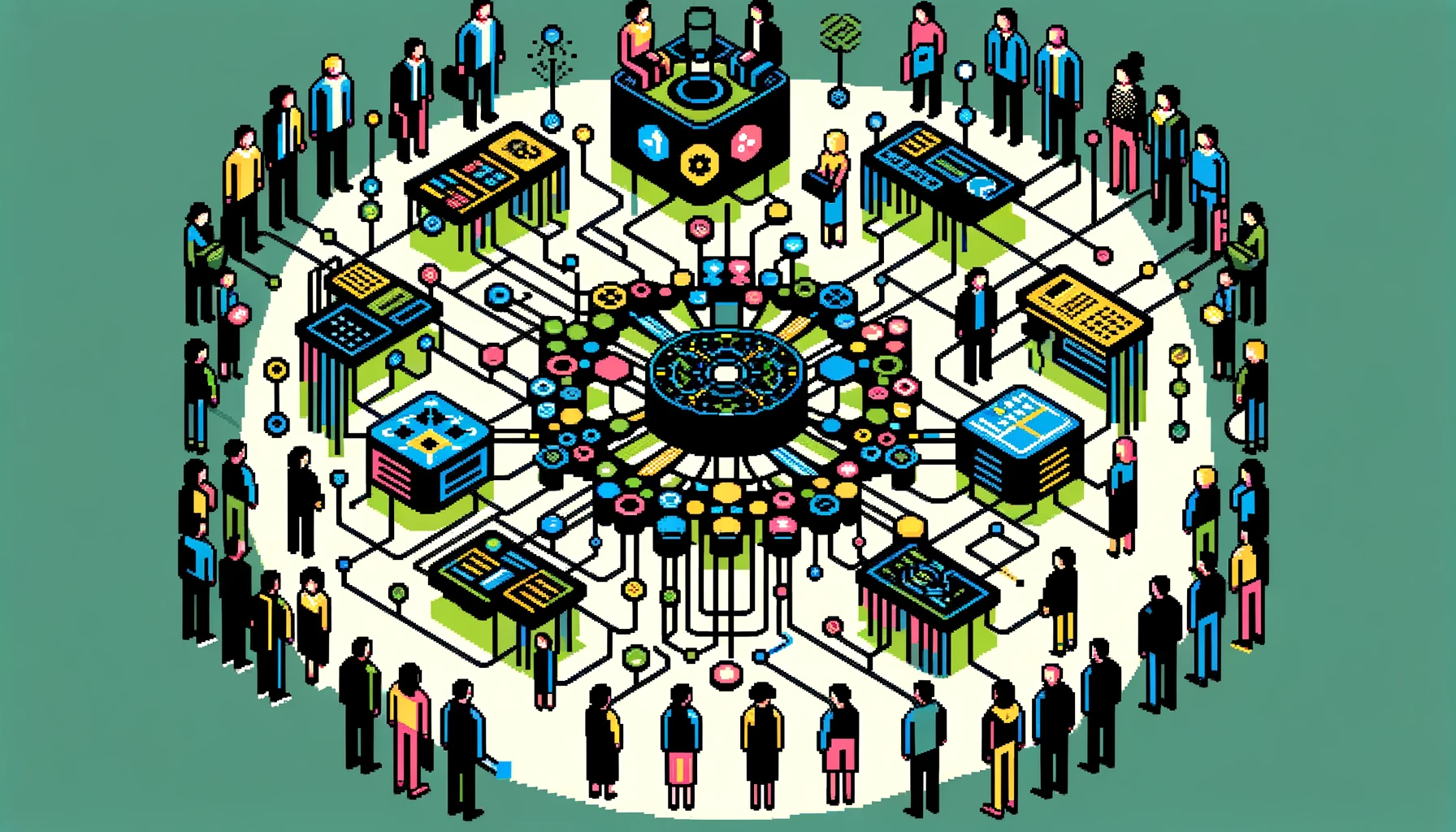
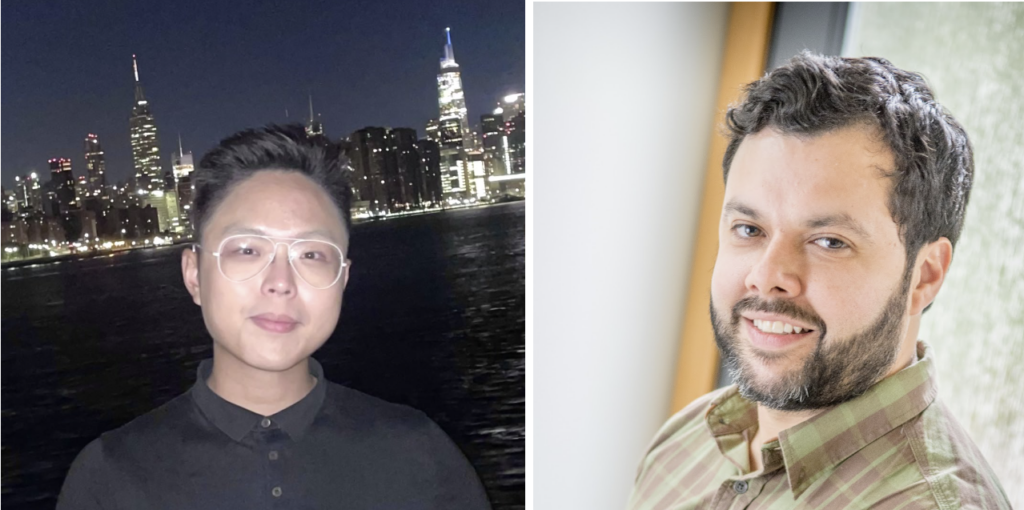
🔬 Research Summary by Stephen Yang and Fernando Delgado.
Stephen Yang is a PhD student at the University of Southern California studying how careful human intervention is possible at the speed and scale of AI. He’s also an expert consultant for Partnership on AI on equity-centered AI.
Fernando Delgado is a PhD candidate at Cornell University studying how non-technologists participate in AI design and how AI techniques are transparent to non-technical system stakeholders. He is also the Director of AI & Analytics at Lighthouse Global, a pioneering legal technology firm.
[Original paper by Fernando Delgado, Stephen Yang, Michael Madaio, and Qian Yang]
Overview: This paper introduces an empirically grounded framework that can guide researchers and practitioners in better explicating the tradeoffs between different participatory goals and approaches when designing AI systems. We provide empirical evidence showing how the current landscape of participatory AI is largely consultative and focused on soliciting discrete inputs from stakeholders on isolated aspects of AI systems. To move the field forward, we call for a departure from conceptualizing participation as an all-or-nothing characteristic of design efforts, advocating instead for articulating how one’s goals for participation are aligned with the methods and scope of stakeholder input into AI design.
The Conceptual Muddle of “Participatory AI”
The past few years have seen great enthusiasm about “participatory AI” –– the idea that participation from affected stakeholders can be a way to incorporate the wider publics into the design and development of AI systems. This interest spans technology companies, such as OpenAI’s call for democratic inputs to AI; nonprofits, with Ada Lovelace Institute examining public participation methods in commercial AI labs; as well as academia, where a workshop at the International Conference on Machine Learning called “Participatory Approaches to Machine Learning” garnered considerable attention.
The aforementioned examples illustrate a growing consensus that stakeholders “should” participate in AI design and development. Yet, there’s a lack of shared understanding of the theories and methods of participation within the AI community –– AI practitioners may be navigating between potentially contradictory approaches and goals that may all be branded as “participatory AI.”
Against this backdrop, this paper presents a conceptual framework that can guide practitioners of participatory AI (and those interested in designing or evaluating participatory AI approaches) in understanding the differences in a broad range of participatory goals and methods. Then, we use that framework to understand the current landscape of participatory AI by analyzing 80 research articles in which authors report using participatory methods for AI design and interviewing 12 authors of these papers to understand their motivations, challenges, and aspirations for participation.
We find that most current participatory AI efforts consult stakeholders for input on individual aspects of AI-based applications (e.g., the user interface) rather than “empowering” them to make key design decisions for datasets, model specifications, or broader questions of appropriate use cases, or whether AI should be used at all. We discuss how many AI practitioners feel caught between their aspirations for participation and practical constraints. Thus, we draw on approaches to “proxy-based participation” –including human stand-ins and algorithmic proxies– to represent stakeholders in shaping AI systems with significant potential drawbacks.
Re-Orienting the Meanings of Participation
Taking a step back from the context of AI design and development, we first tried to understand how various fields have conceptualized public participation. As a starting point, we synthesized literature across technology design, policy, and governance, as well as the social sciences, to derive our conceptual framework of the different aspects of participation:
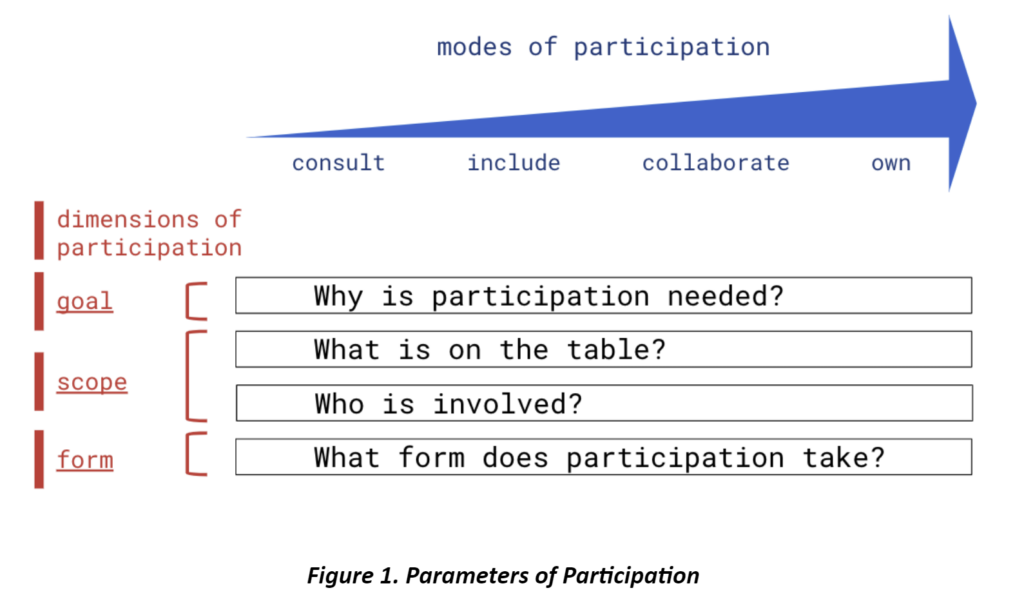
We identified four key dimensions that inform the goals and methods of participation ––
Dimension #1 – Why is Participation Needed
Motivations to engage stakeholders broadly fall under two categories: an instrumentalist motivation that stakeholder participation will result in better outcomes or a normative motivation that participation is important because including affected stakeholders is the right thing to do.
Dimension #2 – What is on the Table
Oftentimes, participation translates to selecting among choices pre-determined by technical experts. Other times, “what is on the table” is treated as a moving target, where participation shapes the broader design decisions, deliberation procedures, and project trajectories.
Dimension #3 – Who is Involved
There are great variations in the rationale and selection criteria for who gets to participate. For instance, most projects involve direct stakeholders and end users, while some intentionally involve marginalized voices and participants with less technological expertise.
Dimension #4 – What Form Does Participation Take
Participation typically takes the form of ranking alternatives on polls, discussing trade-offs between options, and co-creating prototypes for new design ideas.
We also identified four modes of participation that form a spectrum of stakeholders’ involvement –– from consult, include, collaborate, to own. This framework can help demystify what people mean when they refer to AI projects as “participatory.”
A Largely Consultative Landscape
Using the framework to analyze a corpus of 80 papers reporting on participatory AI projects, we found a largely consultative landscape of participatory AI practices. Within each cell, we provide exemplar approaches from the corpus of 80 papers describing participatory AI projects:
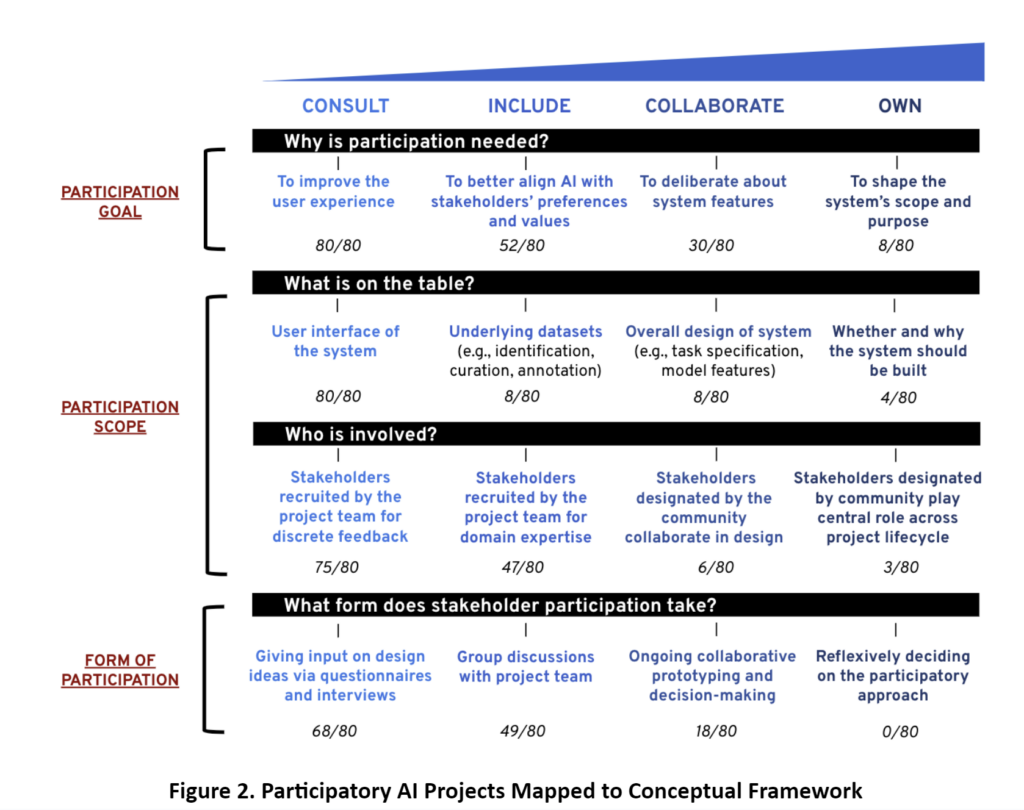
First, we found that in motivating “why is participation needed,” most projects described their goal as improving the user experience or aligning AI with stakeholders’ preferences and values. Only 10% of the projects involved stakeholders in shaping the system’s scope and purpose.
Regarding “what is on the table?” most projects surveyed focused their participatory interventions exclusively on informing the user interface, much like the goals we saw for participation. Only 10% of the projects give stakeholders a say in how the underlying model works, and only 5% allow stakeholders to rule out AI as a solution.
Regarding “who is involved?” in 94% of the projects, the stakeholders were identified and chosen based on criteria pre-determined by the project team. This contrasts with the much smaller number of projects (6 out of 80) that leveraged a community-led approach in determining the stakeholders at the table.
Regarding “forms of participation,” 85% of the projects engaged stakeholders in eliciting preferences. Such participation primarily occurred during designated windows once the system’s scope and purpose were already determined — or were specifically scoped to user interface aspects rather than the underlying AI model.
Proxy-Based Participation
So, what led to this consultative landscape? To understand how we got here, we interviewed 12 authors of papers from our corpus to understand their motivations, challenges, and aspirations of doing participatory AI.
Overall, our interviewees described feeling caught between an idealized world of stakeholder empowerment and the top-down organizational constraints of available time and resources. To navigate between their ideals and constraints, these AI practitioners turned to three proxies for participation –– representative stand-ins, UX/HCI experts, and algorithmic proxies. We outlined such approaches and their associated risks:
Proxy #1 – Representative Stand-Ins
In this approach, participants involved in AI design were not necessarily members of an affected stakeholder group but individuals the project team perceived as able to stand in and voice stakeholders’ preferences and values based on lived experiences. However, it is unclear to what extent the recruited participants represent the groups in question.
Proxy #2 – UX/HCI Experts as Mediators
Other times, project teams designate UX/HCI experts as a bridge between domain stakeholders and AI practitioners. These experts play a central role in deciding which stakeholders to bring in as participants, what role those participants played, and how participants interacted with each other and other team members. Nonetheless, they may inadvertently elevate or augment some voices while leaving others out of the conversation.
Proxy #3 – Algorithmic Proxies
In addition to human proxies, AI project teams recruit stakeholders to train models by voting on preferences or outcomes that nominally reflect their goals. The assumption underlying this approach holds that a predictive model trained on people’s preferences is functionally equivalent to those preferences. This form of participation may narrowly limit stakeholders’ input to predefined policies, preferences, or model outputs — as well as fail to capture people’s changing values and preferences.
Between the lines
Our paper raises questions about whether (or how) meaningful human participation is feasible at the scales at which many AI systems are designed and operated. Careful human interventions and scaling up are often in tension with each other. Our paper suggests two sets of considerations for future researchers to contemplate:
First, as the currently popular paradigm of AI systems is often downstream “applications” of pre-trained “foundation” models (e.g., large language models), it becomes useful to think about the distinction between participating in application design, such as in the form of third-party applications or APIs, and participating in dataset or model design. In the latter’s case, this would mean directly shaping base models like GPT-4. Yet, the extent to which stakeholders can meaningfully contribute to system-wide decisions on this level remains to be explored.
Second, as AI systems are inherently adaptive, it raises questions about when, how often, and by which means it makes sense to bring stakeholders into the loop. Our work raises questions about how researchers can balance empowering and over-burdening when designing adaptive systems.
By providing a conceptual orientation and empirical foundation for participatory AI, this paper may help guide future researchers and practitioners to connect their goals for participation with methods to achieve such goals –– and we believe it can be useful for third parties to evaluate the extent to which claims for participation in AI reflect alignment between the stated goals and methods.
We recommend conceptualizing participation as a set of dimensions that must be explicitly specified at the project level. This means moving away from conceptualizing participation as an all-or-nothing characteristic of a project. Instead, we call for articulating how one’s goals for participation are aligned with the methods and scope of stakeholder input into AI design.