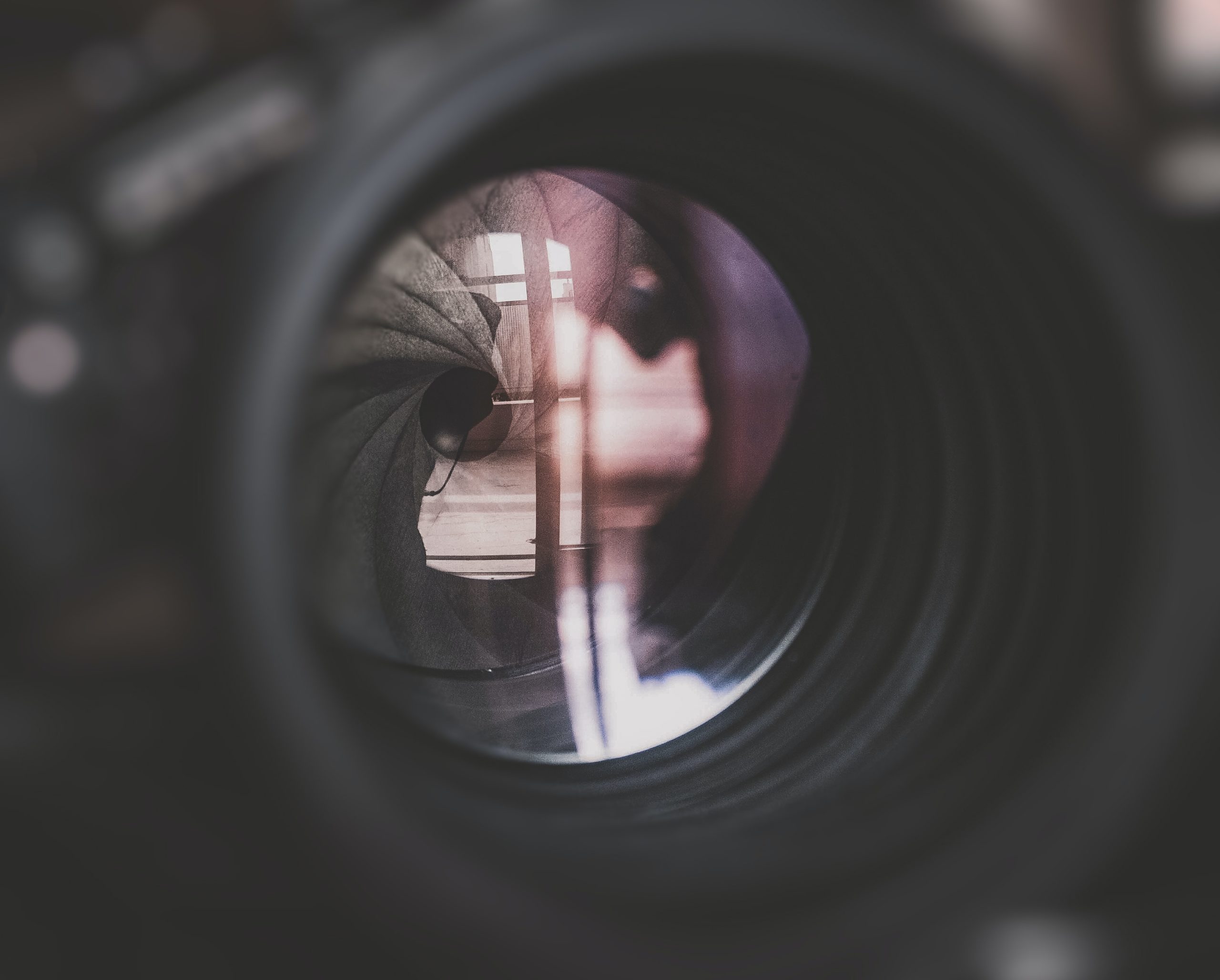
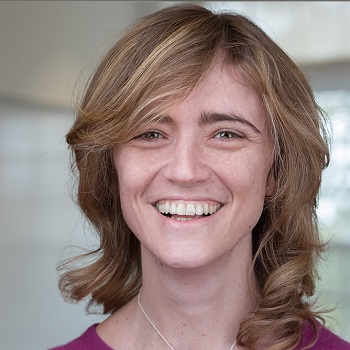
🔬 Research summary by Dr. Marianna Ganapini (@MariannaBergama), our Faculty Director.
[Original paper by Michele Loi, Andrea Ferrario, and Eleonora Viganòngsma]
Overview: It is often said that trustworthy AI requires systems to be transparent and/or explainable. The goal is to make sure that these systems are epistemically and ethically reliable, while also giving people the chance to understand the outcomes of those systems and the decisions made based on those outcomes. In this paper, the solution proposed stems from the relationship between “design explanations” and transparency: if we have access to the goals, the values and the built-in priorities of an algorithm system, we will be in a better position to evaluate its outcomes.
Introduction
How can we make AI more understandable? According to the authors of the paper, we care about making AI more intelligible mostly because we want to understand the normative reasons behind a certain AI prediction or outcome. In other words, we want to know: what justifies the outcome of a certain algorithmic assessment, why should I trust that outcome to act and form beliefs based on it? In the paper, the solution proposed stems from the relationship between “design explanations” and transparency: if we have access to the goals, the values and the built-in priorities of an algorithm-system, we will be in a better position to evaluate its outcomes.
Key Insights
The starting point for talking about transparency and explainability in AI is Lipton’s (2018) claim that interpretations of ML models are divided in two categories: model-transparency and post-hoc explanations. Post-hoc explanations look at the prediction of a model and include, most prominently, counterfactual explanations (Wachter et al. 2017). These are based on certain “model features” which, if altered, change the outcome of the model, other things being equal. By looking at the features that impacted a certain outcome, one can in theory determine the (counterfactual) causes that produced that outcome. Though these tools are often used in explainable-AI, the authors of the paper are skeptical: they believe counterfactual explanations do not provide the necessary insights to understand the normative aspects of the model.
Transparency should somehow tell us how the model works, at least in Lipton’s definition. However, the authors of the paper have something slightly different in mind: they believe transparency is really the result of making “design explanations” explicit. That is, we need to know what the system’s function is and how the system was designed to achieve that function. As the authors put it, “explaining the purpose of an algorithm requires giving information on various elements: the goal that the algorithm pursues, the mathematical constructs into which the goal is translated in order to be implemented in the algorithm, and the tests and the data with which the performance of the algorithm was verified.”
Parallely, they see “design transparency of an algorithmic system to be the adequate communication of the essential information necessary to provide a satisfactory design explanation of such a system.” The most prominent type of transparency in this context is value transparency: we need an accessible account of what values were designed in the system, how they were implemented and to what extent (what tradeoffs were made). Embedded values are values that are designed as part of an algorithmic system and that the system is also able to show in its output. As the authors explain, “[o]nce the criteria to measure the degree of goal achievement are specified” the “a design explanation of an algorithm should provide information on the effective achievement of such objectives in the environment for which the system was built.” That is called “performance transparency” in the paper.
This approach is meant to shed light on the goals algorithmic systems are designed to achieve, the values and tradeoffs built into the systems, the set of priorities the system is designed to have and the benchmarks for evaluating success and failure of this design. The goal of transparency is ultimately to provide “the public with the essential elements that are needed in order to assess the justification […] of the decisions” that are based on automated evaluations. If the decisions are based on a system not designed – either intentionally or at the level of how the values are translated – to foster some ethical values, then one might reasonably suspect the decisions made won’t match some ethical requirements. More importantly, these decisions cannot be morally acceptable since they are not motivated by the right set of priorities. Understanding all this is a key requirement for evaluating AI and the decisions made based on its recommendations.
Between The Lines
In this very interesting paper, the authors offer some actionable recommendations for how to make AI more understandable which seem fully in line with the idea of achieving an “ethics by design” approach to AI. Yet, we also believe that counterfactual and post-hoc explanations could be part of this approach with the goal, for instance, of checking for things that might have gone wrong. Therefore, we would not exclude them from an account of explainability in AI and we recommend a comprehensive approach to make AI understandable to humans.