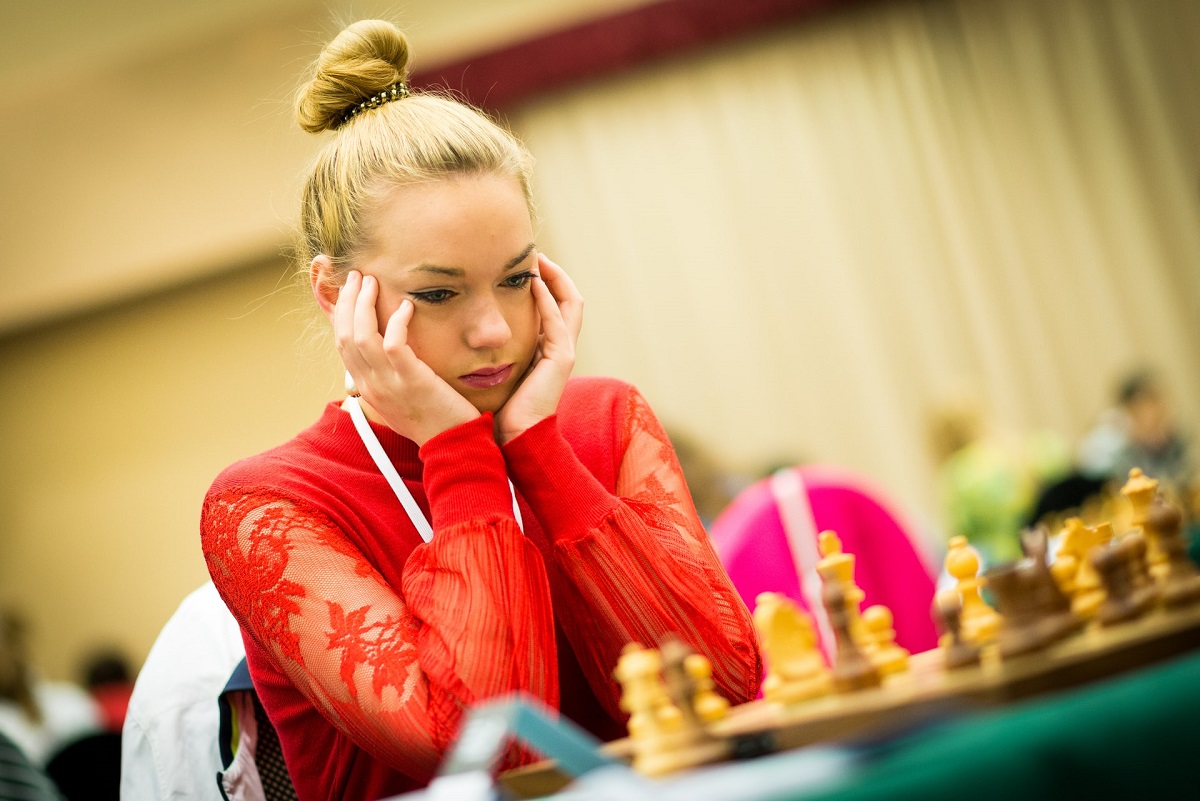
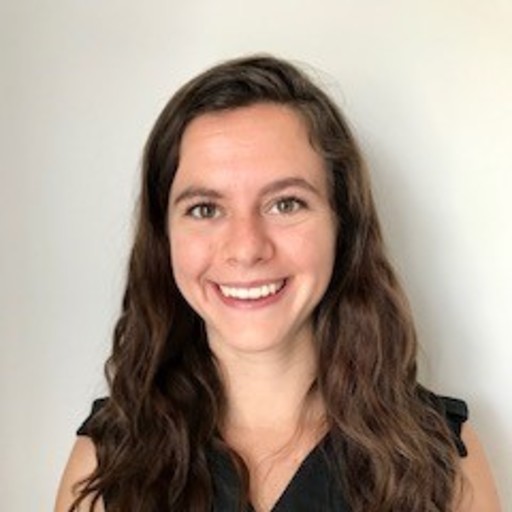
🔬 Research summary by Alexandrine Royer (@AlexandrineRoy2), our Educational Program Manager.
[Original paper by Josh Cowls, Andreas Tsamados, Luciano Floridi, Mariarosaria Taddeo]
Overview: 2020’s record-breaking temperatures renewed the push for technological solutions in tackling climate change. In this report, the authors offer recommendations for policymakers in determining AI’s potential to direct climate action without exacerbating the technology’s own environmental impact.
Introduction
With limited travel and no daily commute for many, COVID-19 housebound restrictions led to a noticeable reduction in global emissions of greenhouse gases in 2020. Yet, such unintended measures did little to curb rising temperatures and cure our climate catastrophe. In January, NASA announced that 2020 was tied with 2016 for the hottest year ever recorded, typifying the planet’s ongoing and dramatic warming trend.
With its capacity to process immense volumes of data, AI is already being used to forecast and model events relating to climate change. AI’s contribution to the climate crisis is twofold. In addition to helping predict the outcomes of rising temperatures, optimizing techniques can allow for greener solutions towards energy generation and use.
Our enthusiasm for AI’s potential to help us cope with climate events obscures how AI itself is a contributing factor in global climate change; particularly, the greenhouse gases emitted from training data and computation-intensive AI systems. Crowls et al. have sought to uncover the factors that contribute to AI’s carbon footprint and address “the trade-off between the emissions required to research, develop and deploy AI, and the energy and resource efficiency gains that AI can offer.” They offer a series of recommendations for policymakers and researchers within the field to determine how AI can help manage the impending effects of climate change without exacerbating the technology’s own environmental impact.
Pairing the green and blue: AI and the fight against climate change
The European Commission has been vocal of its environmental and digital vision for Europe’s economy and, as the authors note, “has embraced the digital and ecological ‘twin transitions’ that will shape our future”. The Commission views these two transitions “as being deeply interrelated,” with the European Green Deal roadmap report citing digital technologies as a significant resource in achieving the EU’s sustainability goals and measuring the impact of green policies. Referencing Floridi’s previous work in collaboration with Nobre, the authors point to how Green Deal exemplifies “a new marriage between the Green of habitats […] and the Blue of our digital technologies […]”.
Policymakers are eager to promote AI’s potential to assist climate change efforts and tend to forget how energy-hungry and ethically complex such systems are.
As the authors aptly point out, “For all the documents that have been produced by the European Commission in which AI has been identified as a key tool, and for all the opportunities they highlight, they tend to overlook the challenges that must be addressed to ensure a successful and sustainable adoption of AI tools”.
3 things to consider when using AI to combat climate change
To accurately assess AI’s potential for climate action, the first step is to identify where it is already being deployed worldwide. Given the speed of technological change, there is currently no single survey that is sufficient to reach a complete overview. So far, there have been a few databases on projects related to AI and UN Sustainable Development Goal 13 (take urgent action to combat climate change and its impact), – the University of Oxford AIxSDG among these – as well as one large-scale study by Rolnick and colleagues on ‘Tackling Climate Change with Machine Learning’ in 2019.
The second step is to consider the ethical pitfalls of using AI solutions. A recurrent concern is that AI models are trained on datasets that produce biased outcomes. One example offered by the authors is using AI to determine charging stations’ locations for electric vehicles based on consumption patterns, which can likely lead to an overabundance of stations in wealthier neighbourhoods. Overreliance on AI may also erode human autonomy in ways that are detrimental to efficient climate action, as climate change requires coordinated action among multiple players- including individuals. Another ethical conundrum is the use of geographical data and the monitoring of human behaviour in AI systems that track an individual’s carbon emissions, which goes against one’s privacy rights.
A third -and often neglected factor- is to calculate the energy required by AI systems that are themselves designed to monitor energy-use and the impact of current environmental policies. AI is not a zero-cost environmental solution; data centers require vast amounts of energy to operate. Some data centers are more polluting than others as the sources of energy, whether renewable or not, evidently differ depending on location.
As the authors underline, “since the advent of deep learning […] the computing power required for model training has doubled every 3.4 months, resulting in growing energy demand”. For example, one training run of the GPT-3, a model which uses deep learning techniques to create human-like texts, can produce as much annual carbon emissions as 49 cars if it were based in the US. Improvements in microchips, which are now equipped with multiple processor cores, also greatly increase AI’s energy demands.
Do the costs outweigh the benefits?
While major data centre operators, among them cloud providers like Microsoft and Google, have invested in more energy-efficient infrastructure, there remains a lack of transparency regarding how their annual greenhouse gas emissions are calculated. To evaluate the sustainability of an AI system, the main factors to consider include the type of hardware, training duration, energy resources used by the energy grid supplying the electricity, memory usage and otherss.
There are also broader systematic issues within AI studies that contribute to energy waste. For example, many papers do not disclose their source codes and often provide limited information on the training conditions behind the achievement of their results. This often leads to massive computational power being generated to replicate and fine-tune such systems. Only wealthy research groups can afford to run such expensive experiments, adding another barrier to smaller institutions outside Asia and Euro-America that struggle to compete with tech giants. One research paper by Google Brain revealed how 12 800 neural networks were trained to obtain a 0.09 percent improvement on an AI model.
Though AI can alleviate tasks that would have required more time, human efforts, and possibly energy, the authors encourage AI developers to question whether the short-term costs significantly outweigh the long-term benefits.
For Crowls et al., “the field needs to reconsider its dedication to computer-intensive research for its own sake, and to move away from performance metrics that focus exclusively on accuracy improvements.”
Moving forward: Recommendations for building a greener AI
The EU, with its dual commitments to climate change and digitization, can take a leading role towards greener AI. As the authors note, “the EU would be a perfect sponsor in addressing the complexities associated with the technology’s own contribution to the problem and in meeting the need for coordinated, multilevel policymaking to ensure the successful adoption of AI solutions.”
The ethical AI space has praised the EU for taking proactive measures towards data privacy and the same legislative enthusiasm to ensure socially beneficial AI should translate to its environmental goals. The EU has the means to fund a large-scale investigation into the uses of AI in climate action and, hence determine industry standards to keep the carbon footprint of AI in check. It also has the political leverage to encourage business-to-business and business-to-government data sharing to facilitate more sustainable AI-based solutions. Finally, EU policymakers can level the European-wide playing field in terms of making research facilities- and the computational power they require-more accessible and affordable. Read the authors’ full list of recommendations in the original paper.
Between the lines
Crowls et al. report is a welcome addition to the AI and environment literature. It calls on policymakers to take an active role in tackling the climate crisis through technological innovation without losing sight of such technologies’ environmental costs. Creating more sustainable AI systems is only a partial solution to the growing global demand for computational power- and energy more generally. Green AI also involves investing in low and middle incomes countries’ capacities to develop renewable and reliable energy sources. It demands that we reconsider our impulses to provide AI-driven solutions rather than perfect existing systems. Indeed, why finance autonomous electric cars when you can improve public transport?