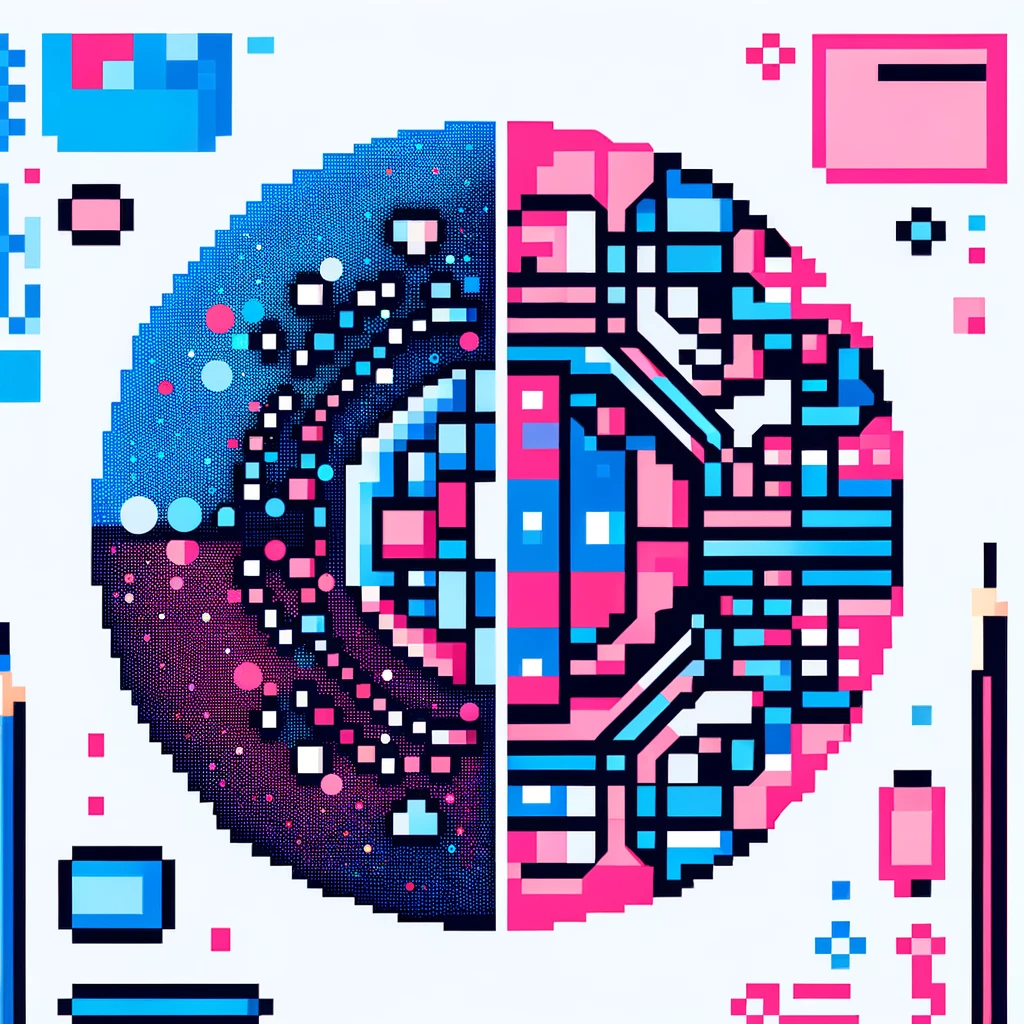
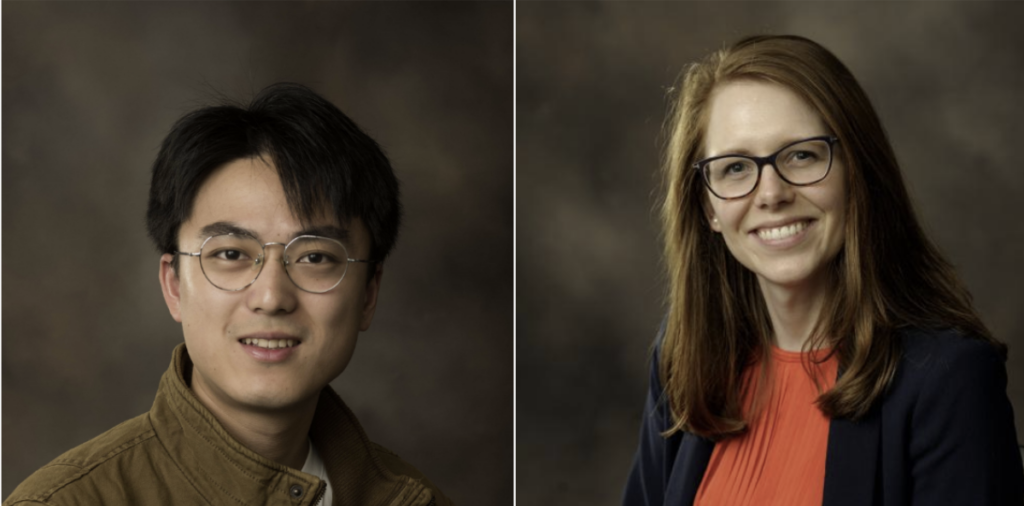
🔬 Research Summary by Kyrie Zhixuan Zhou and Madelyn Rose Sanfilippo.
Kyrie Zhixuan Zhou is a PhD student at the University of Illinois at Urbana-Champaign, aiming to understand, design, and govern ICT/AI experience for vulnerable populations.
Madelyn Rose Sanfilippo is an Assistant Professor at the University of Illinois at Urbana-Champaign, studying the governance of sociotechnical systems and outcomes, inequality, and consequences within these systems.
[Original paper by Kyrie Zhixuan Zhou and Madelyn Rose Sanfilippo]
Overview: Two of the world’s most prominent large language models (LLMs), ChatGPT (US) and Ernie (China), reflect gender bias. In this paper, we qualitatively analyze and compare social media discussions and identify different narrative patterns, e.g., users more often complain about “implicit” bias in ChatGPT. In contrast, Ernie is said to contain “explicit” concerning biases. We propose governance recommendations to regulate gender bias in LLMs.
Introduction
LLMs are quickly gaining momentum yet demonstrate gender bias in their responses. We conducted a content analysis of social media discussions to gauge public perceptions of gender bias in LLMs, which are trained in different cultural contexts, i.e., ChatGPT, a US-based LLM, or Ernie, a China-based LLM. People shared both observations of gender bias in their personal use and scientific findings about gender bias in LLMs. A difference was identified in perceptions of the two LLMs – ChatGPT was more often found to carry implicit gender bias, e.g., associating men and women with different profession titles, while explicit gender bias was found in Ernie’s responses, e.g., overly promoting women’s pursuit of marriage over career. Based on the findings, we reflect on the impact of culture on gender bias and propose governance recommendations to address gender bias in LLMs.
Key Insights
Motivation and Methodology
Gender bias has long been studied in computer systems and other domains. Gender bias in LLMs such as ChatGPT could harm half the global population if it is widely adopted. Understanding public perceptions toward gender bias in LLMs is crucial to ensuring policies and regulations are relevant and effective in meeting people’s needs. In the meantime, LLMs are trained on data collected from search engines, online forums, websites, and so on. Thus, LLMs can reflect and even amplify existing biases in human language. Social biases exist in varying forms in different cultures. LLMs trained on data collected from different cultures may also demonstrate different types and levels of biases. To understand how gender bias manifests in LLMs rooted in different cultures, we examined ChatGPT by OpenAI, based in the US, and Ernie by Baidu, based in China, for a comparative case study toward informing contextual and concrete governance mechanisms, including new regulations and adaptations of existing laws.
We approached public perceptions about gender bias in ChatGPT and Ernie by comparing social media discussions around these two LLMs. Social media discussions about ChatGPT were obtained via the search query “gender bias in chatgpt” from Twitter. Similarly, we searched with the Chinese query “gender bias in Ernie” on Sina Weibo. Our data collection covered nine months of discussions about ChatGPT and six months about Ernie. We collected and analyzed the data simultaneously and stopped searching for more discussions after reaching a theoretical saturation in the analysis, i.e., no new themes emerged. However, we constantly looked for recent discussions in case new perceptions or concerns arose. A thematic analysis approach was adopted to analyze the online discussions.
Findings and Impact
The main findings from the discussion about ChatGPT included (1) observation of implicit gender bias (e.g., associating gender with different profession titles), (2) political correctness (e.g., refusing to tell jokes about women), (3) dissemination of scientific findings regarding LLM bias, and (4) call for action (e.g., an educator promoting fairness in her lessons).
The main findings from the discussion about Ernie included (1) observation of explicit gender bias (e.g., “women had better get married at a young age”), (2) criticism of culture underlying the training data, and (3) a lack of discussion on gender bias in Ernie, possibly due to the censorship on the social media platform where the discussion took place.
Our comparative analysis of gender bias in ChatGPT and Ernie sheds light on the intertwining relationship between culture and LLM/AI gender bias. Further, we propose governance recommendations to regulate gender bias in LLMs (more details in the paper), including (1) creating concrete and contextual policies, (2) applying various existing legal precedents to protect at-risk populations, and (3) forming new norms to mitigate emergent bias in or discrimination by AI.
Between the lines
An important takeaway from our research is that cultural factors impact biases in LLMs since they were trained on data that reflect those cultural contexts. Regulation should thus be contextual and concrete instead of “one-size-fits-all.” Further, we want to inspire norm formation in terms of building non-discriminatory AI – Industrial practices that have built upon social norms to protect user privacy can partly be attributed to legislation around privacy, such as GDPR and enforcement actions against the actors who refused to comply, such as Facebook (Meta). Norms to mitigate discrimination in AI systems might be similarly formed with legislation efforts and by engaging and educating users. The paper discusses several concrete ideas for future research, especially regarding regulation and policy-making. Ultimately, we hope our findings will inspire more interesting, interdisciplinary research in AI ethics and computational social science.