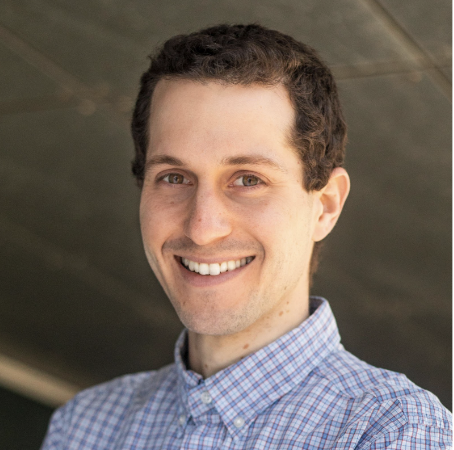
🔬 Research Summary by Daniel Hershcovich, a Tenure-Track Assistant Professor at the Department of Computer Science, University of Copenhagen, working on meaning representation and on promoting sustainable and responsible behavior in and with Natural Language Processing.
[Original paper by Daniel Hershcovich, Nicolas Webersinke, Mathias Kraus, Julia Anna Bingler, and Markus Leippold]
Overview: The environmental impact of AI, particularly Natural Language Processing (NLP), has become significant and is worryingly increasing due to the enormous energy consumption of model training and deployment. This paper draws on corporate climate reporting standards and proposes a model card for NLP models, aiming to increase reporting relevance, completeness, consistency, transparency, and accuracy.
Introduction
Deep learning and, specifically, large language models (LLMs) drove recent breakthroughs in AI capabilities but at a high environmental cost. For example, when OpenAI trained GPT-3 in 2020, the associated energy consumption resulted in carbon emissions equivalent to three round-trip passenger jet flights between San Francisco and New York (the whole plane, not just one passenger). This is a lot but is probably nothing compared to the deployment of this popular model accumulated over the years. The carbon footprint of the AI industry is comparable to that of the aviation industry, and it is only expected to grow in the coming years with increasingly larger deep learning models. How bad is this for the climate and the energy crisis? It’s hard to tell without standardized reporting.
By surveying NLP research papers from the past six years, the authors find that energy consumption and carbon emission reporting is becoming slightly more common but is still only present in less than 5% of research papers that use deep learning for NLP. To address this, the paper proposes a climate performance model card that is practically usable even by researchers with limited expertise and knowledge about carbon emissions and the underlying computer hardware.
Key Insights
Greenhouse Gas Protocol
The Greenhouse Gas Protocol (GHG Protocol) is a standardized approach for measuring, managing, and reporting corporate greenhouse gas emissions. Firms widely use it to report their climate performance, which is considered a best practice. The principles of the GHG Protocol include relevance, completeness, consistency, transparency, and accuracy, which are meant to prevent greenwashing. Greenwashing refers to the practice of making false or misleading claims about the environmental benefits of a product or service to mislead consumers into thinking it is more environmentally friendly than it actually is. The GHG Protocol defines three scopes of emissions: Scope 1 emissions are those that the organization directly emits, Scope two emissions are those that the organization indirectly emits as a result of the consumption of purchased electricity, steam, heat, or cooling, and Scope 3 emissions are those that are indirectly emitted as a result of the organization’s input purchases and use of outputs by end consumers. Scope 3 covers activities from sources not directly owned and indirectly controlled by the organization. The authors argue that similar standards should be applied to AI research, particularly in the field of NLP, which has seen significant progress in recent years but also has a large carbon footprint due to the energy consumption of training and running computational models. The increasing number and size of LLMs being trained and deployed make it even more important to pay attention to the environmental impact of this research.
Translating to NLP Research
To adapt the principles of the GHG Protocol to NLP research, the authors suggest that researchers ensure their climate-related performance assessments appropriately reflect the actual climate-related performance of their models, account for all relevant items, use consistent methodologies, be transparent about their assumptions and methodologies to assess the carbon footprint and achieve sufficient accuracy in their reporting. Reporting climate-related performance is not an end but a means to increase awareness and take action to improve the climate performance of NLP models. It is also important to consider the long-term impact of these models and aim for climate-resilient NLP algorithms that can positively affect a carbon-constrained and highly energy-efficient future. Transparent reporting on the climate-related performance of NLP models can help researchers reflect on their work and make improvements. It can also help downstream technology users make informed decisions about using these models. The authors propose a climate performance model card to support this process.
Model Cards in AI
Model cards are short documents that provide a benchmarked evaluation of machine learning models in various conditions and disclose relevant information about the model’s intended use, performance evaluation procedures, and other details. The climate performance model card proposed in this paper is designed to be practically usable by researchers with limited expertise and knowledge about carbon emissions and computer hardware. It includes ten entries: (1) whether the resulting model is publicly available, (2) the time required to train the final model, (3) the time required for all experiments (including hyperparameter search), (4) the power of the GPU and CPU, (5) the location where the computations were performed, (6) the energy mix at that location, (7) the emissions resulting from training the final model, (8) the emissions resulting from all experiments, (9) the average emissions for the inference of one sample, and (10) any positive environmental impact that can be expected from the work. The authors argue that using model cards can increase awareness about the climate impact of NLP research and facilitate more thorough discussions on this topic.
Recommendations
The authors provide recommendations for NLP researchers to increase transparency and awareness about the climate impact of their work: using the proposed climate performance model card to report on energy consumption and emissions and to road-test its use in research institutions’ climate impact reporting. However, using the model card to assess research quality or net climate-related impacts is discouraged. Instead, the approach aims to increase transparency about the first-order effects of NLP models.
Between the lines
The importance of understanding and addressing the climate impact of NLP research cannot be overstated, as the increasing demand for computational power threatens to worsen the already dire climate crisis. The proposal of a climate performance model card is a welcome step toward increasing transparency and accountability in NLP research. The model card can raise awareness and encourage more sustainable practices by providing a framework for researchers to report on key aspects of their work, such as energy consumption and emissions. However, it will be crucial for the research community to adopt and consistently use the model card to truly make a difference, for example, by making it mandatory for submission to publication venues. It will also be important for researchers to consider the long-term impacts of their work and strive for climate-resilient NLP and algorithms that can have positive, lasting effects.