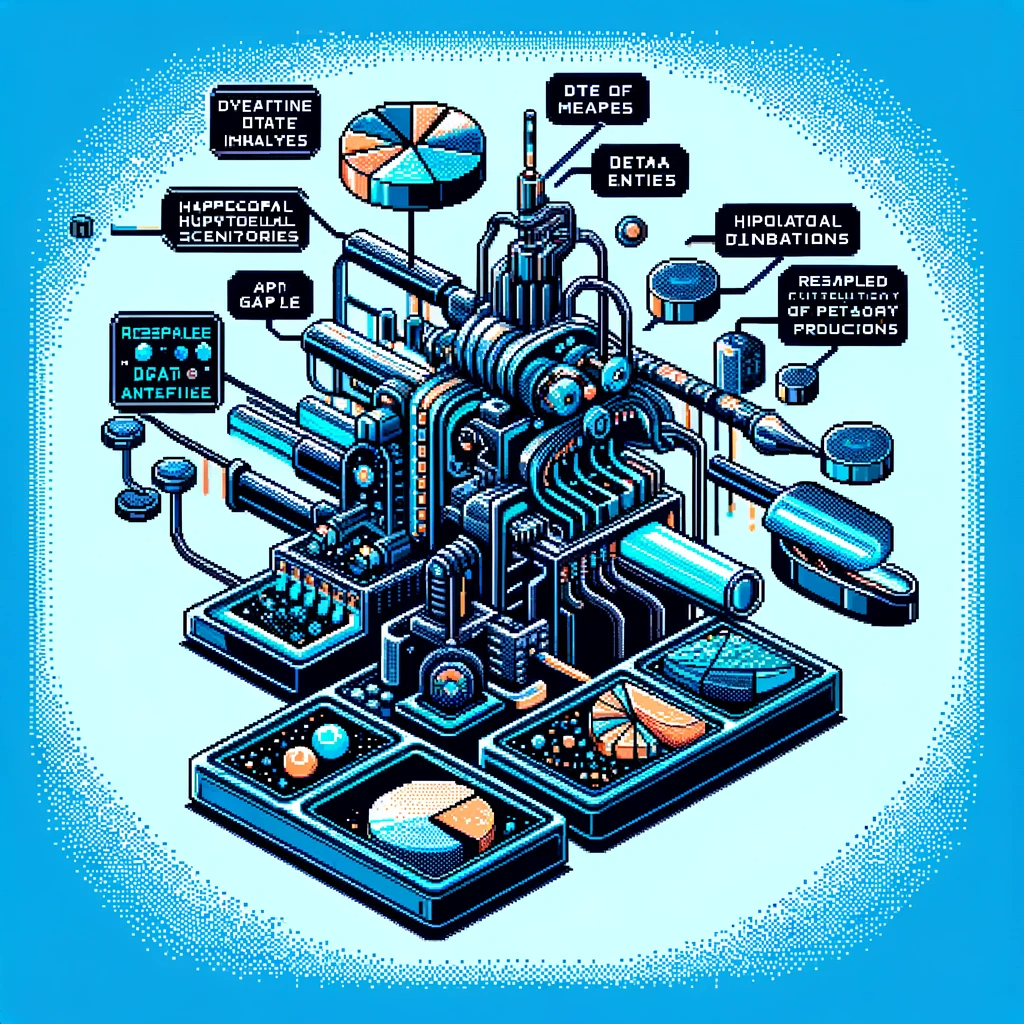
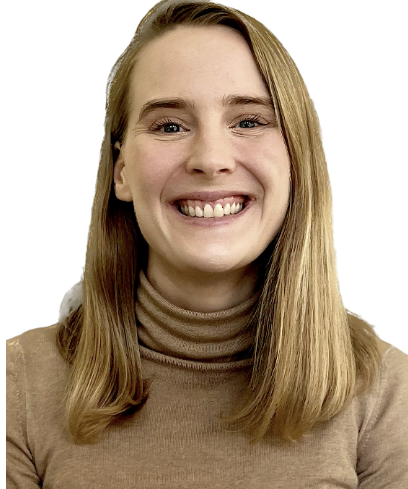
🔬 Research Summary by Jessica Echterhoff, a Ph.D. candidate in computer science at the University of California in San Diego. Her research focuses on human-data-centric artificial intelligence.
[Original paper by Jessica Echterhoff, Bhaskar Sen, Yifei Ren, and Nikhil Gopal]
Overview: What-if analysis is a process in data-driven decision-making to inspect the behavior of a complex system under some given hypothesis. This paper proposes a What-If Machine that resamples existing data distributions to create hypothetical scenarios and measure their impact on a target metric for data-driven decision-making such that hypotheses can be made and quickly evaluated.
Introduction
In the realm of decision-making, uncertainty is an ever-present factor. Organizations and individuals alike are often faced with complex choices that can have far-reaching consequences. In this context, the “what-if” hypothetical scenario analysis technique emerges as a valuable tool for navigating this uncertainty and aiding data-informed decisions. “What-if” analysis refers to the analysis of a possibility. It measures its impact if it were to be implemented, e.g., “What if we opened another branch at location Y? How would it impact our revenue?”. By exploring various potential outcomes under different conditions in the data, this approach can provide a structured framework for evaluating options and mitigating risk.
The paper presents a versatile tool based on Bayesian Optimization and Monte-Carlo simulation that addresses the dynamic landscape of data-driven decision-making. The “What-If Machine” achieves small errors on real-world hypotheses and enables quick data-driven hypothesis confirmation/rejection to speed up the data science pipeline and automatically reveal potential high-impact areas. The tool accelerates the exploration of various possibilities by automating the process of generating “what-if” questions, providing real-time means of decision support. Simultaneously, the tool is an asset for practitioners seeking to evaluate their intuitions against data-driven insights, promoting a synergistic balance between human expertise and automated analytics.
Key Insights
Design Implications
On the one hand, there is a need for a tool for quickly confirming/disproving a hypothesis that was built with an expert’s domain knowledge with underlying data, as evidence-based decisions can improve organizational performance. On the other hand, developing an understanding of what problem should be solved in data science can be a complex and difficult process. Data scientists and decision makers such as program managers often make their decisions based on heuristics or analysis of one scenario at a time. Practitioners might also get stuck in established thought patterns due to cognitive biases. These insights sparked the initial idea of a tool to provide immediate feedback on the impact of hypothetical scenarios, as well as provide the most promising possibilities. Based on the available literature, we develop the design implications hypothesis confirmation/rejection (a tool that should enable both quick evaluations of existing hypotheses) and hypothesis generation (a tool that should give a broad overview of impactful possibilities).
Methods & Usage Scenario
Both hypothesis confirmation/rejection and hypothesis generation rely on a similar underlying algorithmic idea: resample the historical data distribution using Monte-Carlo Sampling to reflect a hypothetical scenario and report the impacts on a target metric. For example, consider a hypothetical scenario for product manager Jamie, whose task is to develop and prioritize ideas and intuitions about their data on power outages. Jamie’s task could be to evaluate ways to reduce power outages in the USA and prioritize them for future planning. For this task, Jamie has access to power outage data between 2000 and 2014, which includes different causes for the outages and the impact on customers given by the number of customers affected and time to restore electricity. Jamie is tasked to develop ideas to reduce the number of outages, time to restore electricity, or customer impact as a target metric. Our work can help Jamie to evaluate his intuition that vandalism caused a decent number of outages or automatically give insights such as the impact of severe weather on the time to restore power, which could mean that if he increased the focus on making the infrastructure more resilient to weather conditions, customers could have their energy outages restored more quickly.
Between the lines
Explainability and interpretability of decision-making systems are ongoing open topics in artificial intelligence. This work offers an attempt at an alternative to black-box algorithms, using only existing historical data to gather insights into different scenarios. The advantage is that by only relying on historical data, there is no doubt about the existence of the events that occurred and their effects, hence limiting uncertainty in the work with automated models. Future work can extend the current system by adding more features that make it more broadly applicable to different use cases (e.g., by extending it to multi-dimensional analysis) or even include historical findings to explain future predictions to decrease uncertainty for the human decision-maker.