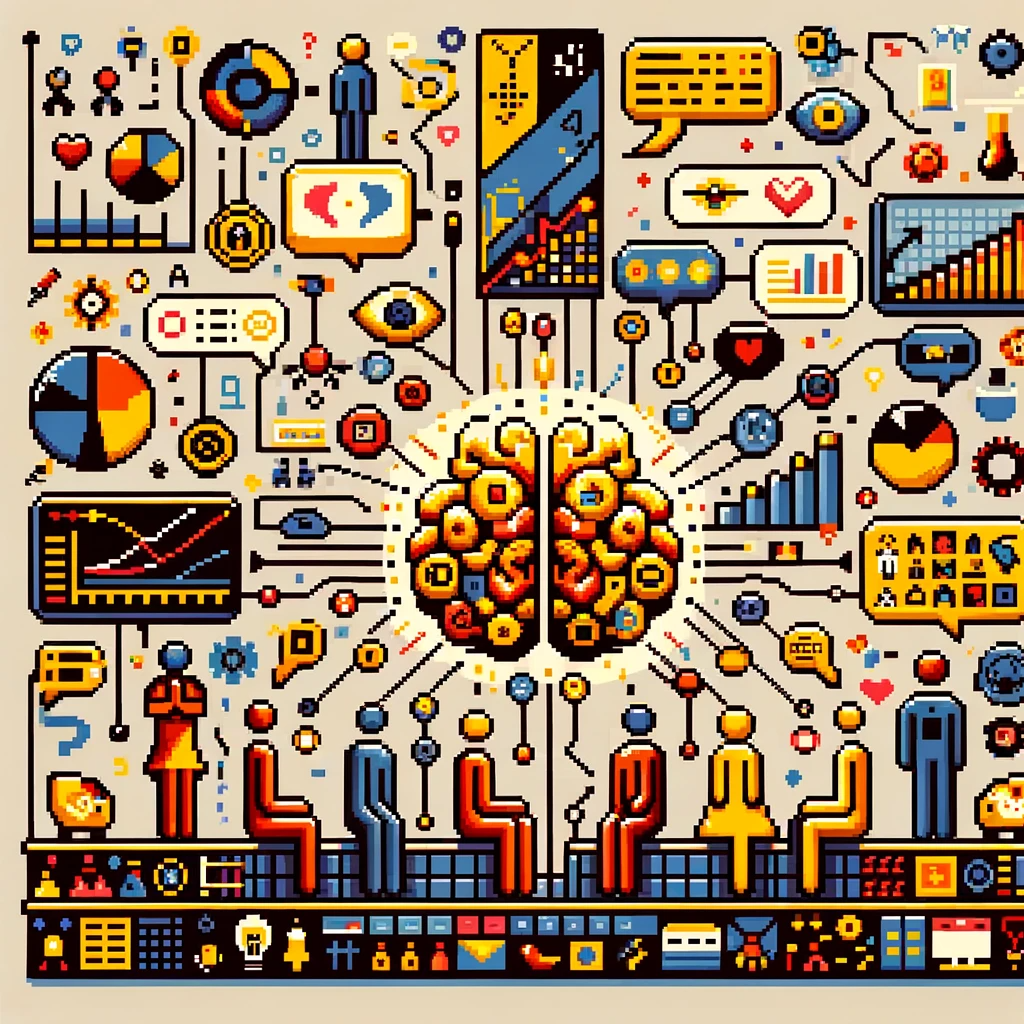
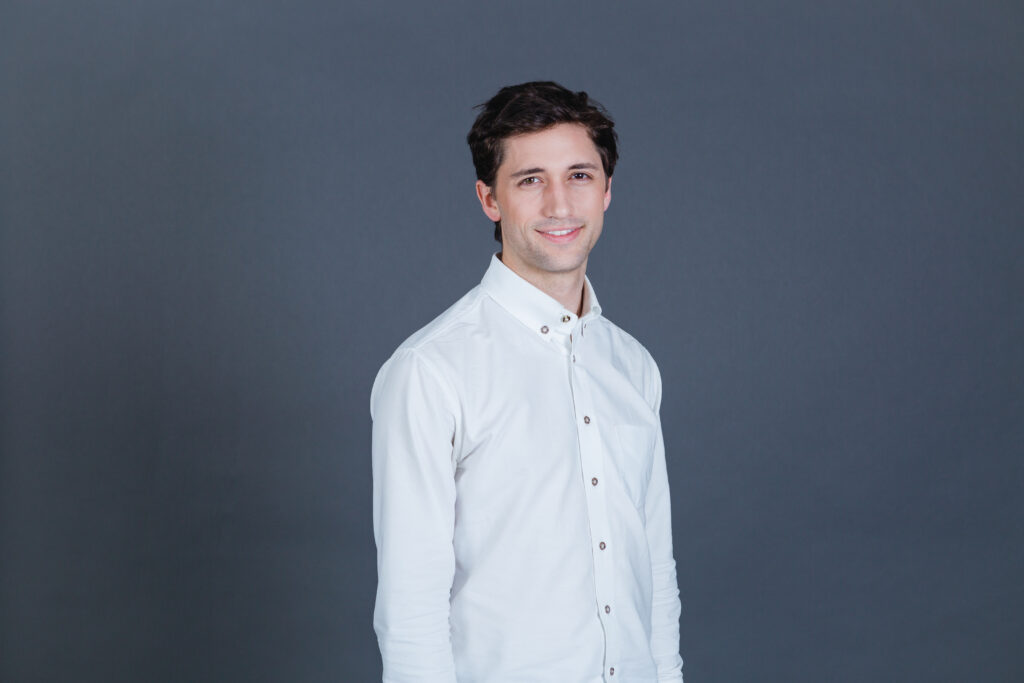
🔬 Research Summary by Simon Hudson , a writer and researcher investigating subjects in AI governance, human-machine collaboration, and Science Communications, and is currently co-leading the core team behind Botto, a decentralized autonomous artist.
[Original paper by Simon Hudson and Matija Franklin]
Overview: Artificial Intelligence (AI) has a communication problem. XAI methods have made AI more understandable and helped resolve some of the transparency issues that inhibit AI’s broader usability. However, user evaluation studies reveal that the often numerical explanations provided by XAI methods have not always been effective for many types of users of AI systems. This article aims to adapt the major communications models from Science Communications into a framework for practitioners to understand, influence, and integrate the context of audiences both for their communications supporting AI literacy in the public and in designing XAI systems that are more adaptive to different users.
Introduction
How “AI literate” should a user be to effectively and responsibly use AI? With the rising integration of AI in various software products, users from various backgrounds encounter the technology daily. However, many grapple with the challenge of understanding these systems due to the lack of transparency. The emergence of Explainable Artificial Intelligence (XAI) has attempted to make AI’s decisions and predictions more transparent. Still, XAI models lean towards numerical explanations, which may not always resonate with everyone. Making AI and its explanations more adaptable to the diverse user base of AI is an important and difficult communication challenge.
Because communications about AI can impact how a user sees and works with technology, then it effectively changes the environment technology designers are building for. Technology experience can also impact how one sees technology and the lens through which they receive future communications about tech. We propose a framework that applies different communication models from the field of Science Communications (SciComms) and attempts to address the wholeness of this communication challenge to enable technology creators to be more sensitive to the many contexts in which their products may be used.
Key Insights
AI’s Communication Challenge
Getting people to effectively use Artificial Intelligence (AI) has been seen as a problem of AI literacy – ensuring users have a solid grasp of how the tech works. Efforts to address this have mainly used an “information-deficit model” in the field of Science Communications (SciComms). The information-deficit model is typically a top-down, one-way communication approach that
relies on disseminating the knowledge of experts to the broader public. Even if the ideas are simplified, they tend to ignore users’ individual contexts and local knowledge and instead favor a one-size-fits-all approach. These efforts tend to fail to impact the overall literacy of a field due to not adapting to the audience’s various perspectives or being made relevant in a way they can find helpful in their daily lives. The result is that many audiences are left behind in both the societal discussion about governing AI and how to make the tools beneficial for them in their respective lives. SciComms has favored new models that emphasize understanding an audience’s context, valuing local knowledge, and encouraging participatory involvement.
With recent advances in generative AI, there’s an opportunity to mesh SciComms models into XAI, making AI explanations more adaptive to individual user contexts. These challenges can be grouped into user and creator categories. For users, the emphasis is on setting reasonable expectations about AI through literacy efforts that are accessible and meaningful to local contexts. For creators, the focus is on designing adaptable AI systems and ensuring these systems can learn and adjust to individual user contexts.
To address AI’s communication challenges, it’s crucial to understand the contexts of various stakeholders and let them provide meaningful input. A three-stage framework is proposed to address these issues: Understanding Context, Influencing Context, and Integrating Context.
Understanding Context
The first stage in addressing AI’s communication challenge is grasping the user’s context. Knowing the user’s background, familiarity with AI, cognitive style, and information needs is vital. Methods from SciComms, such as audience research, psychographics, and user testing, can offer insights. There is rich documentation of the varying attitudes in self-driving vehicles (SDVs) studies that reveal how sociodemographics and their varying historical experiences with past technology innovations can shape perceptions of new technology.
We are considering a broad definition of context involving various factors influencing how information is received, interpreted, and integrated or discarded. Put simply, it considers the audience’s diverse needs to reach a communication goal. It goes beyond a communicator only considering the information that they want to be known by the audience.
Influencing Context
After understanding the user’s context, the next step is shaping the communication. Framing, a SciComms technique, helps prioritize different issues and consequences presented in communications so that the audience will find the material relevant to their lives. However, framing should be unbiased and focus on making the topics relevant to the audience so they can form their own opinion.
2-way dialogues are a 2-for-1. Making focus groups where experts engage with a particular community can be particularly effective for understanding context while building trust in the community because they feel (and are) heard. The closer the community gets to influencing the governance process of priorities and policy, the more trust can be built and new contexts formed. These new contexts can underpin more productive stakeholder engagement and equitable technology outcomes.
Integrating Context
The final stage mimics the first two parts of the framework within the design of the technology itself: ask questions to understand and influence through framing adjustments and two-way dialogue to get a better direction. This integration allows the AI system to learn from user interactions and modify its explanations. Approaches like adaptive communication enable AI to adjust explanations based on user feedback. The challenge is to make this interaction engaging, avoid overwhelming users, and continuously iterate based on feedback to ensure effective communication.
Between the lines
Generalized systems that are not adaptive to different contexts miss out on valuable local knowledge in exchange for systems that undermine user agency in the name of user-friendliness. The AI field, particularly XAI, needs to gain recognition of the interdependence of communications and technology design that makes user expectations and abilities a moving target.
We are at a very early starting point to get to new approaches for adaptable XAI. Major gaps are in computational approaches to mapping good science communications principles that can be integrated into fundamental model design, as well as better generative models in general that are less error-prone. But, following our framework can also help soften the negative impacts of machines presented as all-knowing when, in fact, they need careful human direction and scrutiny.