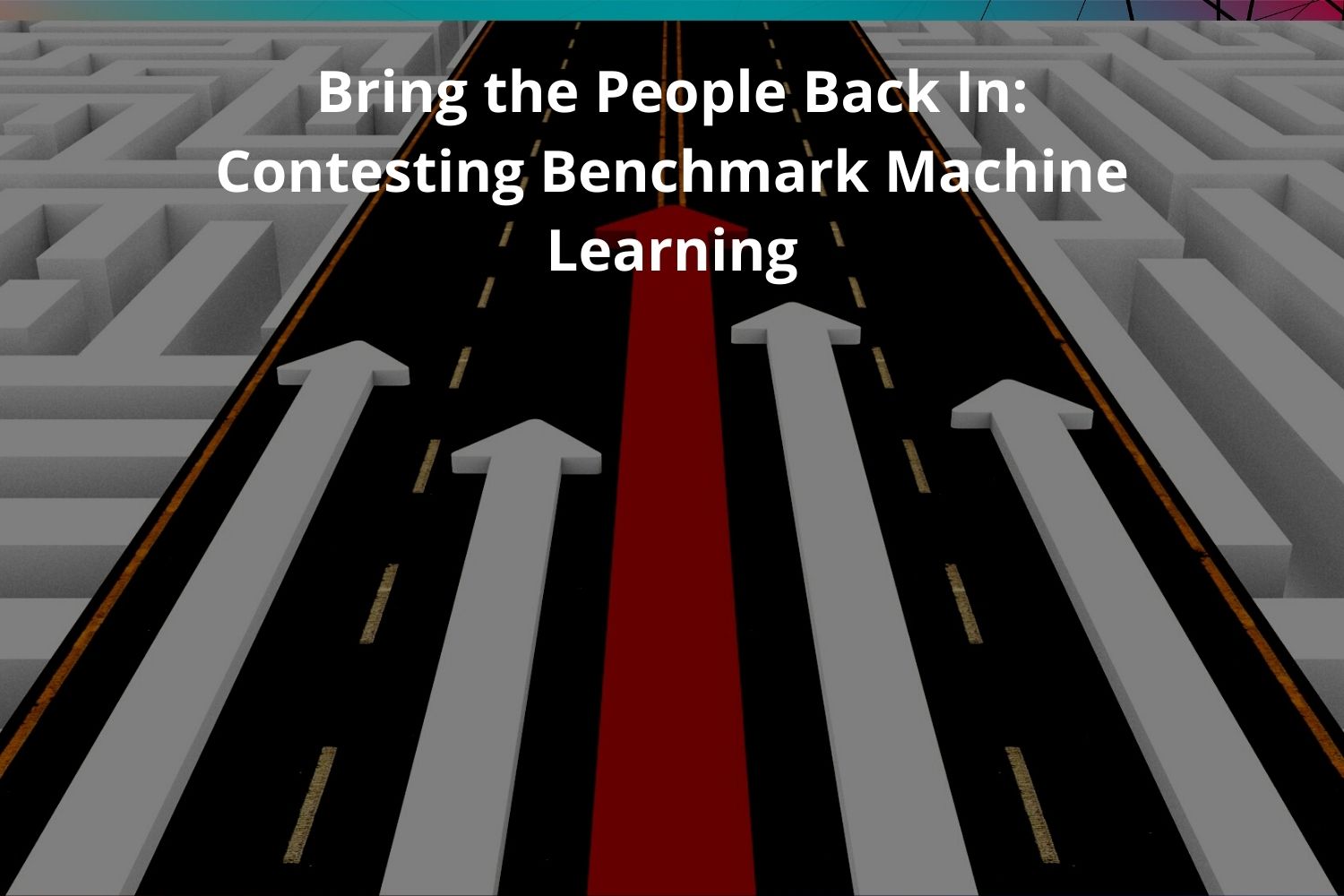
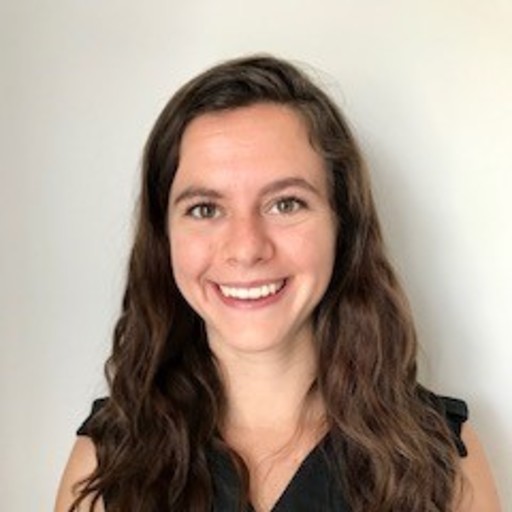
Summary contributed by our researcher Alexandrine Royer, who works at The Foundation for Genocide Education.
*Authors of full paper & link at the bottom
Mini-summary: The biases present in machine learning datasets, which revealed themselves to favour white, cisgender, male and Western subjects, have received a considerable amount of scholarly attention. Denton et al. argue that the scientific community has failed to consider the histories, values, and norms that construct and pervade such datasets. The authors intend to create a research program, what they termed the genealogy of machine learning, that works to understand how and why such datasets are created. By turning our attention to data collection, and specifically the labour involved in dataset creation, we can “bring the people back in” the machine learning process. For Denton et al., understanding the labour embedded in the dataset will push researchers to critically reflect on the type and origin of the data they are using and thereby contest some of its applications.
Full summary:
In recent years, industry and non-industry members have decried the prevalence of biased datasets against people of colour, women, LGBTQ+ communities, people with disabilities, and the working class within AI algorithms and machine learning systems. Due to societal backlash, data scientists have concentrated on adjusting the outputs of these systems. Fine-tuning algorithms to achieve “fairer results” have prevented, according to Denton et al., data scientists from questioning the data infrastructure itself, especially when it comes to benchmarks datasets.
The authors point to how new forms of algorithmic fairness interventions generally center on the parity of representation between different demographic groups within the training datasets. They argue that such interventions fail to consider the issues present within data collection, which can involve exploitative mechanisms. Academics and industry members alike tend to disregard the question of why such datasets are created. Factors such as what and whose values are determining the type of data collected, in what conditions are the collection being done, and whether standard data collection norms are appropriate often escape data scientists. For Denton et al., data scientists and data practitioners ought to work to “denaturalize” the data infrastructure, meaning to uncover the assumptions and values that underlie prominent ML datasets.
Taking inspiration from French philosopher Michel Foucault, the authors offer the first step what they termed the “genealogy” of machine learning. For a start, data and social scientists should trace the histories of prominent datasets, the modes of power as well as the unspoken labour that went into its creation. Labelling within datasets is organized through a particular categorical schema, but it is seen as widely applicable, even for models with different success metrics. Benchmarking datasets are treated as gold standards for machine learning evaluation and comparison, leading them to take on an authoritative status. Indeed, as summarized by the authors, “once a dataset is released and established enough to seamlessly support research and development, their contingent conditions of creation tend to be lost or taken for granted.”
Once datasets achieve this naturalized status, they are perceived as natural and scientific objects and, therefore, can be used within multiple institutions or organizations. Publicly available research datasets, constructed in an academic context, often provide the methodological backbone (i.e. infrastructure) for several industry-oriented AI tools. Despite the disparities in the amount of data collected, industry machine learners will still rely on these datasets to undergird the material research in commercial AI. Technological companies treat these shifts are merely changes in scale and rarely in kind.
To reverse the taken-for-granted status of benchmark datasets, the authors offer four guiding research questions:
- How do datasets developers in machine learning research describe and motivate the decisions that go into their creation?
- What are the histories and contingent conditions of the creation of benchmark datasets in machine learning? As an example, the authors offer the case of Henrietta Lacks, an Afro-American woman whose cervical cancer cells were removed from her body without her consent before her death.
- How do benchmark datasets become authoritative, and how does this impact research practice?
- What are the current work practices, norms, and routines that structure data collection, curation, and annotation of data in machine learning?
The research questions offered by Denton et al. are a good start in encouraging machine learners to think critically as to whether their dataset is aligned with ethical principles and values. Any investigation into the history of science will quickly reveal how data-gathering operations are often part of predatory and exploitative behaviours, especially towards minority groups who have little recourse to contest these practices. Data science should not be treated as an exception to this long-standing historical trend. The creators of data collection should merit as much ethical consideration as the subjects that form this data. By critically investigating the work practices of technical experts, we can begin to demand greater accountability and contestability in the development of benchmark datasets.
Original paper by Emily Denton, Alex Hanna, Razvan Amironesi, Andrew Smart, Hilary Nicole, Morgan Klaus Scheuerman: https://arxiv.org/abs/2007.07399