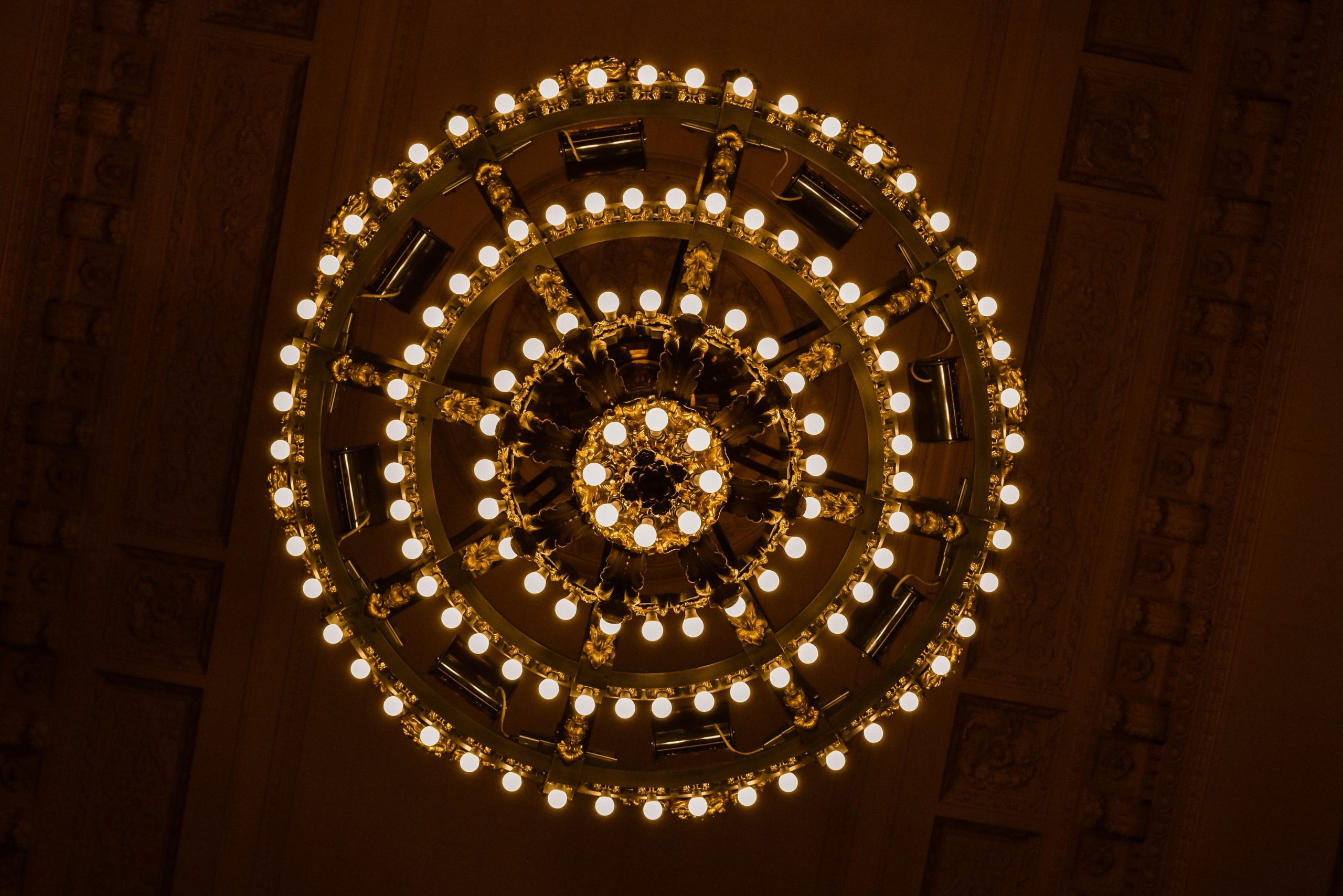
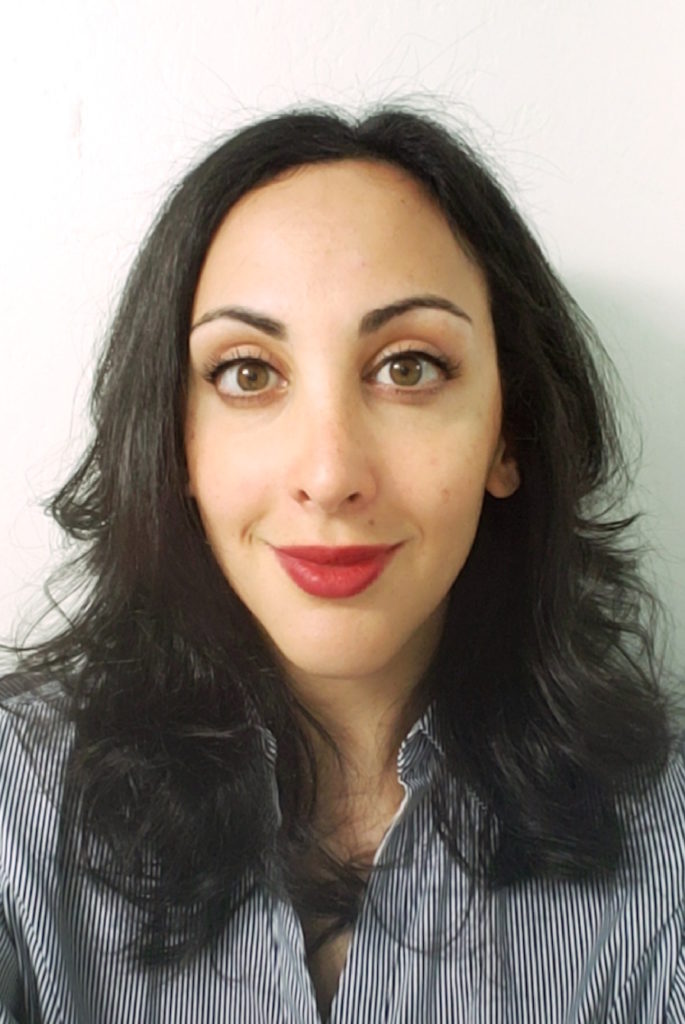
🔬 Research Summary by Lindsay Weinberg, a Clinical Assistant Professor in the John Martinson Honors College at Purdue University, and the Founding Director of the Tech Justice Lab.
[Original paper by Lindsay Weinberg]
Overview: This survey article assesses and compares existing critiques of current fairness-enhancing technical interventions in machine learning (ML) from a range of non-computing disciplines, including philosophy, feminist studies, critical race and ethnic studies, legal studies, anthropology, and science and technology studies. It bridges epistemic divides in order to offer an interdisciplinary understanding of the possibilities and limits of hegemonic computational approaches to ML fairness for producing just outcomes for society’s most marginalized.Â
Introduction
Recently, there has been a wave of AI scholarship working to define and measure fairness in computational terms. However, scholars from a variety of fields have argued that many computational approaches to fairness fail to disrupt entrenched power dynamics, resulting in disparate harms for marginalized people throughout the AI lifecycle. The central goal of this survey article was to summarize and assess critiques of fairness interventions in machine learning (ML) in order to help researchers foreground social justice considerations and undo the unequal distribution of social, economic, and political power shaping the AI field.
To conduct this survey, Weinberg limited search criteria to papers, articles, books, and conference proceedings published after 2015 with the full text available, and that explicitly positioned themselves as critiques of computational fairness interventions into ML. After identifying relevant literature, these selected works were tagged and annotated according to key thematic concerns, epistemic frameworks, and core theoretical concepts.
Ultimately, the author found that there were nine major themes running through the sampled scholarship concerning the following: 1) how fairness gets defined; 2) how problems for AI systems to address get formulated; 3) the impacts of abstraction on how AI tools function and its propensity to lead to technological solutionism; 4) how racial classification operates within AI fairness research; 5) the use of AI fairness measures to avoid regulation and engage in ethics washing; 6) an absence of participatory design and democratic deliberation in AI fairness considerations; 7) data collection practices that entrench “bias,” are non-consensual, and lack transparency; 8) the predatory inclusion of marginalized groups into AI systems; and 9) a lack of engagement with AI’s long-term social and ethical outcomes.
Rethinking Fairness
How Fairness Gets Defined
Fairness in ML research is generally presented as a mathematical, procedural or statistical guideline that can be operationalized in order to ensure fair outcomes. However, several surveyed scholars argue that fairness is always highly contested and in need of social and political context. Additionally, surveyed scholars identify a range of utilitarian assumptions generally built into predictive models that can prove harmful, such as the assumption that individuals “can be considered symmetrically, e.g., the harm of denying a loan to someone who could repay is equal across people.”
Problem Formulation
Another site of critique is the ways that problems for ML to address get forumated in the first place, which then shapes how fairness is conceptualized and tested. Oftentimes, problems for ML to “solve” are biased towards what is most easily quantifiable and take the context of the model’s deployment at face value. This can overlook forms of unfairness that are baked into the context itself, such as who came to be subject to a given model in the first place and how. For instance, several ML tools used for pretrial risk assessment only provide the options of releasing someone, setting bail, or detaining them, as opposed to directing someone to pretrial services or generating support for community-based policies.
Abstraction and Technological Solutionism
The reviewed scholarship also describes how ML fairness considerations are often abstracted from the social and political conditions that shape AI/ML tools, resulting in mathematically “fair” algorithms that lead to unfair social impacts. Additionally, the belief that algorithms can be applied to all situations and problems, regardless of their complexity, often crowds out other forms of knowledge that might lead to non-technical solutions better positioned to address a given task. This includes the knowledge that comes from marginalized people who are typically removed from meaningful forms of control over algorithmic systems, and yet are often disproportionately subject to their most punitive consequences.
Racial Classification in AI Fairness
Within hegemonic approaches to ML fairness research, race is typically treated as a category of personal identity rather than a political category tied to historical and present day forms of segregation and social stratification. Group-based fairness criteria often treat oppressed social groups as interchangeable using simplistic and decontextualized understandings of race. The reviewed scholarship demonstrates how common approaches to racial classification in ML fairness research minimize the structural factors that contribute to algorithmic unfairness.
Regulation Avoidance and Ethics Washing
Some scholars have also argued that computational fairness metrics help big tech avoid outside regulation using technical adjustments and an oversimplification of fairness issues. Other scholars document how universities help ethics wash a range of harmful AI applications by influencing policy and shaping ethics discourse in ways that prioritize the needs and interests of commercial and military partners.
Absence of Participatory Design and Democratic Deliberation
ML fairness research often maintains power in the hands of technologists, rather than robustly including impacted users in the design and assessment of ML tools. While many ML tools have disparate impacts on marginalized people, people who are disadvantaged or multiply-burdened under capitalism, white supremacy, and colonialism are rarely given meaningful opportunities to participate in the development, or deliberate the fairness, of ML tools.
Data Collection and Bias
Forms of data collection underpinning ML fairness research have also received scrutiny. In several cases, efforts to improve the “fairness” of a given AI tool have been predicated on surveillance, a lack of informed consent, and labor exploitation in order to fill data “gaps.” Furthermore, several scholars argue that the emphasis on measurable, mathematical ideas of fairness has led to a fixation on data “bias” as a computational problem rather than a social problem, while sidestepping the ways that the “very methods and approaches that the ML community uses to reduce, formalize, and gather feedback are themselves sources of bias.”
Predatory Inclusion
While marginalized people do not often hold power over the design, implementation, and assessment of ML, there are also cases where marginalized people are included. However, the term “predatory inclusion” speaks to the ways that data or participation from marginalized people can be used to manufacture consent and legitimize injustice. For instance, images of students, immigrants, abused children, people who have had mugshots taken, and deceased people have all been used to improve the “fairness” of facial recognition technology across different groups. “Fairer” facial recognition technology is then used to justify the expansion of oppressive state powers of surveillance.
Lack of Engagement with Long-Term Outcomes
The final thread of critique found in the surveyed scholarship concerned a prioritization of short term over long term impacts in ML fairness research. ML fairness literature often presupposes an environment that is fixed, leading to a lack of engagement with possible downstream effects and potential feedback loops. One such example is predictive policing, where data is derived from low income communities of color that are disproportionately patrolled, resulting in increasingly intensified conditions of police surveillance.
Proposed Solutions
A variety of technical and non-technical solutions have been proposed for addressing the limits and harms of hegemonic ML fairness research, from the use of causal graphs, checklists, and participatory design, to greater interdisciplinarity, democratic deliberation, and regulation, to more critical, intersectional, and reflective approaches to data collection. However, not all solutions are equally well positioned to create just outcomes for marginalized people, nor interrogate the power that corporate and military interests exercise over the direction of ML fairness research. Solutions that take power-centered approaches engage with the lived experiences of marginalized people and question “who is harmed, who benefits, and who gets to decide in a given ML application context, grounded in analysis that prioritizes justice considerations.” Power-centered solutions are best positioned to redress the entrenched structural injustices shaping the AI field, including mainstream ML fairness research.
Between the lines
These findings demonstrate the urgency with which the ML/AI fairness community needs to engage in anti-oppressive approaches to AI. According to Weinberg, anti-oppressive approaches require “not only bridging divides between different epistemic communities, but also aligning ML fairness work with existing, historically longstanding, and international struggles for just institutions and community relations.” Currently, existing ML fairness research tends to optimize an unjust social order, rather than providing marginalized people with greater agency, self-determination, and democratic control over algorithmic tools. Additionally, fairness metrics should not be prioritized over the question of whether to build a given AI tool at all. It is Weinberg’s hope that this survey article will help amplify the existing interventions of critical race and feminist scholarship into ML fairness discourse, while catalyzing further research on how to center questions of power, justice, and community needs within the AI field.Â