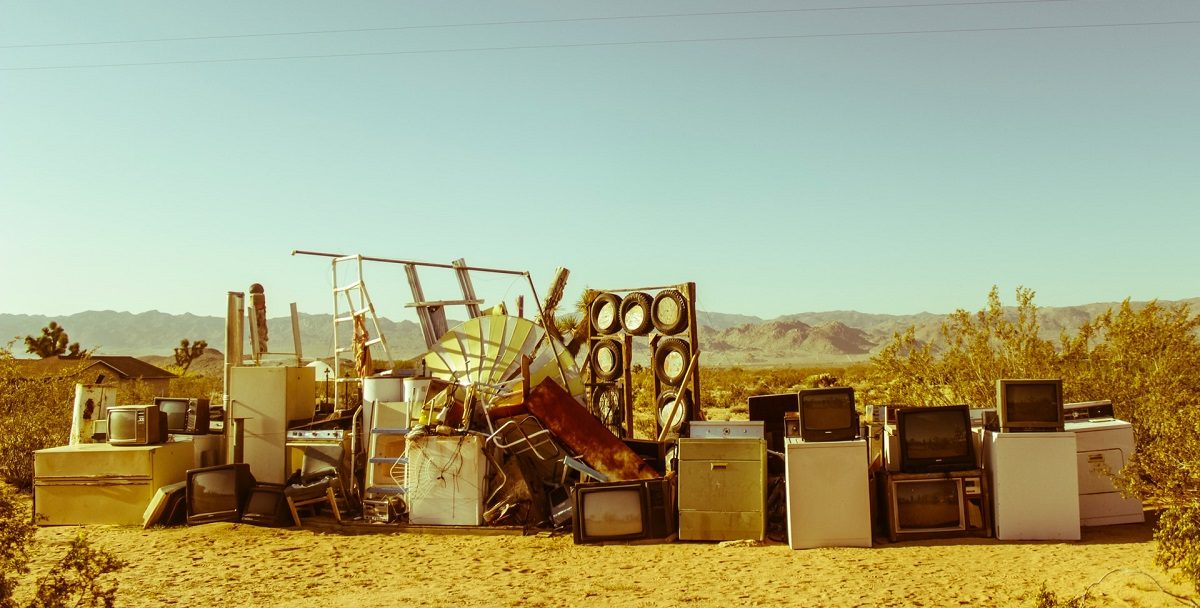
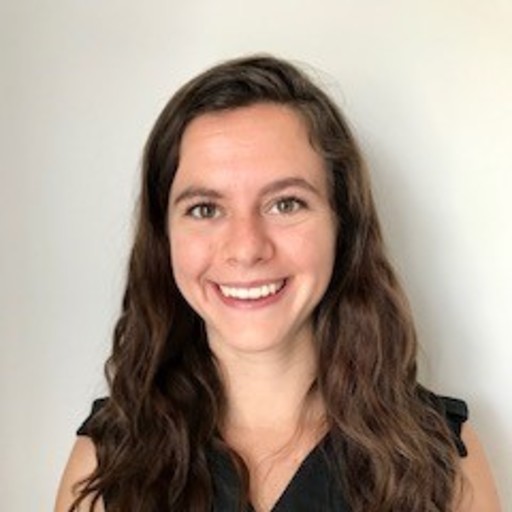
✍️ Column by Alexandrine Royer, our Educational Program Manager.
In mid-March, Netflix revealed the details behind our streaming consumption patterns that tally into the company’s carbon footprint. DIMPACT, a tool that calculates digital companies’ emissions, determined that one hour of streaming was equivalent to one ceiling fan running for four hours in North America or six hours in Europe. While such statistics do not seem shocking nor particularly alarming, they omit what we might be doing instead of spending an additional hour on our computer.
Streaming services also do not operate in the same way in a given environment as, say, a ceiling fan or a washing machine. For a more precise understanding of the environmental and social impacts of streaming, calculations ought to include the energy costs of charging our laptops to keep streaming, securing access to high-speed Internet, upgrading devices and discarding old hardware, and so on.
The widespread usage of streaming platforms shows how a single technological change produces ripple effects that modify our habits, our energy needs and our carbon footprint, even if they appear minute. As many anthropologists have argued, AI systems should be viewed as socio-technical systems instead of single bounded entities. The term further invites a holistic approach in understanding the social and environmental impact of having these algorithms run in our lives and what happens once they enter disuse.
Our inability to see and comprehend the lines of code behind the design of our favourite apps and platforms has helped foster the view of AI systems as operating in a virtual realm of their own. The invisibility of algorithmic systems contributes to the lack of transparency regarding the biases integrated within and integral to these systems. Kept away from peering eyes are the massive data centers required to keep these systems running and their polluting effects, leading us to quickly forget that tech is a double-edge sword capable of preventing and generating damage to the environment.
The implications of our long-term reliance on tech are often tinged with techno-dystopian discourses of artificial intelligence taking over and dispelling the end of the human race. Such alarmist views encourage a distorted view of AI systems’ current capacities and what is probable and possible. Instead, I argue for a more critical inquiry of the social and environmental effects of AI systems that follows each step of the systems’ life cycle and how they interact with previously existing structures along the way.
As highlighted by Eric Broda, ML systems’ lifecycle from tech companies such as Microsoft and Google is often presented through the same stages of data understanding/project objectives, acquiring and engineering data, model development and training and model deployment and monitoring. For Broda, the requirements of model reproducibility, traceability and verifiability tend to be omitted or underplayed in these AI/ML lifecycles. To this criteria, I would add the sustainability of AI systems, the long-term consequences of keeping these systems running and their likely expiration date within the tech space.
To better understand the shortcomings of AI systems, Broda suggests introducing a life cycle catalogue, a type of “book of record”, that “provides a viewport into the data science lifecycle” by allowing “data scientists to visualize, categorize, group, manage, and govern all of an enterprise’s data assets”. The lifecycle catalogue can be a valuable tool to estimate an AI system’s impacts from within its code to its outside connections to other existing systems and deployment into our lives. It can serve to provide visible ethical ‘checkpoints’ for legislators and citizens alike to understand the implications of each stage of the AI/ML process.
The catalogue also pushes us to reflect on what happens to the end of life of a system, knowing that systems cannot exist forever and that they must dispose of the masses of accumulated data. Scholars such as Edina Harbinja have already pushed for legislating and regulating post-mortem privacy to protect the trails of data that individuals have left behind through a lifetime of online activities. But beyond the individual level, little is known as to how companies dispose of their data (and perhaps for good security reasons). Just as importantly, few reports have addressed the consequences of the dismantling systems that people have come to rely on.
With the lifecycle catalogue mindset, we can return to our initial example of Netflix. Netflix streaming services will have accumulated precise personal information over viewers’ series and movie selection preferences throughout their deployment stage. If Netflix is suddenly unable to compete with other streaming service providers and must be discontinued, it will have to dispose of the masses of data on their users. Even if the data is anonymized, individuals can be easily traced back through cross-checking with user profiles on other public sites, such as IMDB.
Alongside these ethical privacy concerns are the environmental costs of keeping these systems running. In their continuous learning and monitoring phase, Netflix’s algorithms will be tweaked to incentivize viewers to keep watching for hours on end, increasing both the company’s computational power demands and the individual’s energy consumption. Individuals looking to improve their streaming experiences will be encouraged to acquire high-speed internet and devices with better image quality. Tracking the sustainability of AI systems throughout their lifecycle will require monitoring all these factors.
Thinking holistically about AI systems also involves thinking about their endpoint. AI as socio-technical systems can create a series of connections but also ruptures and breaks. How much do we know about AI systems that have been discarded? To frame this question otherwise, what might be resting in the AI junkyard? And what can we learn from it?