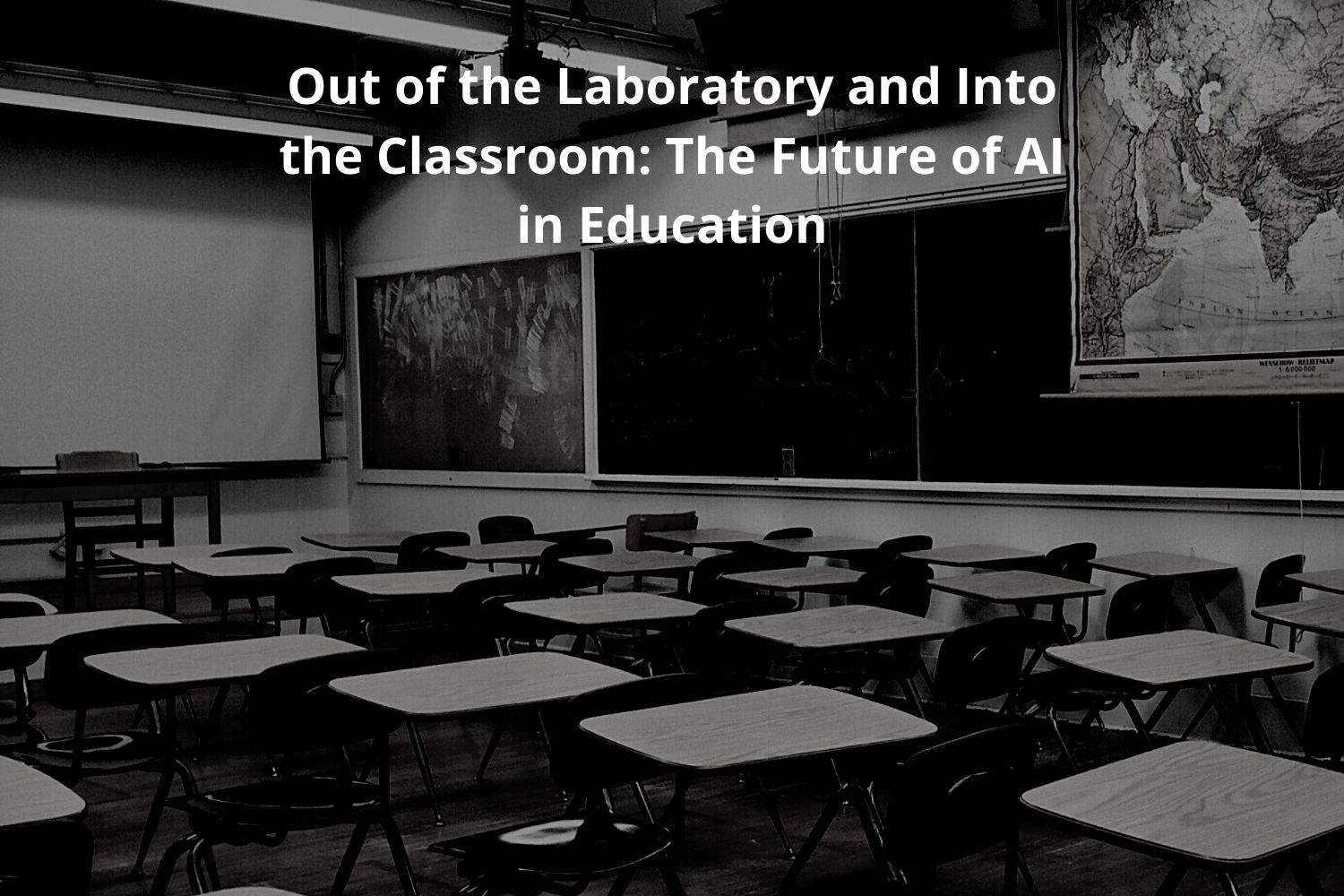
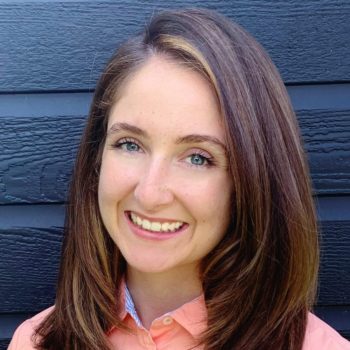
Summary contributed by Camylle Lanteigne (@CamLante), who’s currently pursuing a Master’s in Public Policy at Concordia University and whose work on social robots and empathy has been featured on Vox.
Link to full paper + authors listed at the bottom.
Mini-summary: Schiff begins by giving a broad overview of the often fraught relationship between education and new technological advances. From the arrival of writing in Platoâs time to social media only a few years ago, novel technologies have generated concerns about their effect on learning and students alike. Clearly, AIEd is no different.
Schiff also makes the crucial distinction between distance education and AIEd. Distance education (or distance learning) follows the structure of the classroom, employs textbooks and lectures in video format, and doesnât usually involve AI. One prominent form of distance education is Massive Open Online Courses (MOOCs). These courses are meant to be widely accessible, and are typically pre-recorded. The content, level of difficulty, and delivery of the course materials are not personalized for each student. While some believed MOOCs would allow greater accessibility for learners around the world who had little or no access to education, it later became clear that MOOCs instead overwhelmingly reached learners who already had adequate access to education.
On the other hand, one of AIEdâs key features is being able to personalize education in accord with each learnerâs preferences, abilities, and situation. AIEd also is not bound by the typical course structure (classroom, lectures, textbooks, et cetera). The main goal of AIEd, according to the author, is to âsimulate teachersâ and those who might be doing work similar to a teacherâs: mentors, tutors, and perhaps even educational administrators. Ultimately, AIEd doesnât only aim to convey course material, but also to do this in a way that is characteristic of how a teacher or other person in a teaching position would customize the material for learners.
Full summary:
The topic of AI in education has received considerably less attention so far, especially when compared to AI for predictive policing, for financial markets, or for social media, for example. Of course, this is no indicator that AI in education (or AIEd) doesnât hold important and pressing risks, along with exciting possibilities for education. Daniel Schiffâs paper âOut of the laboratory and into the classroom: the future of artificial intelligence in educationâ shines light on the current promises and future potential of AIEdâand, more specifically, intelligent tutoring systems as well as anthropomorphized artificial educational agentsâas it relates to the current structure of education systems, to labour displacement, and to delivering quality education to all students.
Schiff begins by giving a broad overview of the often fraught relationship between education and new technological advances. From the arrival of writing in Platoâs time to social media only a few years ago, novel technologies have generated concerns about their effect on learning and students alike. Clearly, AIEd is no different.
Schiff also makes the crucial distinction between distance education and AIEd. Distance education (or distance learning) follows the structure of the classroom, employs textbooks and lectures in video format, and doesnât usually involve AI. One prominent form of distance education is Massive Open Online Courses (MOOCs). These courses are meant to be widely accessible, and are typically pre-recorded. The content, level of difficulty, and delivery of the course materials are not personalized for each student. While some believed MOOCs would allow greater accessibility for learners around the world who had little or no access to education, it later became clear that MOOCs instead overwhelmingly reached learners who already had adequate access to education.
On the other hand, one of AIEdâs key features is being able to personalize education in accord with each learnerâs preferences, abilities, and situation. AIEd also is not bound by the typical course structure (classroom, lectures, textbooks, et cetera). The main goal of AIEd, according to the author, is to âsimulate teachersâ and those who might be doing work similar to a teacherâs: mentors, tutors, and perhaps even educational administrators. Ultimately, AIEd doesnât only aim to convey course material, but also to do this in a way that is characteristic of how a teacher or other person in a teaching position would customize the material for learners.
While AIEd may seem promising, its widespread adoption is not inevitable. Schiff gives three main reasons for this. First, barriers like cost, teachersâ ability to operate the AIEd system, and the preexisting school and system-level structures are only a few of the realities that might slow down or completely halt the implementation of AIEd technologies. Second, technology in education is not applied or used in a linear way. It is often, for a variety of reasons, adapted and used in ways that differ from technologistsâ plans. Thus, AIEd may find itself being diverted and changed in ways that may disrupt a complete and inevitable implementation. Third, it is still unclear that AIEd will be able to deliver the âquality educationâ distance education has already sought to offer. For one, student/(human) teacher interaction, the dialogue between the two parties, and the âactive involvement of the teacher,â may very well be necessary elements to quality education (regardless of content, personalization, or support from an AIEd system). Due to this, it at least seems unlikely that AIEd taking over our schools is inevitable.
Schiff evaluates some possible futures for AIEd using the factory and city models. The factory model represents a future where AIEd is used to promote efficiency, whereas the city model represents AIEd primarily promoting freedom in terms of learning and education. The author acknowledges that he emphasizes the factory model, which results in a skeptical and somewhat dystopian outlook on the future of AIEd. Through the lenses of the factory and city models, Schiff explores a few specific characteristics that may affect AIEd and its effects on society.
Algorithmic bias is a significant potential issue for AIEd, just as it is for AI systems more generally. The data that will be used to train AIEd systems will probably come from schools that can afford to implement AIEd early on, which are most likely located in wealthy, high-income countries. This will mean AIEd systems wonât be adapted to the needs of students in low-income countries, even though these âare the places that could be most positively impacted by AIEd.â The author also addresses the potential of AIEd to turn students away from non-STEM courses or advanced courses because they arenât available on the platform. The significant impact of AIEd systems on teachersâ roles, and the displacement teachers may suffer is likewise touched upon.
Schiff introduces issues related to international development and premature automation due to AIEd in low-income countries, as well as problems related to the flexibility and ownership of educational systems. In the first case, premature automation may mean that low-income countries and communities lock themselves âinto a kind of permanent second-tier educational systemâ if they havenât invested in educational infrastructure and high-quality teachers prior to investing in AIEd. In the second case, a lack of flexibility in AIEd means that curricula will most likely be focused on certain types of content, and built in a way that is partial to âcertain content, cultures, and learning styles.â AIEd also carries the risk for a mass standardization of education, which could affect creativity and educational freedom overall. The last element Schiff analyzes is nudging and manipulation. AIEd systems could for example employ techniques to shame students if they make a mistake, or âgamifyâ lessons. Such features can have significant effects on learners, both positive and negative.
In light of the above-mentioned risks, Schiff ends his paper by exploring ways in which AIEd research may be improved, so AIEd systems themselves become better. He mentions Responsible Research and Innovation (RRI), where researchers engage critically with the potential societal impacts of their research and the public is part of the dialogue regarding research aims. The peer review process can also be a tool to push researchers to consider the broader impacts of their research and highlight âtechnical or policy options that would mitigate those harms.â Lastly, it is imperative that AIEd research be inclusive of all the stakeholders that are involved in education. This includes teachers, students, parents, and administrative staff to name just a few.
Schiff hopes that continued efforts and dialogue around technologies like AIEd are essential for these to benefit all of us while avoiding the worst harms.
Original paper by Daniel Schiff: https://link.springer.com/article/10.1007/s00146-020-01033-8