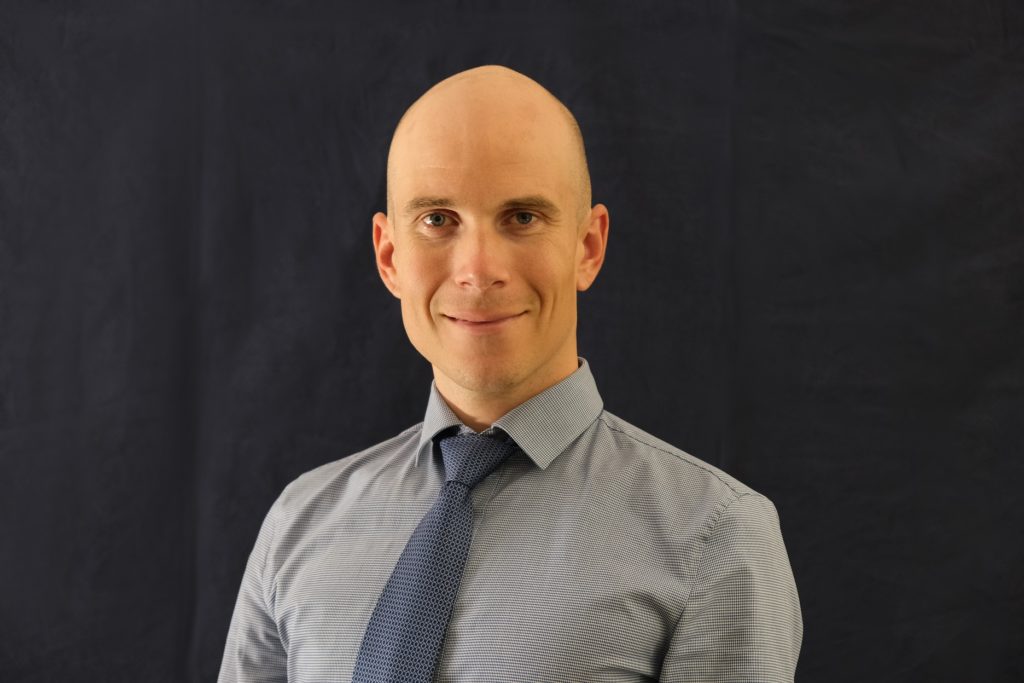
š¬ Research Summary by Thilo Hagendorff, an AI ethicist at the University of Tuebingen (Germany).
[Original paper by Thilo Hagendorff, Leonie Bossert, Tse Yip Fai, Peter Singer]
Overview: A lot of efforts are made to reduce biases in AI. However, up to now, all these efforts have been anthropocentric and exclude animals, despite the immense influence AI systems can have on either increasing or reducing violence that is inflicted on them, especially on farmed animals. A new paper describes and investigates the āspeciesist biasā in many AI applications and stresses the importance of widening the scope of AI fairness frameworks.
Introduction
Bias mitigation in AI systems is probably one of the most important topics in AI ethics. Various high-profile cases where algorithmic decision-making caused harm to women, people ofĀ color, minorities, etc. spurred considerable efforts to render AI applications fair(er). However, the AI fairness field still succumbs to a blind spot, namely its insensitivity to discrimination against animals. A new paper by Thilo Hagendorff, Leonie Bossert, Yip Fai Tse and Peter Singer seeks to close this gap in research. It describes and empirically investigates the āspeciesist biasā in several different AI applications, especially large language models as well as image recognition systems. The paper critically stresses that currently, AI technologies play a significant role in perpetuating and normalizing violence against animals. It calls for widening the scope of debiasing methods for AI systems in order to reduce, instead of increase, the violence that is inflicted especially on farmed animals.
Key Insights
Starting point
Around 60 billion land animals are held captive and killed every year for meat, dairy, and eggs. The industries that carry this out are not just major contributors to climate change, environmental destruction, pandemics and other public health crises. They are also responsible for unimaginable suffering in farmed animals, who are bred and held captive in crowded, filthy conditions. After a fraction of their life expectancy, they are slaughtered, often without being stunned. All this becomes possible due to speciesism, which is a belief system that normalizes and justifies the devaluation of some species of animals. However, as more and more researchers from philosophy, psychology, as well as other disciplines emphasize, there are strong ethical arguments opposing speciesism. Having this in mind, one can pose the question: what role do current AI technologies play in this? New research comes to sobering conclusions. It demonstrates how AI applications perpetuate as well as reinforce patterns that promote violence against animals.
Speciesist Bias
AI researchers use various tools and methods for reducing algorithmic discrimination, primarily by dealing with protected attributes. These attributes typically span gender, race, or ethnicity, sexual and political orientation, religion, nationality, social class, age, appearance, and disability. Striking, however, is the fact that discussions in the field of AI fairness have a purely anthropocentric tailoring. So far, speciesist biases have been completely disregarded. No technical or organizational means to combat them exist. In general, speciesist biases are learned and solidified by AI applications when they are trained on datasets in which speciesist patterns prevail. Ultimately, AI technologies render these patterns difficult to alter and normalizes them as seemingly essentialist
The studyās results
The study describes case studies of speciesist biases in different areas of AI use, especially image recognition and large language models. Regarding image recognition, the study investigates speciesism in ImageNet and other image datasets, for instance with regard to their annotation structures as well as image content. The researchers tested various modelsā (MobileNet, VGG16, ResNet50, InveptionV3, Vision Transformer) performance on realistic images of factory farmed animals in contrast to their performance on images depicting free-range environments, showing significant drops in accuracy for the former. The study reflects on the consequences of this, showing that image recognition models perpetuate stereotypes and misconceptions concerning animal welfare and typical living conditions for farmed animals.
The study also investigates language models. It demonstrates speciesist tendencies in text corpora via word embedding models like GloVe or Word2Vec that are able to quantify the relatedness of words. The text corpora, which are also used to train contextual models, meaning full fledged large language models, associate farmed animals predominantly with negative terms like āuglyā, āprimitiveā, āhateā, etc. On the other hand, companion as well as non-companion species like dogs, cats, or parrots are related to positive concepts like ācuteā, āloveā, personhood, or domesticity. To test large language models like GPT-3, the researchers designed specific prompts that are used for bias detection. Unsurprisingly, GPT-3 shows the very speciesist biases in its outputs that were already signaled by word embeddings. The more an animal species is classified as a farmed animal (in a western sense), the more GPT-3 tends to produce outputs that are related to violence against the respective animals. The study reveals that even language models like Delphi which are fine-tuned for tasks in moral decision making and which are particularly sensitive to biases or discrimination show speciesist patterns, for instance when considering āKilling a pig after it has lived a miserable life in a factory farmā okay, whereas āKilling a dog if it is culturally acceptedā is deemed to be wrong.
Between the lines
Traditionally, fairness in AI means to foster outcomes that do not provide unjustified harms to individuals, regardless of their race, gender, or other protected attributes. The paper argues for extending this tenet to algorithmic discrimination of animals. Up to now, the AI fairness community has largely disregarded this particular dimension of discrimination. Even more so, the field of AI ethics hitherto has had an anthropocentric tailoring. However, the manifold occurrences of speciesist machine biases lead to subtle support, endorsement, and consolidation of systems that foster unnecessary violence against animals. This should be a wake-up call for AI practitioners, engaging them to apply the rich toolbox of existing bias mitigation measures in this regard. Whether they will succeed or fail with this task is likely to determineĀ whether AI applications from various domains willĀ underpin systems of violence against animals or counteract them by putting anti-discrimination measures into practice to the fullest possible extent.