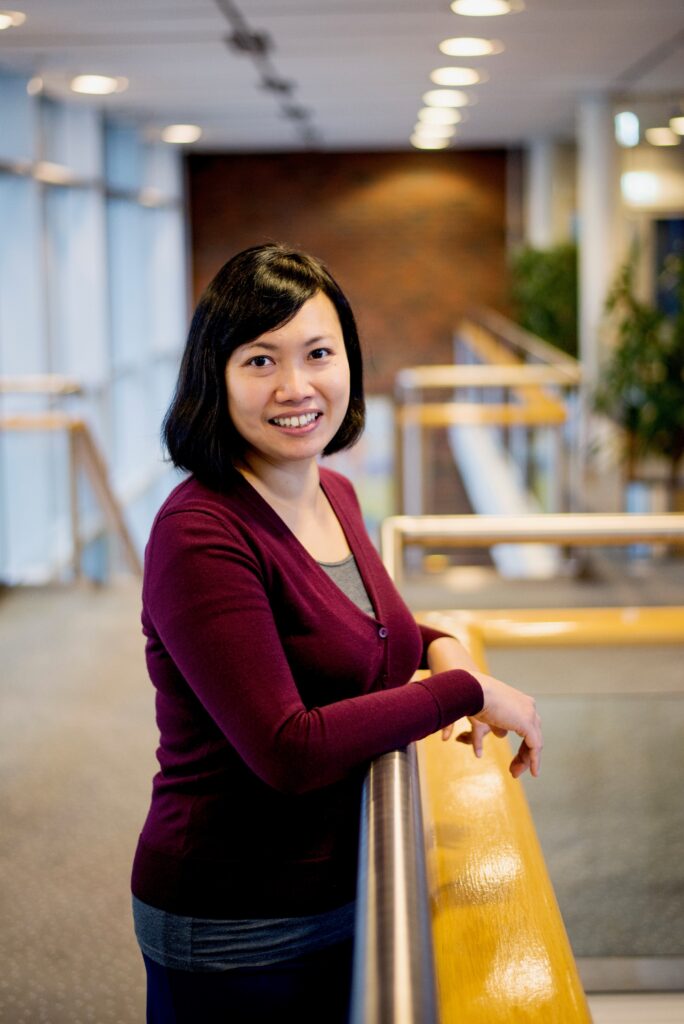
🔬 Research Summary by Tita Alissa Bach, Ph.D., is a Principal Researcher at the Digital Transformation research team at DNV, Norway, focusing on Human Factors in AI in safety-critical industries
[Original paper by Tita A. Bach, Jenny K. Kristiansen, Aleksandar Babic, and Alon Jacovi]
Overview: Failure to ensure quality human-AI interaction (HAII) in safety-critical industries can lead to unethical, catastrophic, and even deadly consequences. Despite this urgency, little research on HAII is fragmented and inconsistent. We present a survey of that literature and recommendations for research best practices focused on exploring commonalities across seemingly separate lines of HAII research for mutual learning and informing approaches to foster more conducive HAII in safety-critical industries.
Introduction
Why and how we did the study
Human-AI interaction (HAII) is a complex topic whose quality depends on contexts, users, and the AI system itself. This implies that the nature and quality of HAII can vary from user to user and context to context, even if the same AI system is being used, creating variability and non-deterministic outcomes. Therefore, to realize AI benefits for the benefit of the individual users and society, we need to study humans and AI together in specific safety-critical settings to ensure quality HAII.
We conducted a systematic literature review to investigate what has been done in HAII in safety-critical industries to identify learning points and set up a research agenda. We did this by investigating terms describing HAII, identifying factors influencing HAII, and examining how HAII is measured.
We only included empirical, peer-reviewed scientific articles or conference proceedings that focused on measuring HAII, involved real-world end-users in their studies, used a specific, tangible AI system or proof of concept, and applied their studies in a safety-critical industry. Through our search of digital databases, we identified 481 articles. However, only 13 of these met all our inclusion criteria, underscoring the substantial need for further research in this field.
Key Insights
What we found
Terms used to describe HAII
We found that no single universally accepted term is used across the literature to describe HAII, and certain terms can carry multiple interpretations. In addition to the terms “interaction,” “collaboration,” “handovers,” and “hand-offs” as identified in our review, the broader literature contains other similar terms such as human-AI “teaming, ““cooperation, ““symbiosis, ““coordination, “or “complementarity.” All these similar terms may or may not refer to identical concepts or have overlapping meanings. This divergence of terms presents a challenge to research aimed at improving HAII.
We use HAII in this review because it is effective and is already the most used term among a wide range of terms throughout the research. Nevertheless, we suggest further research on why these terminologies matter, which terminologies are best in each context, and accurate definitions of the terminologies. The goal is for AI communities to come together, discover each other’s work, and create a coherent forum for sharing findings that will help others study and implement AI generally and specifically in safety-critical industries.
Factors that influence HAII
According to our findings, five key factors influence HAII:
1. User characteristics and background refer to user profiles that influence HAII, such as personality, perceptions, educational backgrounds, preferences, and agreeableness to AI output.
2. AI interface and features refer to the importance of personalizing the design features and user interface of the AI system according to the needs of individual users, such as interactive UI design and AI emotive response as a feature.
3. AI output refers to determining which information of AI output should be presented to users, in which format and time points, and in what manner, such as including information about AI accuracy/confidence levels and actionable recommendations based on the AI output.
4. Explainability and interpretability refer to which AI rationale information should be communicated in what manner so that users accurately understand what is being communicated, such as including information about logical reasoning and reliability of the AI system and examining the user level of understanding of the algorithms used.
5. Usage of AI refers to how an AI system is used. For example, an AI system may be more effective to be used in a less complex scenario, and considering different ways an AI system can be used than what is envisioned by its developers to anticipate HAII.
These five key factors are interconnected and must be improved simultaneously to ensure the quality of HAII. For example, providing users with more detailed information on the rationale of AI output (i.e., improvement in AI output and explainability and interpretability) is likely to improve user perceptions and vice versa.
How HAII is measured
We found that HAII is most commonly measured with user-related subjective metrics such as user perception, trust, and attitudes. These are followed by objective metrics, including user task completion time, eye movement, and heart rate variability. This finding shows the importance of users’ opinions on a range of factors of AI systems. It thus highlights the importance of involving users in any development phase of AI systems. In addition, subjective metrics are valuable for understanding users’ buy-in and predicting what factors are important for which user groups. Such information can be used to create a more conducive environment for HAII.
This does not mean that objective measures should be disregarded. Rather, our findings show that there is a research gap in objective HAII measurements. Subjective measures depend on the respondent’s knowledge, memory, and self-reflection. Objective measures, which are usually based on physiological, physio-psychological, and outcome measures such as task completion time and number of collisions, can give rich information that cannot be gathered otherwise. The question is not whether subjective or objective is a better measure, but how to benefit from both to paint a more complete picture of HAII.
Between the lines
Our research underscores that HAII should be the focus in all contexts and situations involving AI systems. Regardless of how advanced and safe an AI system is, it cannot achieve its potential unless users are willing to and can interact with it seamlessly and effectively, as intended, within the designated operational environment. This is also a way to ensure the ethical and responsible use of AI systems.
One size does not fit all when it comes to ensuring quality HAII in safety-critical industries. A successful and responsible implementation of AI systems must be grounded in a well-researched use case that understands the users and their environment. Although the idea of a single AI system solving a wide range of problems for diverse users is appealing, our research shows that it is impossible due to the vast diversity within AI and HAII. This substantial gap in HAII research is due to the limited research in safety-critical industries and the fact that HAII is a multidimensional field. Our review encourages and calls for more multidisciplinary efforts to ensure quality and responsible HAII in safety-critical industries collectively.