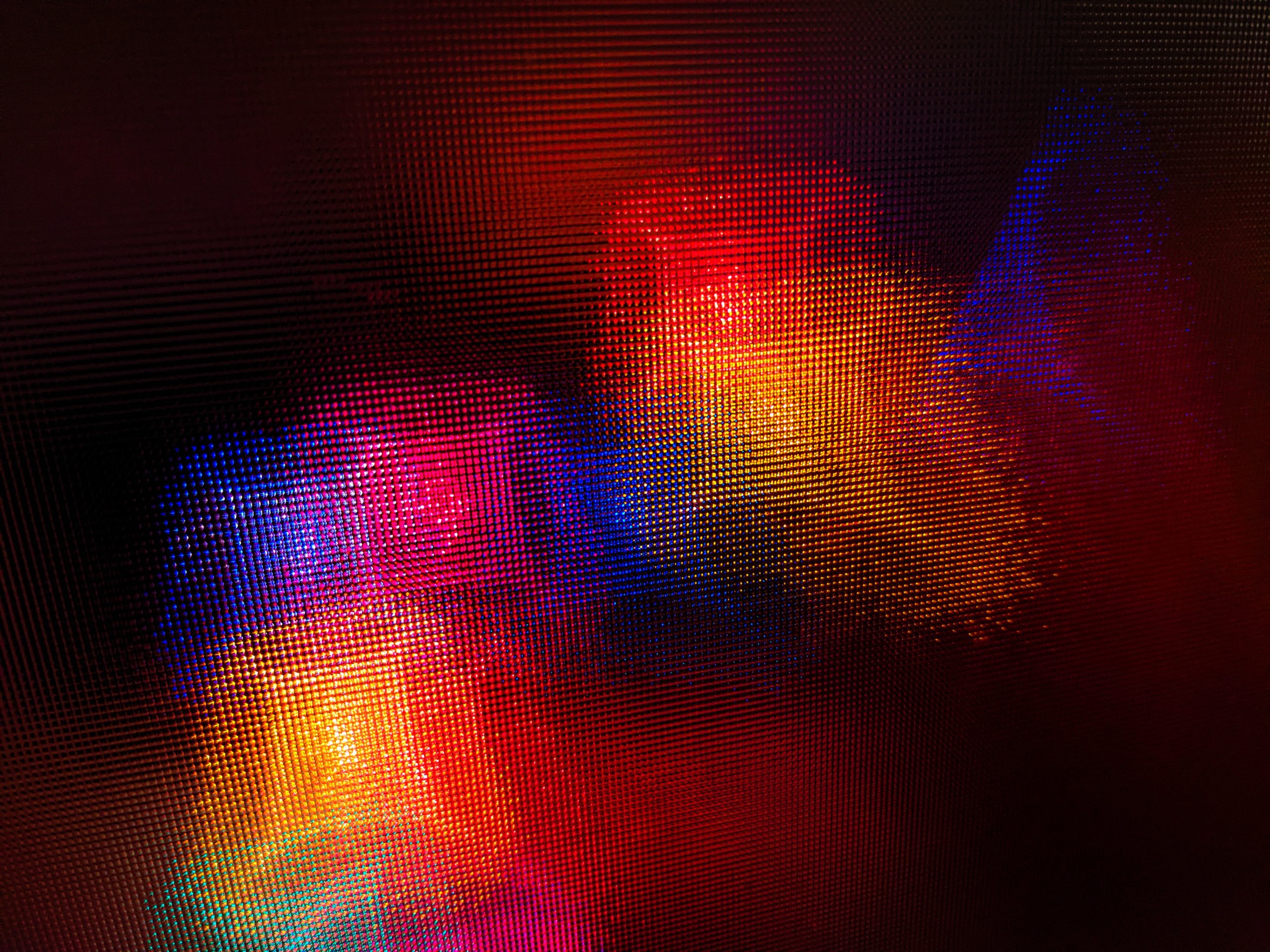
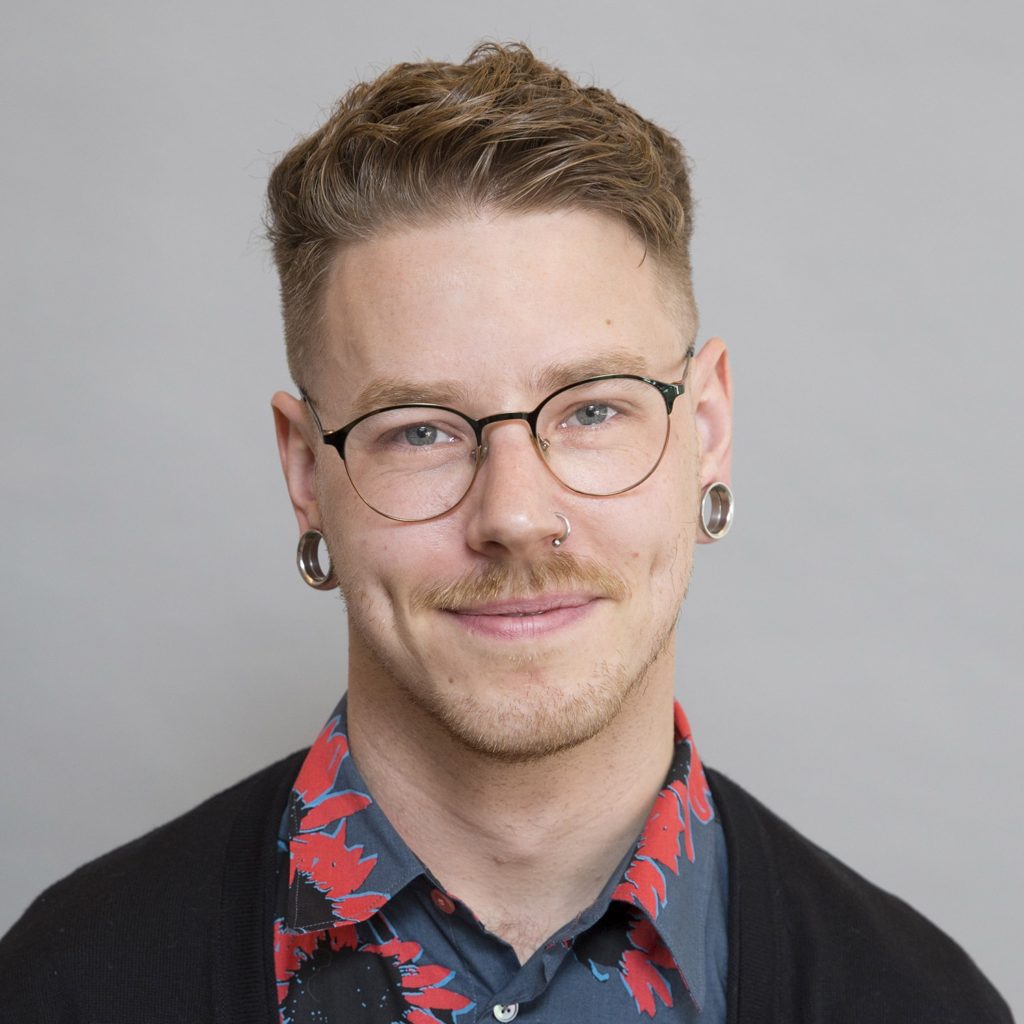
đŹ Research Summary by Travis LaCroix, an assistant professor (ethics and computer science) at Dalhousie University
[Original paper by Travis LaCroix]
Overview: Researchers focusing on implementable machine ethics have used moral dilemmas to benchmark AI systemsâ ethical decision-making abilities. But philosophical thought experiments are designed to pump human intuitions about moral dilemmas rather than serve as a validation mechanism for determining whether an algorithm âisâ moral. This paper argues this misapplication of moral thought experiments can have potentially catastrophic consequences.
Introduction
Benchmarks are a common tool for measuring progress in AI research. At the same time, AI ethics is increasingly prominent as a research direction. Thus, it stands to reason that we need some way of measuring the âethicalityâ of an AI system. We want to determine how often a model chooses the âcorrectâ decision in an ethically-charged situation or whether one model is âmore ethicalâ than another.
Key Insights
Benchmark Datasets. A benchmark is a dataset and a metric for measuring the performance of a model on a specific task. For example, ImageNetâa dataset of over 14 million labelled imagesâcan be used to see how well a model performs on image recognition tasks. The metric measures how accurate the modelâs outputs are compared to the ground truthâi.e., the image label. There is a matter of fact about whether the systemâs decision is correct. The rate at which the modelâs outputs are correct measures how well the model performs on this task.
Moral Decisions. Some models act in a decision space that carries no moral weight. For example, it is inconsequential whether a backgammon-playing algorithm âchoosesâ to split the back checkers on an opening roll. However, the decision spaces of, e.g., autonomous weapons systems, healthcare robots, or autonomous vehicles (AVs) may contain decision points that carry moral weight. For example, suppose the brakes of an AV fail. Suppose further that the system must âchooseâ between running a red lightâthus hitting and killing two pedestriansâor swerving into a barrierâthus killing the vehicle’s passenger. This problem has all the trappings of a âtrolley problemâ. The trolley problem is a thought experiment introduced by the philosopher Philippa Foot (1967). Its original purpose was to analyse the ethics of abortion and the âdoctrine of double effectâ, explaining when it is permissible to perform an intentional action with foreseeable negative consequences.
Moral Benchmarks. The standard approach in AI research uses benchmarks to measure performance and progress. It seems to logically follow that this approach could apply to measuring the accuracy of decisions with moral weight. That is, the following questions sound coherent at first glance.
How often does model A choose the ethically-âcorrectâ decision (from a set of decisions) in context C?
Are the decisions made by model A more (or less) ethical than the decisions made by model B (in context C)?
These questions suggest the need for a way of benchmarking ethics. Thus, we need a dataset and a metric for moral decisions. Some researchers have argued that moral dilemmas are apt for measuring or evaluating the ethical performance of AI systems.
Moral Machines. In the AV case described above, the most common dilemma that is appealed to is the trolley problemâdue, in no small part, to the Moral Machine Experiment (MME). The MME is a multilingual online âgameâ for gathering human perspectives on trolley-style problems for AVs. Some of the authors of this experiment suggest their dataset of around 500,000 human responses to trolley-style problems from the MME can be used to automate decisions by aggregating people’s opinions on these dilemmas.
Moral Dilemmas for Moral Machines. The thought is that moral dilemmas may be useful as a verification mechanism for whether a model chooses the ethically-âcorrect’ option in a range of circumstances. In the example of the MME data as a benchmark, the dataset is the survey data collected by the experimentersâi.e., which of the binary outcomes is preferred by participants, on average. Suppose human agents strongly prefer sparing more lives to fewer. In that case, researchers might conclude that the ârightâ decision for their algorithm to make is the one that reflects this sociological fact. Thus, the metric would measure how close the algorithmâs decision is to the aggregate survey data. However, this paper argues that this proposal is incoherent because, in addition to being logically fallacious, it misses the point of philosophical thought experiments, like moral dilemmas. In a related project, co-authored with Sasha Luccioni, we argue that it is impossible to benchmark ethics because the metric has no ground truth.
The Use of Thought Experiments. Although there is a vast meta-philosophical literature on the use of philosophical thought experiments, one such use is to pump intuitions. Roughly, a scenario and target question are introduced. When cases evoke incompatible or inconsistent reactions, this is supposed to shed light on some morally salient differences between the cases. In the trolley problem, we ask why it might seem morally permissible to act in one scenario but not in another, although their consequences are identical. The thought experiment elucidates something about our intuitions regarding certain actionsâ moral rightness or wrongness. Then we can work toward a normative theory that consistently explains those intuitions. However, the thought experiment does not tell us which action is correct in each scenario. A moral dilemma is a dilemma, after all. The thought experiment is useful because people are less likely to carry pre-theoretic intuitions about trolleys than about abortions.The Danger of Proxies. A moral benchmark would need to measure the accuracy of a model against a ground truthâwhat is the morally-âcorrect’ choice in a situation. However, thought experiments like moral dilemmas provide no such ground truth. At best, they tell us a sociological fact about which outcomes people say they would prefer in such a situation. This is precisely what the MME data provides. However, if this is the benchmark dataset for moral AI, then sociological facts are a proxy for the actual targetâmoral facts. This precedent is dangerous for work in AI ethics because these views get mutually reinforced within the field, leading to a negative feedback loop. The actual target(s) of AI ethics are already highly opaque. The more entrenched the approach of benchmarking ethics using moral dilemmas becomes as a community-accepted standard, the less individual researchers will see how and why it fails.
Between the lines
This research matters because a âcommon senseâ approach to morality assumes objective truths about ethics. Although such platitudes have been questioned in a philosophical context, they are pervasive in how we think about the world. However, it is important to maintain sensitivity to the distinction between descriptive claims (what people believe is ethical) and normative claims (what one ought to do). Using moral dilemmas as an elucidatory tool is neither prior to, nor follows from, moral theorising about AI applications.