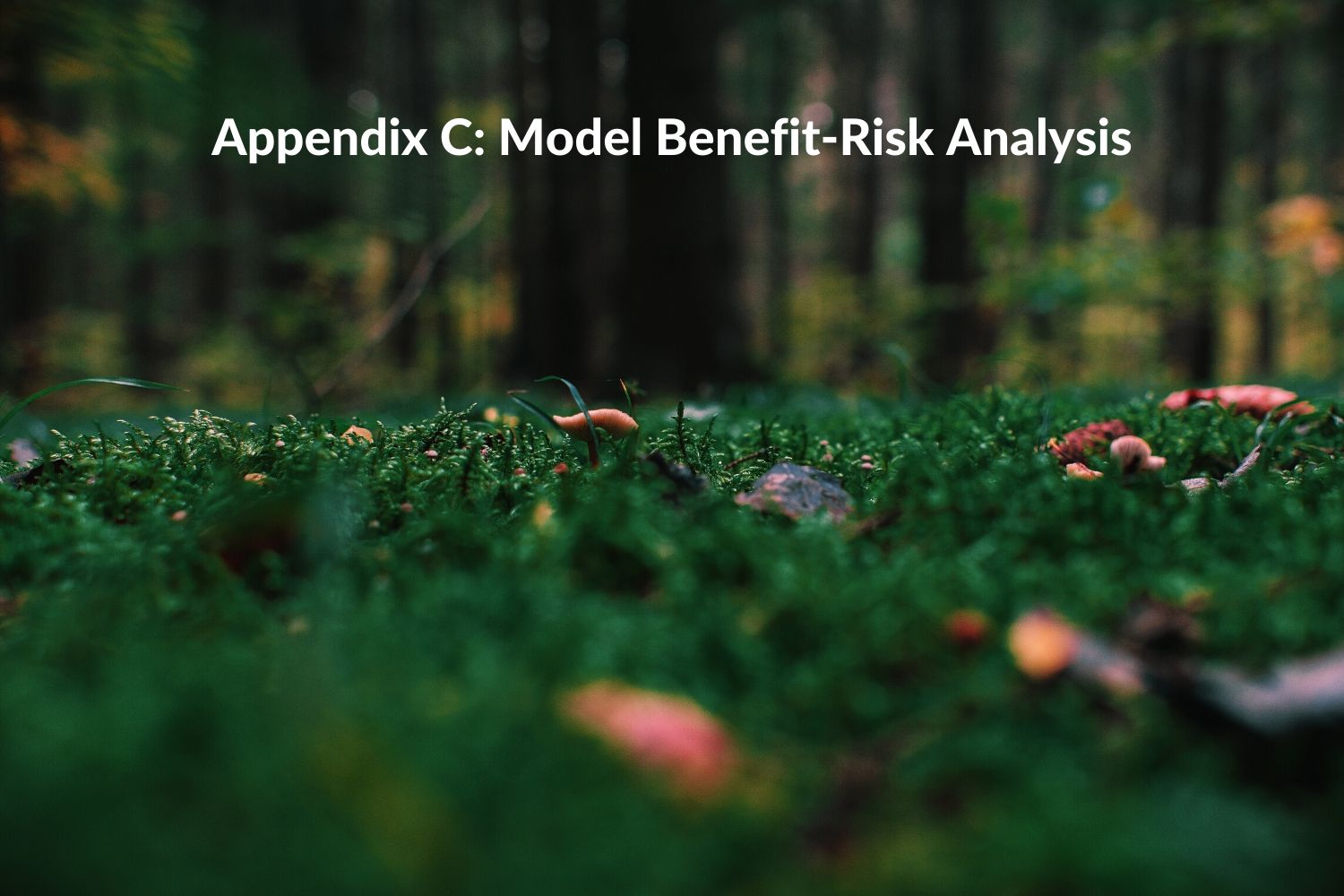
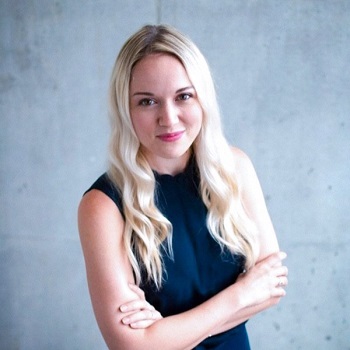
Summary contributed by Victoria Heath (@victoria_heath7), Communications Manager at Creative Commons.
*Author & link to original paper at the bottom
Data transparency is a key goal of the open data movement, and as different federal and municipal governments create open data policies, it’s important that they take into account the risks to individual privacy that come with sharing data publicly. In order to ensure open data privacy, open data managers and departmental data owners within governments need a standardized methodology to assess the privacy risks and benefits of a dataset. This methodology is a valuable component of building what the Future of Privacy Forum (FPF) calls a “mature open data program.”
In their City of Seattle Open Data Risk Assessment report, the FPF presents a Model Benefit-Risk Analysis that can be utilized to evaluate datasets and determine whether or not they should be published openly. This analysis is based on work by the National Institute of Standards and Technology, the University of Washington, the Berkman Klein Center, and the City of San Francisco. There are five steps to the analysis:
- Evaluate the information the dataset contains
This step involves identifying whether there are direct or indirect identifiers, sensitive attributes, non-identifiable information, spatial data, and other information in the dataset; assessing whether the dataset is linkable to other datasets, and; analyzing the “context in which the data was obtained.”
- Evaluate the benefits associated with releasing the dataset
This step includes considering the potential benefits and users of the dataset, including identifying whether the data fields involve aggregate data or individual records. To evaluate the potential benefits of the dataset, the evaluator selects a qualitative and quantitative value of the benefits and then selects a value for the likelihood of those benefits occurring. Those ratings are then compared to identify the overall benefits of releasing the dataset.
- Evaluate the risks associated with releasing the dataset
This step includes considering the potential privacy risks and negative users of the dataset. The foreseeable privacy risks include the re-identification (and false re-identification) impacts on individuals and/or organizations; data quality and equity impacts; and public trust impacts. To evaluate the potential privacy risks of the dataset, the evaluator selects a qualitative and quantitative value of the risks and then selects a value for the likelihood of those risks occurring. Those ratings are then compared to identify the overall privacy risk of releasing the dataset.
- Weigh the benefits against the risks of releasing the dataset
This step includes combining the scores from Steps 2 and 3 in order to determine whether to a) release the dataset openly, b) release it in a limited environment, c) create formal application and oversight mechanisms before publishing the dataset, or d) keep the dataset closed unless the risk can be reduced or there are other public policy reasons to consider. In this step, it is important to consider the level of acceptable privacy risks, while also considering the overall benefits of publishing the dataset openly; and, if necessary, what technical, administrative, and legal controls can be put in place to mitigate the identified risks. Technical controls include suppression, generalization/blurring, pseudonymization, aggregation, visualizations, perturbation, k-Anonymity, differential privacy, and synthetic data. Administrative and legal controls include contractual provisions, access fees, data enclaves, tiered access controls, and ethical and/or disclosure review board.
- Evaluate countervailing factors
This step includes considering any factors that may “justify releasing a dataset openly regardless of its privacy risk.” For example, if it’s in the public’s interest to release the dataset (e.g. salaries of elected officials), then it’s important to consider analyzing the dataset “holistically” and proceeding cautiously—because once a dataset is published openly it’s impossible to make it closed once again. It’s also important to document the analysis, considerations, and thinking behind publishing a dataset openly, especially if it was initially determined to remain closed. This is key to building and maintaining trust in the open data program.
Original paper by the Future of Privacy Forum: fpf.org/wp-content/uploads/2018/01/FPF-Open-Data-Risk-Assessment-for-City-of-Seattle.pdf