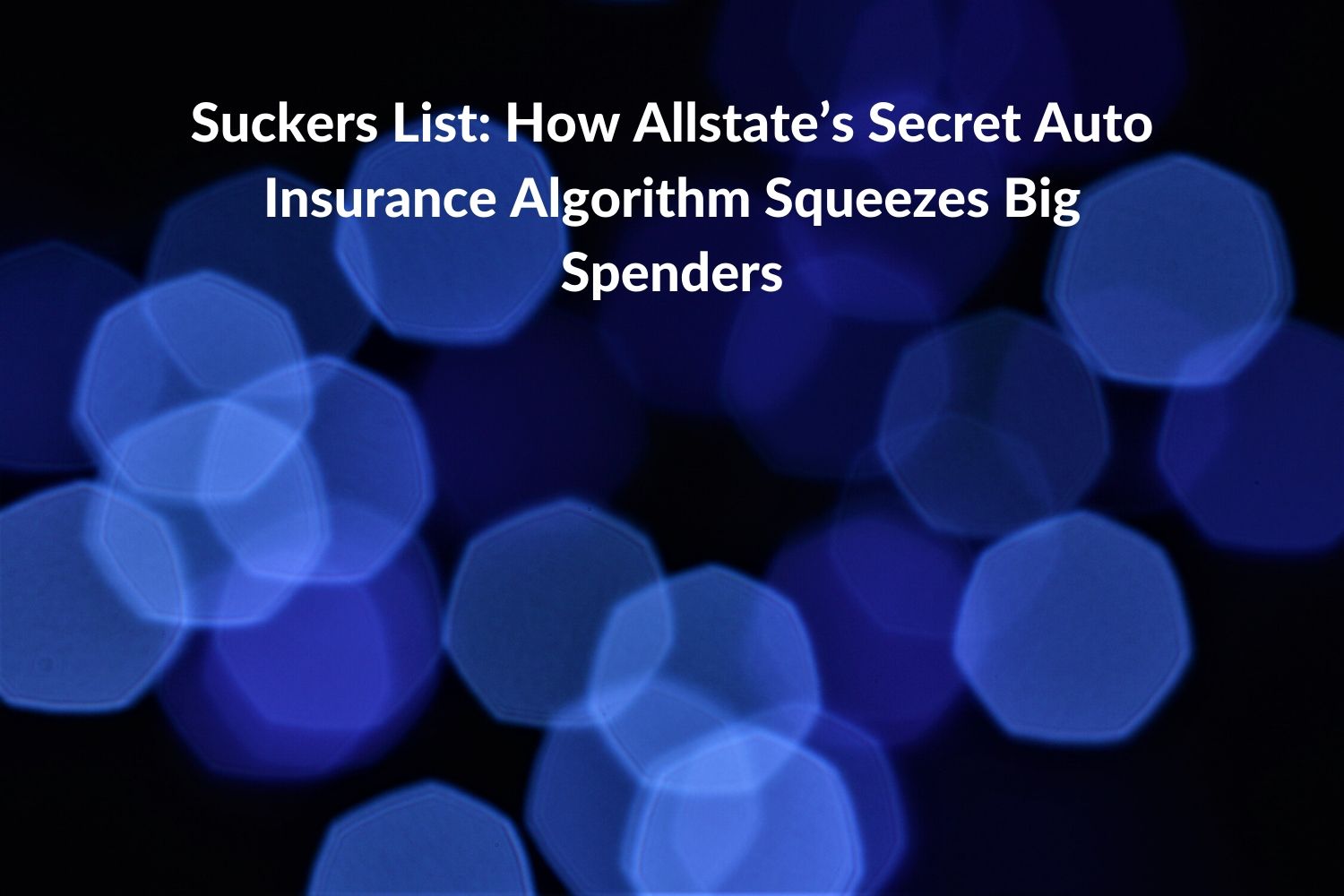
Top-level summary: Ever wondered what goes into pricing your insurance premiums the way they are and why they are different from others who you know, even when you share a largely similar risk profile? This investigative piece from The Markup by Maddy Varner and Aaron Sarkin delved deep into the discriminatory pricing practice from the US auto insurer Allstate that utilized purportedly complex models to offer fairer prices to their consumers when in reality their model’s complexity boiled down to 2 key things: how price-inelastic the consumer was and how unwilling they are to switch insurance providers. While price discriminaton has been done in a variety of industries and has often been shrugged off as the state of affairs, this case has been particularly impactful because it is doing so in the domain of something that is an essential service in being able to own and operate a vehicle.
Additionally, the impacts were disproportionately on those who had less of an ability to adjust to the changing circumstances, often where the difference of a few hundred dollars meant fewer meals on the table. Opacity is often practiced under the legal cover of wanting to protect privacy and trade secrets, this move by Allstate gave a rare insight into their operating models in part because of the demands from regulators to justify and support their claims for revising pricing policies. The documentation that was provided by the company showed how increases weren’t necessarily limited, in places where they had been overcharging, the discounts offered were only minimal creating a distortion where they limited their downside by offering pennies on the dollar while maximizing their upside. Resigning to this unfair practice, consumers are forced to bear the burden of having to constantly check prices from competitors to ensure that they aren’t being price-gouged. Regulators, at the moment, stand toothless because they lack the data and aren’t asking the right questions that would unearth some of these unfair practices.
In Maryland, Allstate, an auto insurer, filed with the regulators that the premium rates needed to be updated because they were charging prices that were severely outdated. They suggested that not all insurance premiums be updated at once but instead follow recommendations based on an advanced algorithmic system that would be able to provide deeper insights into the pricing that would be more appropriate for each customer based on the risk that they would file a claim. This was supposed to be based on a constellation of data points collected by the company from a variety of sources.
Because of the demand from the regulators for documentation supporting their claim, they submitted thousands of pages of documentation that showed how each customer would be affected, a rare window into the pricing model which would otherwise have been veiled under privacy and trade secret arguments. A defense that is used by many companies that utilize discriminatory pricing strategies using data sourced beyond what they should be using to make pricing decisions.
According to the investigating journalists, the model boiled down to something quite simple: the more money you had and the higher your willingness to not budge from the company, the more the company would try to squeeze from you in terms of premiums.
Driven by customer retention and profit motives, the company pushed increases on those that they knew could afford them and would switch to save dollars. But, for those policies that had been overpriced, they offered less than 0.5% in terms of a discount limiting their downsides while increases were not limited, often going up as high as 20%.
While they were unsuccessful in getting this adopted in Maryland where it was deemed discriminatory, the model has been approved for use in several states thus showing that opaque models can be deployed not just in high-tech industries but anywhere to provide individually tailored pricing to extract away as much of the consumer surplus as possible based on the purportedly undisclosed willingness of the customer to pay (as would be expressed by their individual demand curves which aren’t directly discernible to the producer).
Furthermore, the insurers aren’t mandated to make disclosures of how they are pricing their policies and thus, in places where they should have offered discounts, they’ve only offered pennies on the dollar, disproportionately impacting the poorest for whom a few hundred dollars a year can mean having sufficient meals on the table.
Sadly, in the places where their customer retention model was accepted, the regulators declined to answer why they chose to accept it, except in Arkansas where they said such pricing schemes aren’t discriminatory unless the customers are grouped by attributes like race, color, creed or national origin. This takes a very limited view of what price discrimination is, harkening back to an era where access to big data about the consumer was few and far between. In an era dominated by data brokers that compile thick and rich data profiles on consumers, price discriminaton extends far beyond the basic protected attributes and can be tailored down to specificities of each individual.
Other companies in retail goods and online learning services have been following this practice of personalized pricing for many years, often defending it as the cost of doing in business when they based the pricing on things like zip codes, which are known proxies for race and paying capacity. Personalized pricing is different from dynamic pricing, as seen when booking plane tickets, which is usually based on the timing of purchase whereas here the prices are based on attributes that are specific to the customer which they often don’t have any control over.
A 2015 Obama Administration report mentioned that, “Differential pricing in insurance markets can raise serious fairness concerns, particularly when major risk factors are outside an individual customer’s control.” Why the case of auto insurance is so much more predatory than, say buying stationery supplies, is that it is mandatory in almost all states and not having the vehicle insured can lead to fines, loss of licenses and even incarceration. Transport is an essential commodity for people to get themselves to work, children to school and a whole host of other daily activities.
In Maryland, the regulators had denied the proposal by Allstate to utilize their model but in official public records, the claim is marked as “withdrawn” rather than “denied” which the regulators claim makes no internal difference but Allstate used this difference to get their proposal past the regulators in several other states. They had only withdrawn their proposal after being denied by the regulators in Maryland.
The National Association of Insurance Commissioners mentioned that most regulators don’t have the right data to be able to meaningfully evaluate rate revision proposals put forth by insurers and this leads to approvals without review in a lot of cases. Even the data journalists had to spend a lot of time and effort to discern what the underlying models were and how they worked, essentially summing up that the insurers don’t necessarily lie but don’t give you all the information unless you know to ask the right set of questions.
Allstate has defended its price optimization strategy, called Complementary Group Rating (CGR) as being more objective, and based on mathematical rigor, compared to the ad-hoc, judgemental pricing practices that have been followed before, ultimately citing better outcomes for their customers. But, this is a common form of what is called “mathwashing” in the AI ethics domain where discriminatory solutions are pushed as fair under the veneer of mathematical objectivity.
Regulators in Florida said that setting prices based on the “modeled reaction to rate changes” was “unfairly discriminatory.” Instead of being cost-based, as is advocated by regulators for auto-insurance premiums because they support an essential function, Allstate was utilizing a model that was heavily based on the likelihood of the customer sticking with them even in the face of price rises which makes it discriminatory. These customers are price-inelastic and hence don’t change their demand much even in the face of dramatic price changes.
Consumer behaviour when purchasing insurance policies for the most part remains static once they’ve made a choice, often never changing insurers over the course of their lifetime which leads them to not find the optimal price for themselves. This is mostly a function of the fact that the decisions are loaded with having to judge complex terms and conditions across a variety of providers and the customers are unwilling to have to go through the exercise again and again at short intervals.
Given the opacity of the pricing models today, it is almost impossible to find out what the appropriate pricing should be for a particular customer and hence the most effective defense is to constantly check for prices from the competitors. But, this unduly places the burden on the shoulders of the consumer.
Original piece by Maddy Varner and Aaron Sarkin: https://themarkup.org/allstates-algorithm/2020/02/25/car-insurance-suckers-list