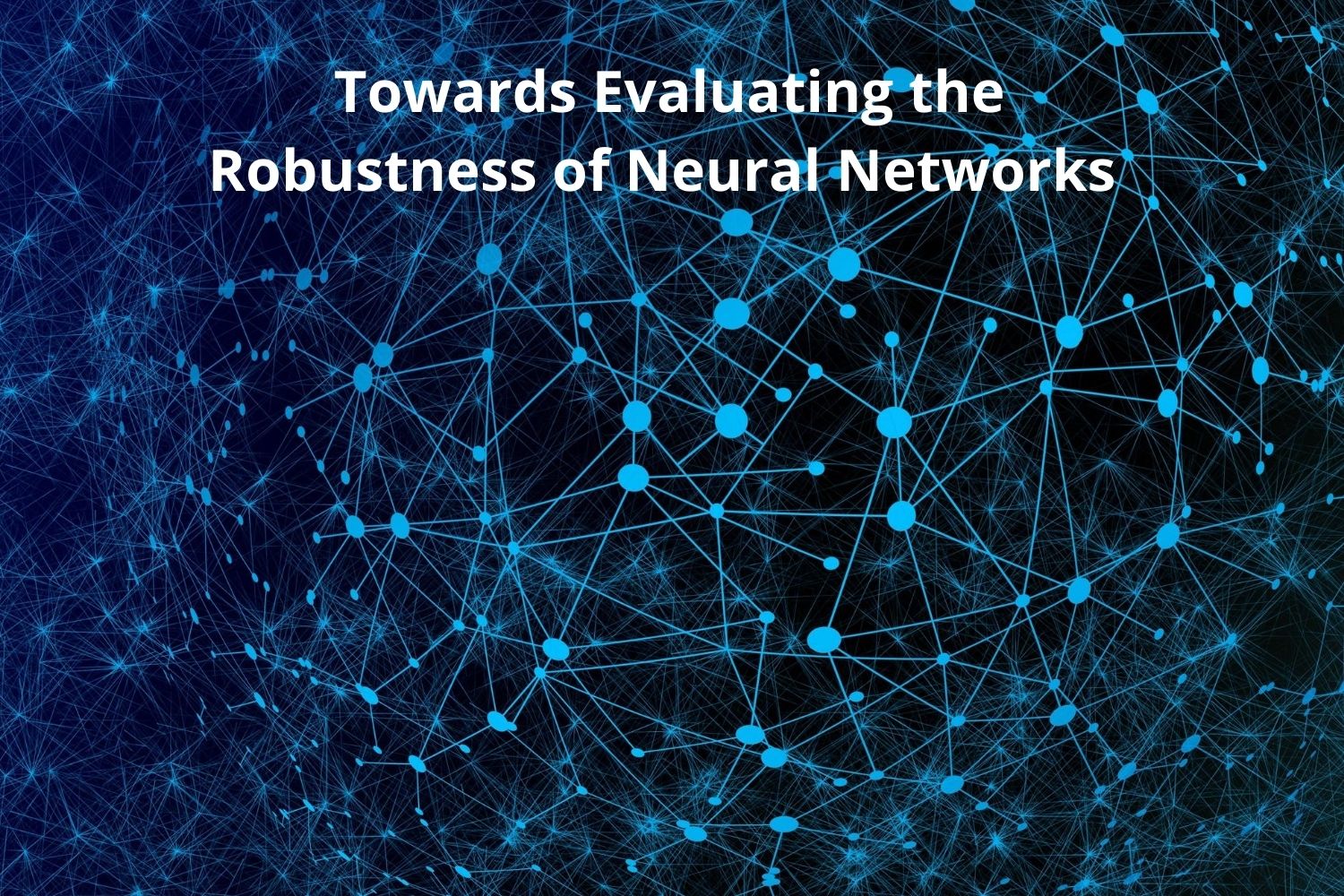
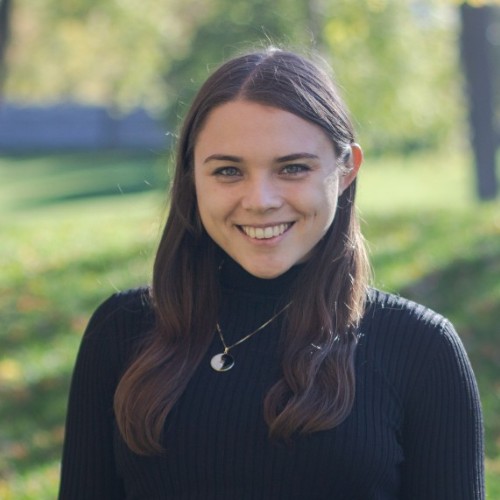
Summary contributed by Shannon Egan, Research Fellow at Building 21 and pursuing a master’s in physics at UBC.
*Author & link to original paper at the bottom.
Mini-summary:
Neural networks (NNs) have achieved state-of-the-art performance on a wide range of machine learning tasks. However their vulnerability to attacks, including adversarial examples (AEs), are a major barrier to their use in security-critical decisions.
AEs are manipulated inputs x’ which are extremely similar to an input x with correct classification C*(x), and yet are misclassified as C(x’) =/= C*(x). In this paper Carlini and Wagner highlight an important problem: there is no consensus on how to evaluate whether a network is robust enough for use in security-sensitive areas, such as malware detection and self-driving cars.
To address this, they develop 3 adversarial attacks which prove more powerful than existing methods. All 3 attacks generate an AE by minimizing the sum of two terms: 1) The L2, L0 or L∞ distance between the original input and the presumptive AE and 2) an objective function which penalizes any classification other than a chosen target class. The latter term is multiplied by a constant c, with larger c corresponding to a more “aggressive” attack and larger manipulation of the input. If c is too small, the resulting AE may fail to fool the network.
Using 3 popular image classification tasks, MNIST, CIFAR10, and ImageNet, the authors show that their attacks can generate an AE for any chosen target class. Furthermore, the adversarial images are often indistinguishable from the originals. The L2 and L∞ attacks are especially effective, only requiring a small c to achieve the desired classification.
Crucially, the new attacks are effective against NNs trained by defensive distillation, which was proposed as a general-purpose defense against AEs. While defensive distillation blocks AEs generated by L-BFGS, fast gradient sign, DeepFool and JSMA, the new attacks still achieve a 100% success rate at finding an AEs, with minimal increase in the aggressiveness of the attack.
These results suggest that stronger defenses are needed to ensure robustness against AEs, and NNs should be vetted against stronger attacks before being deployed in security-critical areas. The powerful attacks proposed by Carlini and Wagner are a step towards better robustness testing, but NN vulnerability to AEs remains an open problem.
Full summary:
Neural networks (NNs) have achieved state-of-the-art performance on a wide range of machine learning tasks, and are being widely deployed as a result. However their vulnerability to attacks, including adversarial examples (AEs), is a major barrier to their application in security-critical decisions.
AEs are manipulated images x’ which remain extremely close, as measured by a chosen distance metric, to an input x with correct classification C*(x), and yet are misclassified as C(x’) =/= C*(x). One can even choose an arbitrary target class t, and optimize the AE such that C(x’) = t. The stereotypical AE in image classification is so close to its base image that a human would not be able to distinguish the original from the adversarial by eye.
Despite the fact that AEs exist, and moreover have proven easy to generate, there is little consensus on how to test NNs for robustness against adversarial attacks, and even less on what constitutes an effective defense. One promising defense mechanism, known as defensive distillation, has been shown to reduce the success rate of existing AE generation algorithms from 95% to 0.5%. In this paper, Carlini and Wagner devise 3 new attacks which show no significant performance decrease when attacking a defensively “distilled” NN. Defensive distillation’s inefficacy against these more powerful attacks underlines the need for better defenses against AEs.
The authors’ new attacks generate an AE by minimizing the sum of two terms: 1) The L2, L0, or L∞ distance between the original input and the presumptive adversarial and 2) an objective function that penalizes any classification other than the target. The latter term is multiplied by a constant c, which is used as a proxy for the aggressiveness of the attack. A larger c indicates that a larger manipulation is required to produce the target classification.
Using 3 popular image classification tasks, MNIST, CIFAR10, and ImageNet, the authors show that their attacks can generate an AE for any chosen target class, with a 100% success rate. Furthermore, the adversarial images are often visually indistinguishable from the originals. The L2 and L∞ attacks are especially effective, only requiring a small c to achieve the desired classification (and therefore a small manipulation of the input). When compared to existing algorithms for generating AEs, including Szegedy et al.’s L-BFGS, Goodfellow et al.’s fast gradient sign method (FGS), Papernot et al.’s Jacobian-based Saliency Map Attack (JSMA), and Deep-fool, Carlini and Wagner’s AEs fool the NNs more often, with less severe modification of the initial input.
Crucially, the new attacks are effective against NNs trained by defensive distillation, an alternative supervised learning approach which was invented to prevent overfitting. This is achieved by training the network twice: the first time using the standard approach of inputting only the correct label to the cost function; and the second time using the “soft labels” which indicate the probability of each class, returned by the network itself after the initial training. While defensive distillation blocks AEs generated by L-BFGS, fast gradient sign, DeepFool and JSMA, the new attacks still achieve a 100% success rate at finding an AE, with minimal increase in the aggressiveness of the attack (i.e. c does not have to increase significantly to produce an AE with the desired target classification).
The stronger attacks proposed by Carlini and Wagner are important for demonstrating the vulnerabilities of defensive distillation, and for establishing a potential baseline for NN robustness testing. However the problem of NN susceptibility to AEs will not be solved by these attacks. In future, a defense which is effective against these methods may be proposed, only to be defeated by an even more powerful (or simply different) attack. An effective defense will likely need to be adaptive, capable of learning as it gathers information from attempted attacks.
We should also look to general properties of AE behaviour for guidance. One key to better defenses may be the transferability principle, a phenomenon whereby AEs generated for a certain choice of architecture, loss function, training set etc. are often effective against a completely different network; even eliciting the same faulty classification. A strong defense against AEs will have to somehow break transferability, otherwise an attacker could generate AEs on a network with weaker defenses, and simply transfer them to the more robust network.
The attacks proposed by Carlini and Wagner are a step towards better robustness testing, but NN vulnerability to AEs remains an important open problem.
Original paper by Nicholas Carlini and David Wagner: https://arxiv.org/abs/1608.04644