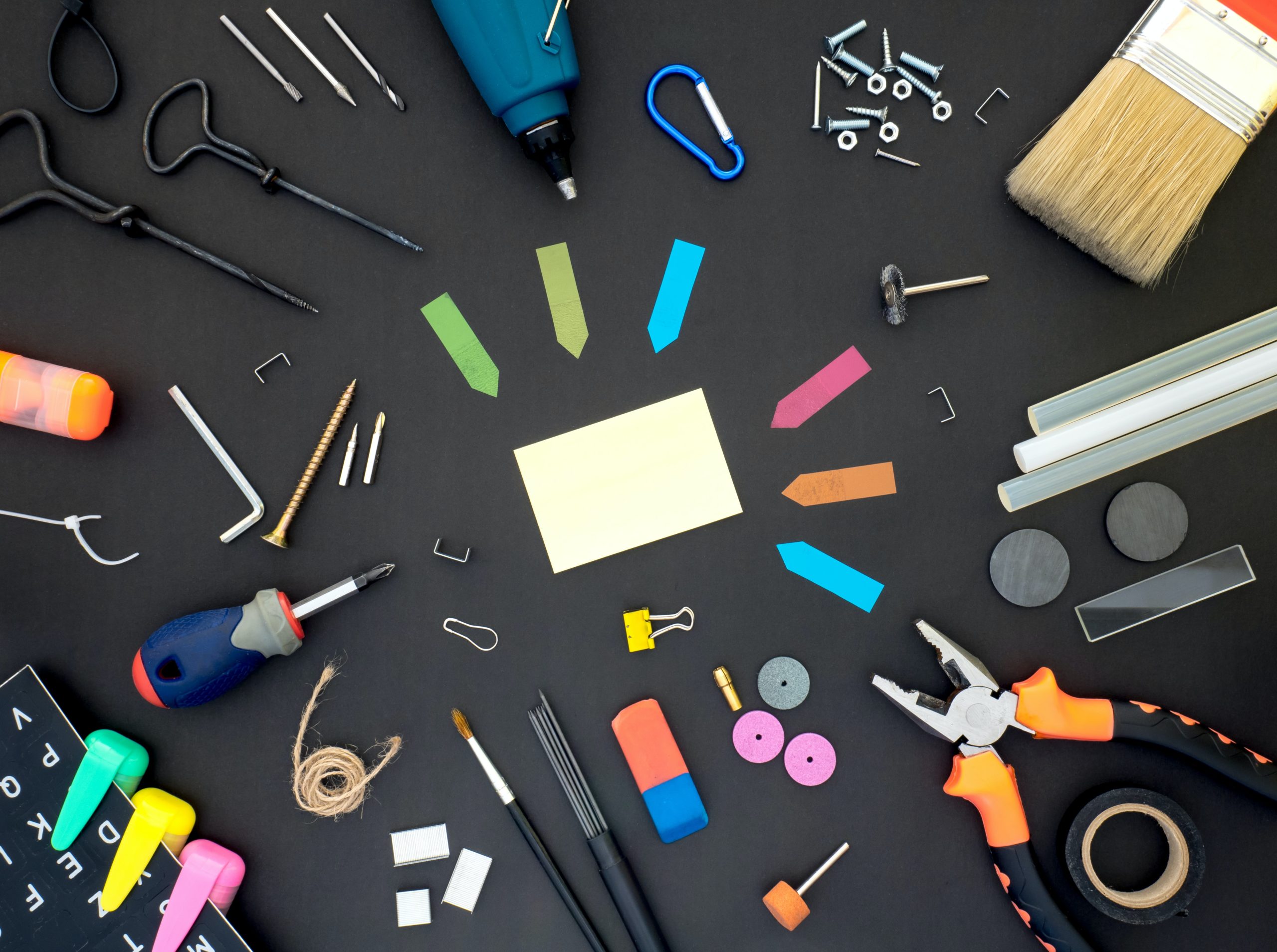
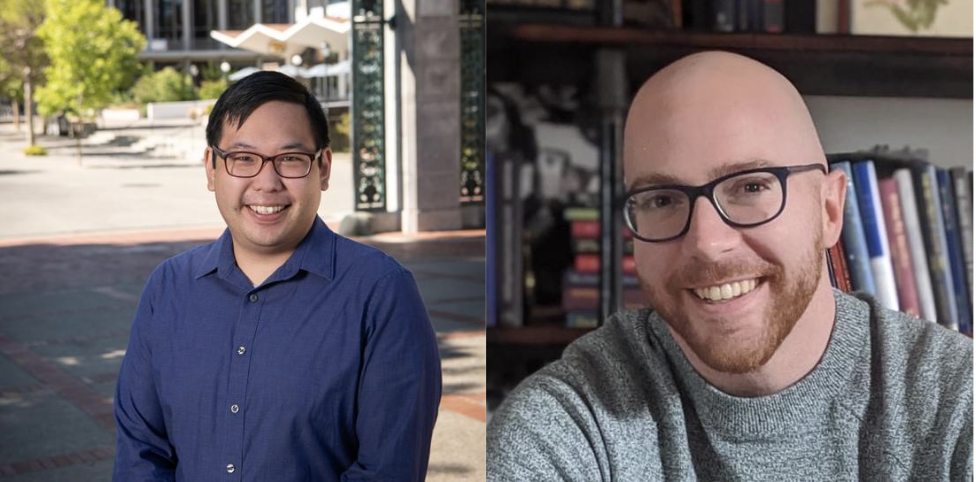
🔬 Research Summary by Richmond Y. Wong and Michael A. Madaio.
Richmond Wong is a Postdoctoral Researcher at the University of California Berkeley Center for Long-Term Cybersecurity, where he studies how technology professionals address social values and ethical issues in their work.
Michael Madaio is a postdoctoral researcher at Microsoft Research, where his research is at the intersection of HCI and FATE (Fairness, Accountability, Transparency, and Ethics) in AI.
[Original paper by Richmond Y. Wong, Michael A. Madaio, Nick Merrill]
Overview: Numerous toolkits have been developed to support ethical AI development. However, ethical AI toolkits, like all tools, encode assumptions in their design about what the work of “doing ethics” looks like—what work should be done, how, and by whom. We conduct a qualitative analysis of AI ethics toolkits to examine what their creators imagine to be the work of doing ethics, and the gaps that exist between the types of work that the toolkits imagine and support, and the way that the work of ethical AI actually occurs within technology companies and organizations.Â
Introduction
Recent controversies have highlighted how the creation, adoption, and deployment of AI systems can cause harm to humans: from controversies over uses of facial recognition in surveillance, to algorithmic decision making in schools, to image recognition in medical contexts. In response, technology developers, researchers, policymakers, and others have identified the design and development process of AI systems as a site for interventions to promote more ethical and just ends for AI systems. Recognizing this opportunity, researchers, practitioners, and activists have created a plethora of tools, resources, guides, and kits to promote ethics in AI design and development—of which the dominant paradigm is a “toolkit”.
However, as the field appears to coalesce around this paradigm, it is critical to ask how these toolkits help to define and shape the work of AI ethics? Specifically, we conduct a qualitative analysis of 27 AI ethics toolkits to ask:
- Who do the toolkits imagine as doing the work of addressing ethics in AI?
- What do toolkits imagine to be the specific work practices of addressing ethics in AI?
We outline several salient findings in the following sections (with more detail on findings and methods in our paper).
Key Insights
Who do Toolkits Envision as Doing AI Ethics?
The toolkits we analyzed mention several types of potential users of those tools, often based on their job categories or roles within technology companies: software engineers; data scientists; cross-functional, cross disciplinary teams; risk or internal governance teams; C-level executives; board members. When a toolkit envisions a specific stakeholder or job role as doing the work of addressing AI ethics via that toolkit, it also suggests a particular worldview—particular skills, tools, knowledge, and assumptions—that should be used to address AI ethics.
For instance, toolkits that envision their users as those in engineering and data science roles often focus on ethics as the practical, humdrum work of creating engineering specifications and then meeting those specifications. In contrast, for C-level executives and board members, toolkits frame ethics as both a business risk and a strategic differentiator in a crowded market. For instance, one Responsible AI guide states, “Sustainable innovation means incentivizing risk professionals to act for quick business wins and showing business leaders why fairness and transparency are good for business.”
Of course, the primary type of users envisioned by many AI ethics toolkits are members of AI design and development teams across a variety of roles. The next question then (borrowing language from Anna Lauren Hoffman, who in turn channels Sara Ahmed), is what are the “terms of inclusion” for each of these types of stakeholders within technology companies? On what terms do these stakeholders get to participate in the work of AI ethics?
In large part, toolkits appear to provide technical-oriented tooling that envisions technical users who contribute directly to production codebases. While these toolkits may rhetorically describe non-technical staff as important to the conversation, they often do not provide concrete resources or processes for other types of skills and knowledge to contribute, such as those skilled in social sciences and human-centered practices.
The rhetoric of AI ethics toolkits is one of collaboration—between cross-functional teams (comprised of different roles), between C-suite executives and tech labor, and between stakeholders both internal and external to the organization. But no toolkit quite specifies how this collaboration should be enacted in practice, nor do they provide concrete resources for this interdisciplinary or cross-functional collaboration. In addition, toolkits rarely acknowledge or provide resources to address the social power differentials between workers and executives, or tech workers and external stakeholders. Even those rare toolkits that do acknowledge social power as a factor under-specify how this power should be dealt with.
The same patterns repeat when considering toolkits’ engagement with broader communities and stakeholders external to companies producing AI systems. This group includes clients, vendors, customers, users, civil society groups, journalists, advocacy groups, community members, and others impacted by AI systems. These stakeholders are imagined as outside the organization in question. However, the work these external stakeholders are imagined to do in these circumstances is under-specified. Their specific roles are under-imagined, relegated to the vague “raising concerns” or “providing input” from “on-the-ground perspectives.”
What Work Practices do AI Ethics Toolkits Envision?
We find that there are often gaps between the types of claims that the toolkits make, and the types of work practices they promote.
Most toolkits focus on technical work with ML models, in specific workflows and tooling suites, despite claims that fairness is sociotechnical. In practice, this means that tools’ suggested uses are oriented around the ML lifecycle, often integrated into specific ML tool pipelines. This emphasis on technical functionality offered by the toolkits, as well as the fact that many are designed to fit into ML modeling workflows and tooling suites suggests that non-technical stakeholders (whether they are non-technical workers involved in the design of AI systems, or stakeholders external to technology companies) may have difficulty using these toolkits to contribute to the work of ethical AI. In this envisioned work, what role is there for social scientists, for UX and user researchers, for domain experts, or for people impacted by AI systems, in doing the work of AI ethics?
Many toolkits call for engagement with stakeholders external to the team or company but provide little guidance on how. Toolkits suggest that stakeholders and impacted communities can help inform development teams about potential ethical impacts, and can be useful groups for the AI design team to inform and communicate about ethical risks. However, there is little guidance provided by the tools on how to do this. Imagined roles for external stakeholders are generally framed as either providing domain information or being recipients of information from developers. These forms of engagement may actually be disempowering, as they do not provide external stakeholders with a meaningful ability to shape systems’ designs. Furthermore, the technical orientation of many toolkits may preclude meaningful participation by non-technical stakeholders.
As framed by the toolkits, the work of ethics is often imagined to be done by individual data scientists or ML teams, both of whom are imagined to have the power to influence key design decisions, without considering how organizational power dynamics may shape those processes. For many toolkits, individuals within the organization are envisioned to be the catalysts for change via oaths or individual self-reflection exercises. In other cases, the implicit theory of change involves product and development teams having conversations, which are then thought to lead to changes in design decisions towards more ethical design processes or outcomes.
More generally, the toolkits enact some form of solutionism—the belief that ethical issues that may arise in AI design can be solved if individuals and teams use the right tool or process (typically the approach proposed by that toolkit). However, individual workers or teams may not have enough power to drive meaningful change at either a product or organizational level. In fact, despite many toolkits’ claims to empower individual practitioners to raise issues, toolkits largely appeared not to address fundamental questions of worker power and collective action.
Between the lines: Implications for AI Ethics Toolkit Design
Among all toolkits, we identify a mismatch between the imagined work of ethics and the support they provide for doing that work. While we find shortcomings in the current approaches of AI ethics toolkits, we do not think they need to be thrown out wholesale. Practitioners will continue to require support in enacting ethics in AI, and toolkits are one potential approach to provide such support, as evidenced by their ongoing popularity. Our findings suggest three concrete recommendations for improving toolkits’ potential to support the work of AI ethics.
- Toolkits should embrace the non-technical dimensions of AI ethics work. Despite emerging awareness that fairness is sociotechnical, the majority of toolkits provided resources to support technical work practices. Embracing non-technical dimensions of AI ethics work might entail resources to support understanding the theories and concepts of ethics in non-technical ways. For instance, toolkit designers might incorporate methods from qualitative research, user research, or value-sensitive design. As a precursor to this, practitioners may need support in identifying the stakeholders for their systems and use cases, in the contexts in which those systems are (or will be) deployed. Approaches such as stakeholder mapping from fields like Human-Computer Interaction may be useful here, and such resources may be incorporated into AI ethics toolkits.
- Toolkits should support engagement with stakeholders from non-technical backgrounds. Although many toolkits call for engaging stakeholders from different backgrounds and forms of expertise, the toolkits themselves offer little support for how their users might bridge disciplinary divides. Toolkits should support this translational work. This might entail, for instance, asking what fairness means to the various stakeholders implicated in ethical AI, or communicating the output of algorithmic impact assessments (e.g., various fairness metrics) in ways that non-technical stakeholders can understand and work with.Â
- Toolkits should structure the work of AI ethics as a problem for collective action. One aspect we found missing in the toolkits was support for stakeholders (particularly those working in technology companies) in grappling with the organizational dynamics involved in doing the work of ethics. Toolkits should structure ethical AI as a problem for collective action for multiple groups of stakeholders, rather than work for individual (technical) practitioners. This perspective may entail supporting collective action by workers within tech companies, or fostering communities of practice of professionals working on ethical AI across institutions (to share knowledge and best practices, as well as shift professional norms and standards), or supporting collective efforts for ethical AI across industry professionals and communities impacted by AI. This might involve support for helping practitioners communicate to organizational leadership and advocating for the need to engage in ethical AI work practices, or advocating for additional time or resources to do this work. This might also involve providing support for organizing collective action in the workplaces, such as unions, tactical walkouts, or other uses of labor power based on their role in technology production.